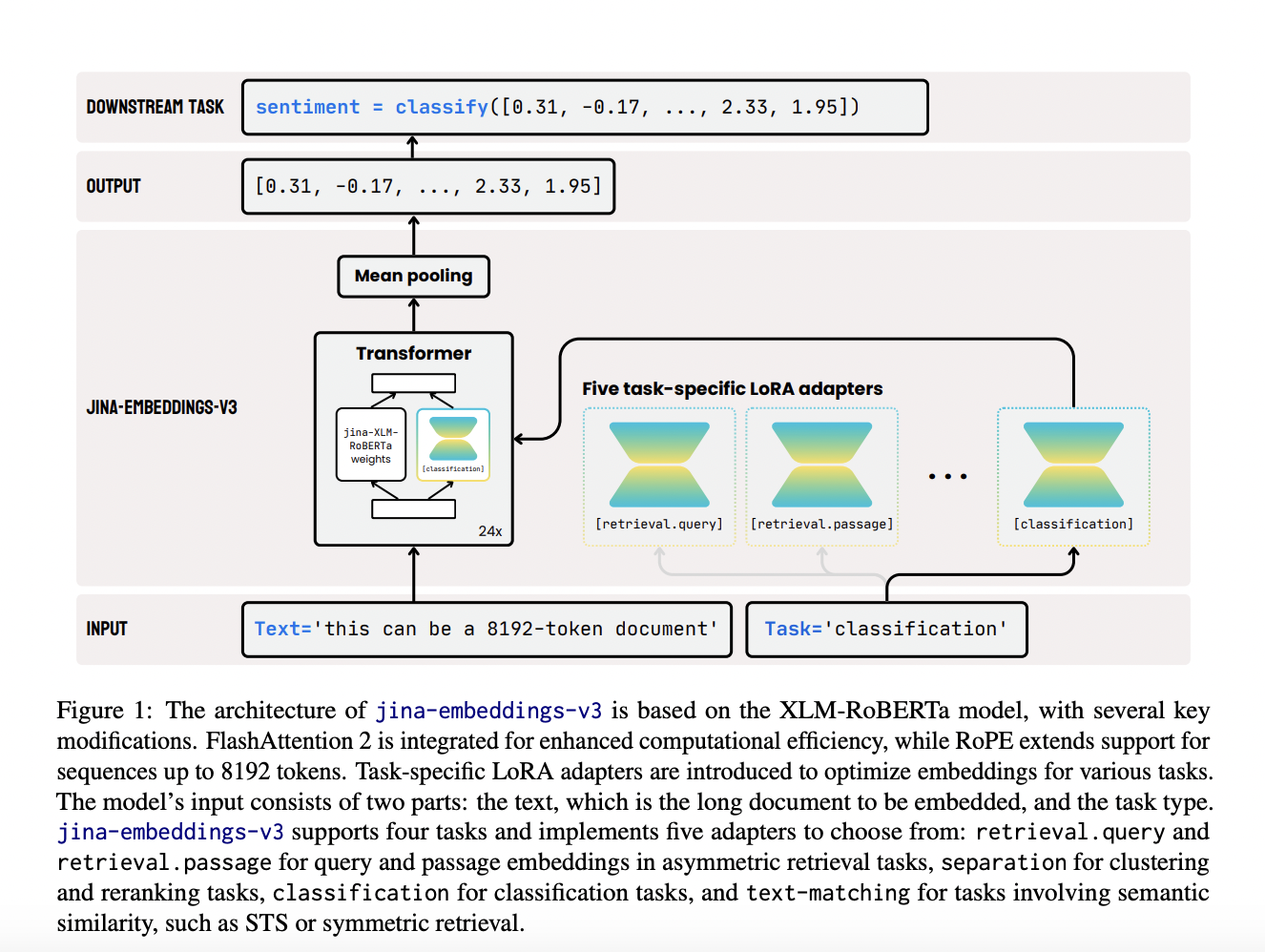
**Practical Solutions and Value of Jina-Embeddings-v3**
**Revolutionizing Text Embedding Efficiency**
Transform text into high-dimensional vectors for tasks like document retrieval, classification, and clustering.
Supports handling of multiple languages and long text sequences, enhancing performance in various NLP applications.
Solves inefficiencies of previous models by offering optimized performance across tasks and supporting longer-text contexts.
Improves computational efficiency with FlashAttention 2 and RoPE positional embeddings, enabling processing of tasks with up to 8192 tokens.
Utilizes Matryoshka Representation Learning to truncate embeddings without compromising performance, balancing space efficiency and task effectiveness.
Outperforms competitors in benchmark tests, demonstrating superior results in classification accuracy and multilingual tasks.
Efficient and cost-effective, making it ideal for deployment in real-world settings, especially for fast, on-edge computing applications.
Integrates LoRA adapters and advanced techniques to handle various functions without excessive computational burden.
Sets a new standard for embedding efficiency, providing a clear path forward for advancements in multilingual and long-text retrieval.
Valuable tool in NLP applications, offering scalability and efficiency in multilingual and long-context tasks.