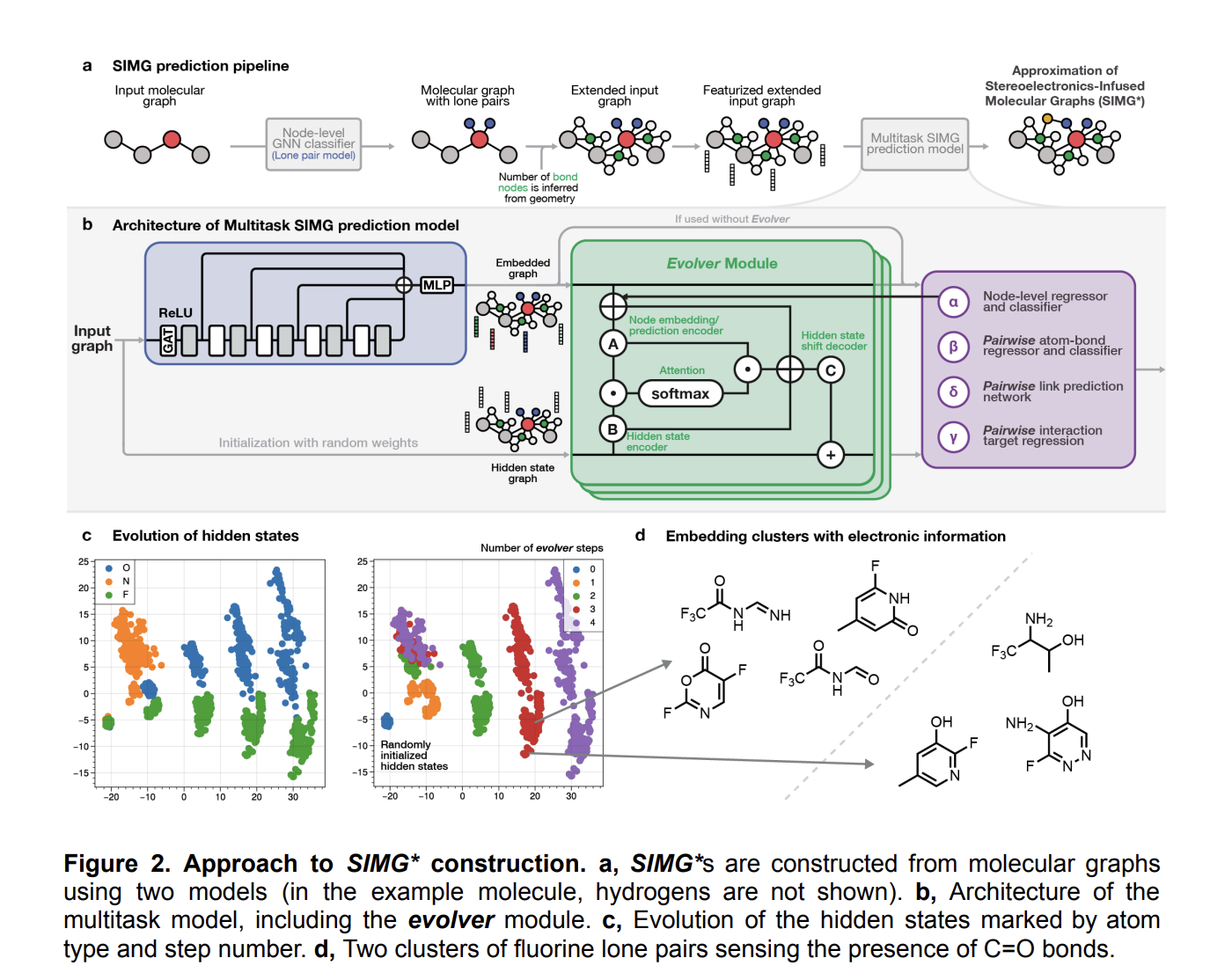
Enhancing Molecular Property Predictions with AI
Introduction
AI solutions struggle with traditional molecular representations due to their limitations. Our work introduces Stereo Electronics-Infused Molecular Graphs (SIMGs) to revolutionize the interpretation and performance of machine learning models in predicting molecular properties.
Practical Solutions
We address gaps by incorporating quantum-chemical interactions into molecular graphs, enhancing the understanding of molecular behavior and enabling the evaluation of previously intractable systems, such as entire proteins.
Value
Our model architecture and performance evaluation demonstrate exceptional predictive capabilities across various molecular properties, enabling detailed understanding of molecular behaviors. This approach facilitates high-throughput Natural Bond Orbital analysis, potentially accelerating theoretical chemistry research.
Conclusion and Future Scope
Our novel approach significantly enhances machine-learning model performance, enabling predictions for previously inaccessible molecules and expanding applications in drug discovery and materials science. Further exploration of stereoelectronic effects could lead to more sophisticated models, revolutionizing predictive capabilities in chemistry and related fields.
Check out the Paper and GitHub. All credit for this research goes to the researchers of this project.
Evolving with AI
Identify Automation Opportunities, Define KPIs, Select an AI Solution, and Implement Gradually to redefine your work processes with AI. Connect with us at hello@itinai.com for AI KPI management advice and continuous insights into leveraging AI.
Redefining Sales Processes and Customer Engagement
Discover how AI can redefine your sales processes and customer engagement at itinai.com.