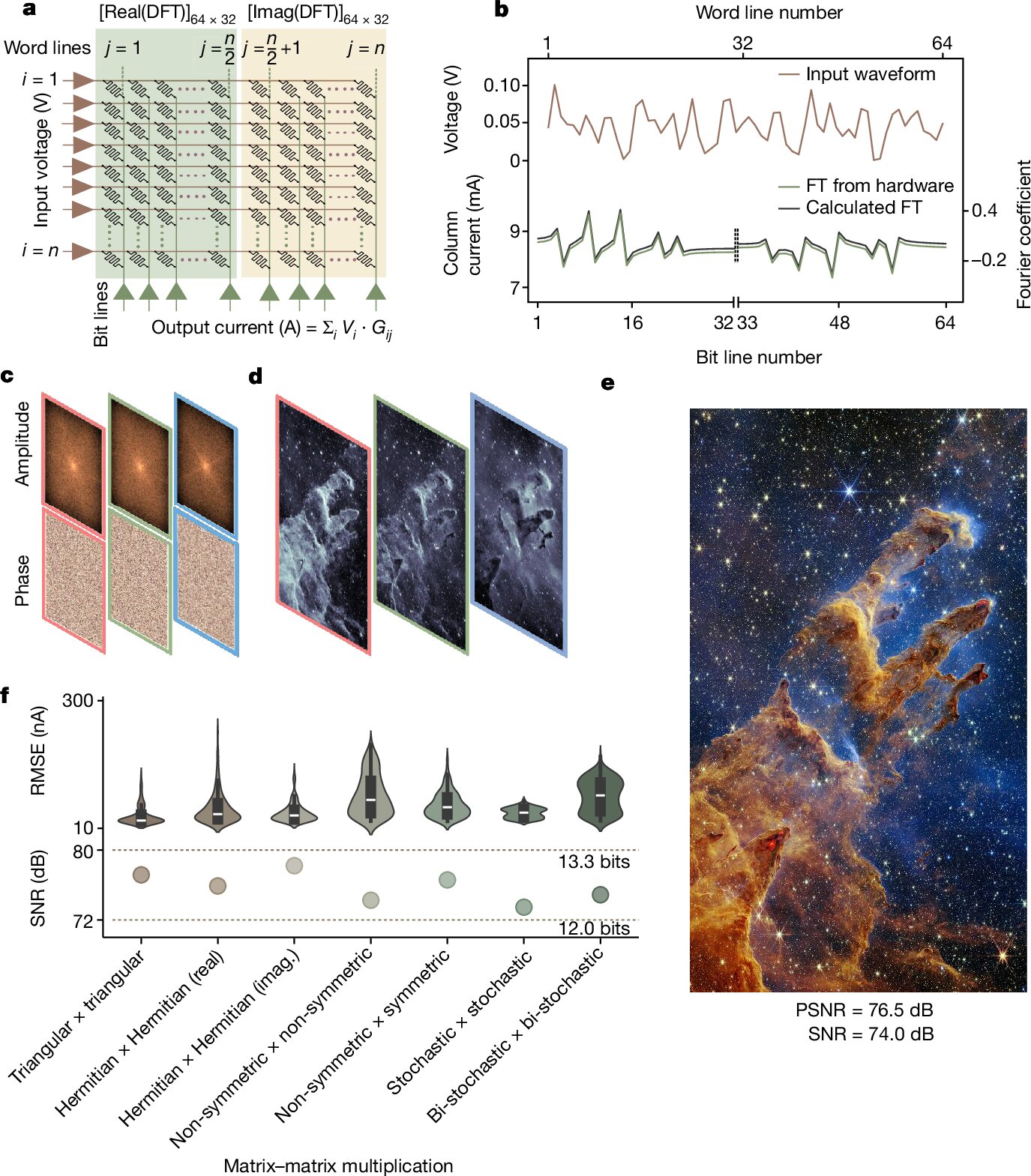
Practical Solutions for AI Hardware Development
Energy Efficiency and Computational Speed
Traditional computing systems face limitations in energy efficiency and computational speed. New hardware architectures are needed for complex tasks like AI model training.
Current Challenges
Current approaches rely on resource-intensive data centers, making AI model training inaccessible to small-scale users. Neuromorphic computing has faced technical hurdles in fully utilizing analog computing systems.
Breakthrough in Neuromorphic Computing
A team at the Indian Institute of Science (IISc) developed a brain-inspired analog computing platform capable of storing and processing data in 16,500 distinct conductance states within a molecular film.
Advancements and Impact
The platform drastically reduces the energy and time required for matrix multiplication, making AI acceleration faster and more efficient. It also integrates memory and processing functions, improving energy efficiency and streamlining complex AI tasks.
Implications and Future Prospects
The platform’s advancements could democratize AI by enabling complex tasks to be performed on smaller, more accessible devices. Ongoing efforts to develop an indigenous neuromorphic chip hold promise for revolutionizing industrial and consumer applications.
AI Implementation Guidance
For companies looking to evolve with AI, practical steps include identifying automation opportunities, defining measurable impacts, selecting suitable AI solutions, and implementing AI usage judiciously.
AI KPI Management and Sales Processes
For AI KPI management advice and insights into leveraging AI for sales processes and customer engagement, connect with us at hello@itinai.com or stay tuned on our Telegram t.me/itinainews or Twitter @itinaicom.