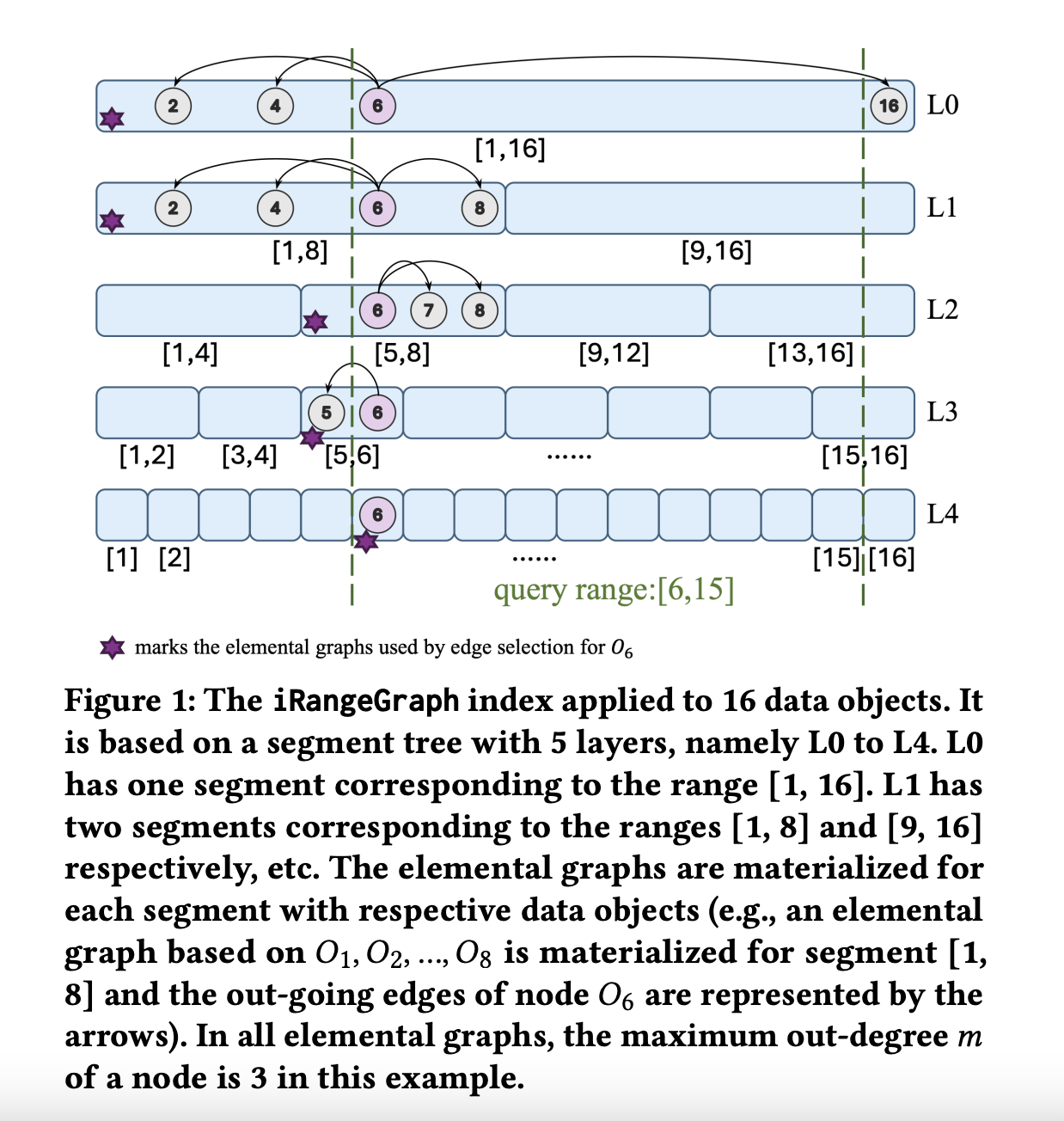
Practical Solutions for Efficient Nearest Neighbor Search with iRangeGraph
Enhancing Data Retrieval and Machine Learning
Graph-based methods play a crucial role in data retrieval and machine learning, especially in nearest neighbor (NN) search. This method helps identify data points closest to a given query, which is essential for high-dimensional data such as text, images, or audio. Approximate nearest neighbor (ANN) methods, like iRangeGraph, balance response time and accuracy, making them widely used in real-world applications such as recommendation engines, e-commerce platforms, and AI-based search systems.
Challenges and Solutions in NN Search
One major challenge in NN search arises when combining vector-based search with numeric attribute constraints. Traditional methods face performance issues, but iRangeGraph dynamically constructs graph indexes during query processing, conserving memory and ensuring efficient query response time. It can handle multi-attribute RFANN queries effectively, offering valuable solutions for large datasets across various industries.
Performance and Testing of iRangeGraph
iRangeGraph outperformed existing methods significantly in performance testing on real-world datasets, achieving 2x to 5x better query-per-second (qps) performance at 0.9 recall. It also demonstrated a memory footprint consistently smaller than competitors, making it suitable for large-scale systems where storage is critical. For multi-attribute RFANN queries, iRangeGraph showed a performance improvement of 2x to 4x in qps compared to other methods.
Revolutionizing Nearest Neighbor Search
iRangeGraph presents a novel and efficient solution for range-filtering approximate nearest neighbor queries, addressing the shortcomings of existing techniques. Its ability to deliver high performance across various query workloads while significantly reducing memory consumption makes it an ideal choice for large-scale data systems. The method’s flexibility in handling multi-attribute queries extends its applicability in real-world scenarios.