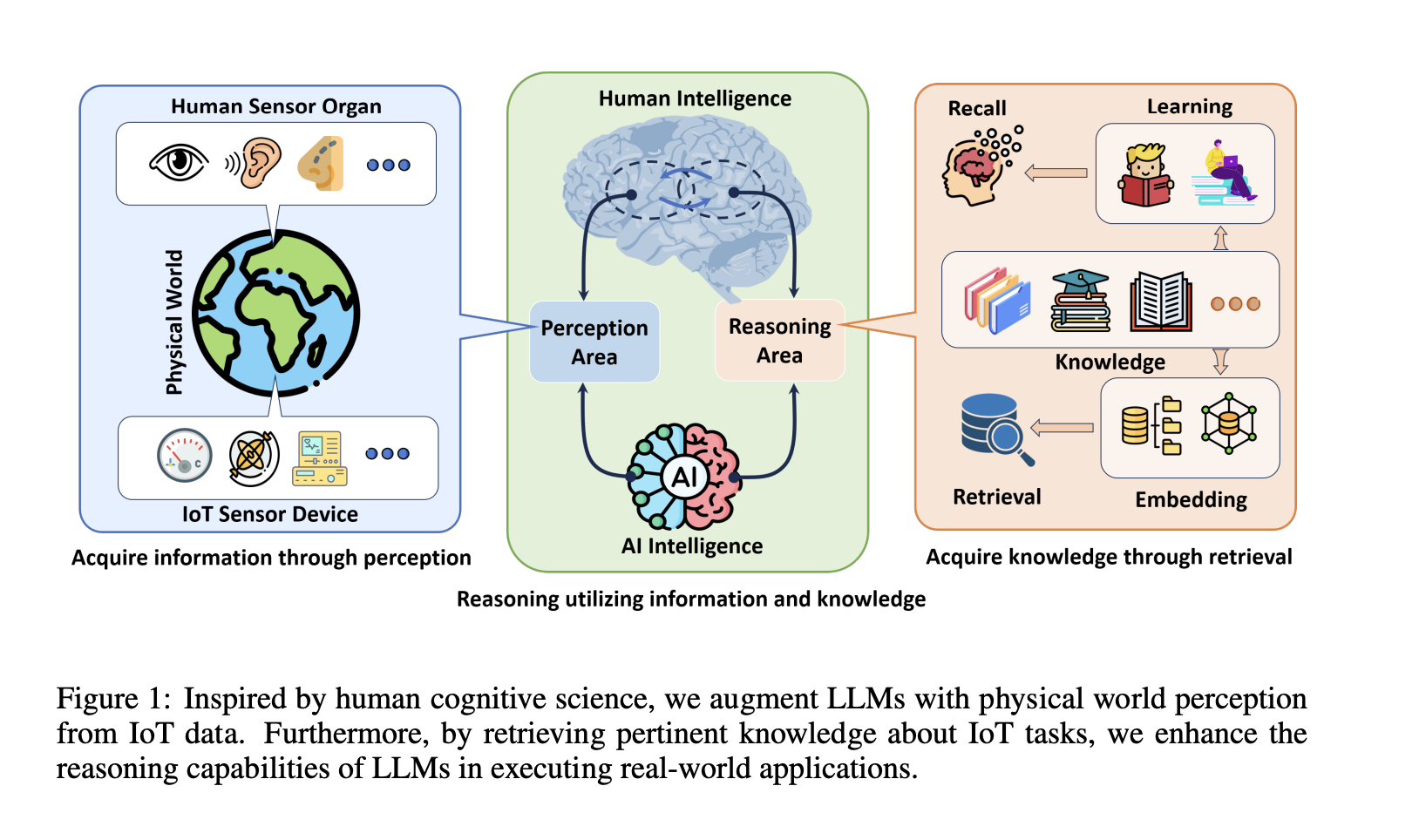
Enhancing IoT with AI: The IoT-LLM Framework
Growing sectors like Healthcare, Logistics, and Smart Cities rely on interconnected devices that need advanced reasoning capabilities. To address this, researchers are integrating real-time data and context into Large Language Models (LLMs). Traditional LLMs struggle with complex real-world tasks, leading to inaccurate results. The MARS Lab at NTU has developed a new IoT-LLM framework to improve how LLMs handle these challenges.
Challenges with Traditional Models
Conventional systems, including rule-based and basic AI models, face significant hurdles in processing IoT data. They often fail to capture context, leading to poor performance in tasks like activity recognition and machine diagnosis. For instance, traditional LLMs achieve only 40% accuracy in activity recognition. The IoT-LLM framework customizes LLMs for specific IoT tasks, enhancing their reasoning abilities through a three-step approach.
The Three Steps of the IoT-LLM Framework
- Preprocessing: Raw IoT data is transformed into formats that LLMs can easily understand, enriching the data with context.
- Commonsense Knowledge Activation: This step uses chain-of-thought prompting to simplify complex tasks, allowing LLMs to reason more like humans.
- IoT-Oriented Retrieval-Augmented Generation: LLMs dynamically retrieve context-specific information, enabling quick adaptation to real-time changes.
Results and Value
The integration of these steps has led to a significant improvement in LLM capabilities, achieving a task accuracy of 65%. This was validated through real-world tasks, such as heartbeat anomaly detection, demonstrating superior performance compared to traditional models.
Conclusion
The IoT-LLM framework effectively addresses the task-reasoning challenges in IoT contexts. By combining advanced prompting techniques and retrieval-augmented generation, it enhances LLMs’ ability to process IoT data. This innovation paves the way for future developments in self-operated systems, medical assistance, and smart city applications.
For more insights, check out the research paper and follow us on Twitter, join our Telegram Channel, and connect with us on LinkedIn. Don’t miss our newsletter and join our 50k+ ML SubReddit community.
Upcoming Live Webinar
Oct 29, 2024: Discover the best platform for serving fine-tuned models with the Predibase Inference Engine.
Transform Your Business with AI
Stay competitive by leveraging the IoT-LLM framework. Here’s how:
- Identify Automation Opportunities: Find key customer interactions that can benefit from AI.
- Define KPIs: Ensure measurable impacts on business outcomes.
- Select an AI Solution: Choose tools that fit your needs and allow customization.
- Implement Gradually: Start with a pilot program, gather data, and expand AI usage wisely.
For AI KPI management advice, contact us at hello@itinai.com. For continuous insights, follow us on Telegram or Twitter.
Explore how AI can transform your sales processes and customer engagement at itinai.com.