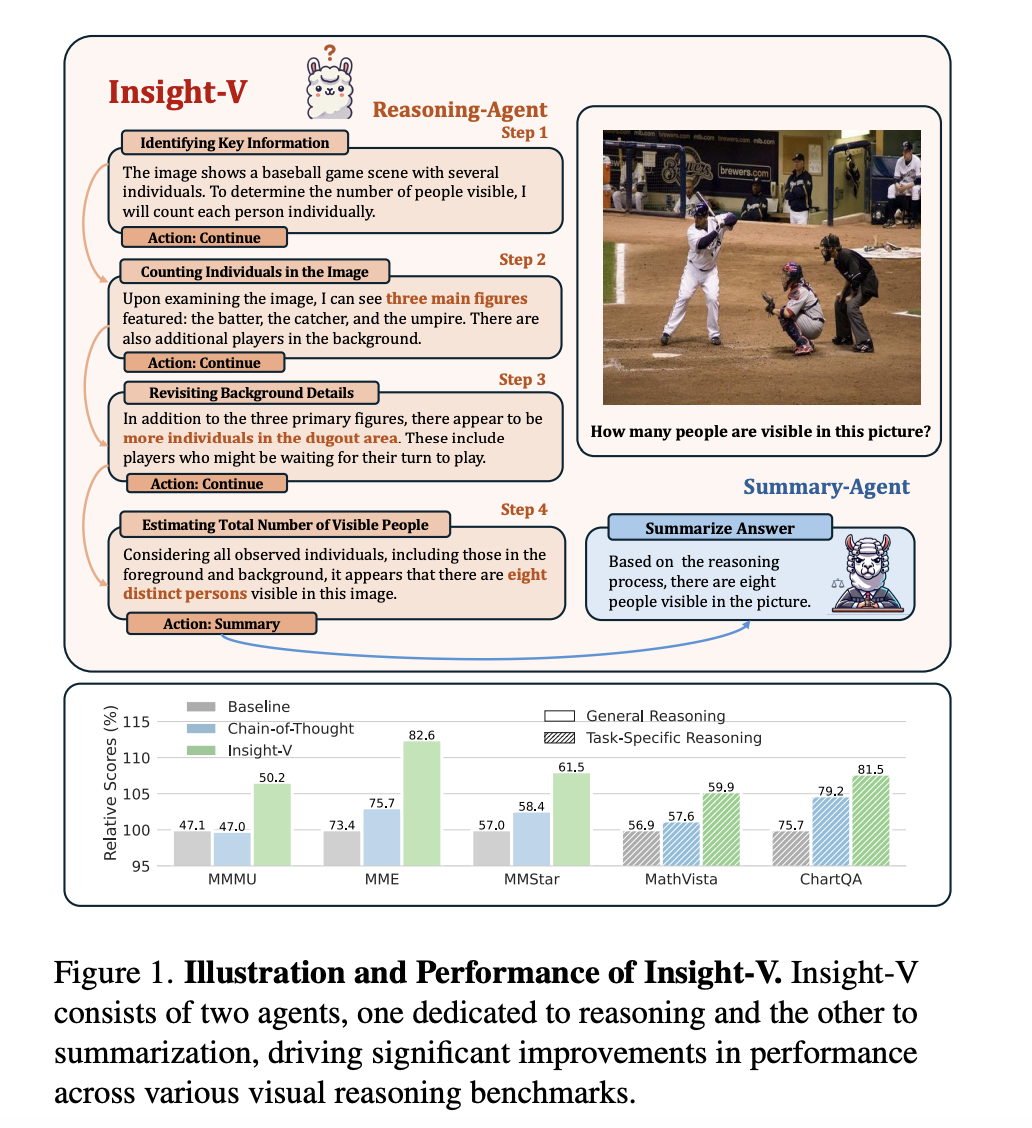
Understanding Multimodal Large Language Models (MLLMs)
Challenges in AI Reasoning
The ability of MLLMs to reason using both text and images presents significant challenges. While tasks focused solely on text are improving, those involving images struggle due to a lack of comprehensive datasets and effective training methods. This hinders their use in practical applications like autonomous systems, medical diagnosis, and educational tools.
Limitations of Traditional Approaches
Current methods to improve reasoning mainly include Chain-of-Thought (CoT) prompting and structured datasets. However, these strategies have major downsides:
– Creating annotated datasets for visual reasoning is costly and labor-intensive.
– Single-step reasoning often leads to fragmented and illogical results.
– The absence of diverse datasets limits generalization across different tasks.
Introducing Insight-V
Innovative Solutions Through Collaborative Framework
Researchers from NTU, Tencent, Tsinghua University, and Nanjing University developed Insight-V to overcome these challenges. Here’s how it works:
– **Scalable Data Generation**: Insight-V generates diverse reasoning pathways that maintain coherence and quality.
– **Multi-Agent System**: It uses two agents:
– **Reasoning Agent**: Creates detailed logical steps.
– **Summary Agent**: Validates and refines these steps to reduce errors.
– **Reinforcement Learning**: By using Iterative Direct Preference Optimization (DPO), it aligns outputs with human judgment, significantly improving reasoning accuracy.
Robust Training Dataset
Insight-V is built on a dataset containing over 200,000 reasoning samples and 1.2 million summarization examples. The training process includes:
– Role-specific supervised fine-tuning.
– Iterative preference optimization to enhance alignment with human decision-making.
This structured approach promotes effective generalization across various reasoning tasks.
Performance and Impact
Significant Improvements
Insight-V shows a remarkable mean relative improvement of 7.0% over previous models in benchmark tasks. This includes enhancements in areas like:
– Detailed analysis of charts.
– Mathematical reasoning.
– General perception tasks like TextVQA.
These improvements confirm the effectiveness of the system in tackling complex reasoning tasks.
A Future-Focused Framework
Insight-V presents a transformative approach for multi-modal reasoning by combining innovative data generation with a collaborative architecture. It prepares MLLMs to handle reasoning-intensive tasks efficiently and adapt across different fields.
Get Involved and Explore More
For in-depth insights, check out the Paper and GitHub Page. Follow us on Twitter, join our Telegram Channel, and connect with our LinkedIn Group. If you appreciate our work, subscribe to our newsletter and engage with our thriving ML SubReddit community.
Upcoming Event
Don’t miss our FREE AI VIRTUAL CONFERENCE, SmallCon, on December 11th. Join industry leaders from Meta, Salesforce, and more to learn about building powerful models.
Enhance Your Business with AI
To leverage Insight-V for your company:
– **Identify Opportunities**: Find key areas for AI integration.
– **Set Measurable Goals**: Define KPIs for tracking impact.
– **Choose Suitable Tools**: Select AI solutions tailored to your needs.
– **Implement in Phases**: Start small, gather insights, and expand effectively.
For AI KPI management advice, reach out to us at hello@itinai.com. Stay updated on AI trends via our Telegram or Twitter. Explore the possibilities at itinai.com.