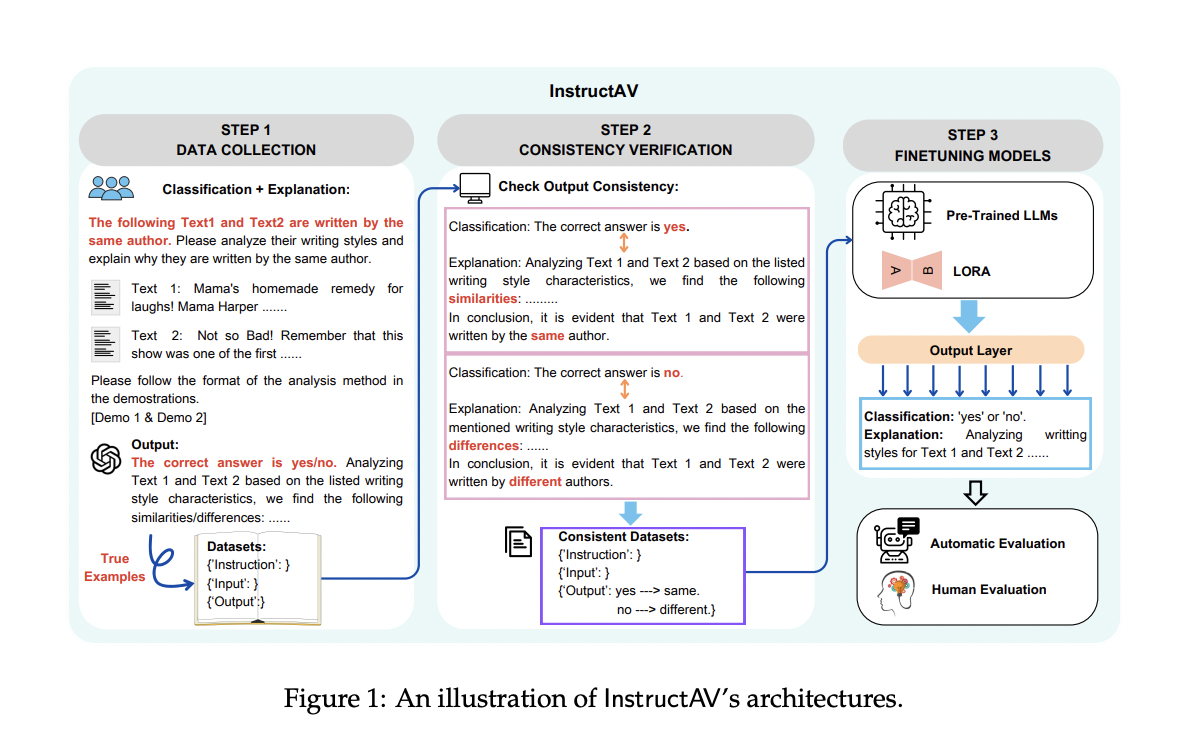
Authorship Verification with AI: Enhancing Accuracy and Explainability
Practical Solutions and Value
Authorship Verification (AV) is crucial in natural language processing (NLP) for determining whether two texts share the same authorship. Traditional approaches relied on stylometric analysis, but modern deep learning models like BERT and RoBERTa offer superior performance.
The primary challenge in AV is to accurately determine authorship and provide clear explanations for classification decisions. The lack of explainability in current AV models is a gap in academic interest and a practical concern. Therefore, AV models must be accurate and interpretable, providing detailed insights into their decision-making processes.
The InstructAV framework, developed by the Information Systems Technology and Design research team, aims to enhance accuracy and explainability in authorship verification tasks. It integrates explainability directly into the classification process, ensuring that the models make accurate predictions and provide deep insights into their decision-making logic.
The performance of InstructAV was evaluated through comprehensive experiments across diverse AV datasets, demonstrating state-of-the-art accuracy and setting new benchmarks in generating coherent and substantiated explanations for its findings.
AI Solutions for Business Transformation
Identify Automation Opportunities: Locate key customer interaction points that can benefit from AI.
Define KPIs: Ensure your AI endeavors have measurable impacts on business outcomes.
Select an AI Solution: Choose tools that align with your needs and provide customization.
Implement Gradually: Start with a pilot, gather data, and expand AI usage judiciously.
For AI KPI management advice, connect with us at hello@itinai.com. For continuous insights into leveraging AI, stay tuned on our Telegram channel or Twitter.
AI for Sales Processes and Customer Engagement
Discover how AI can redefine your sales processes and customer engagement. Explore solutions at itinai.com.