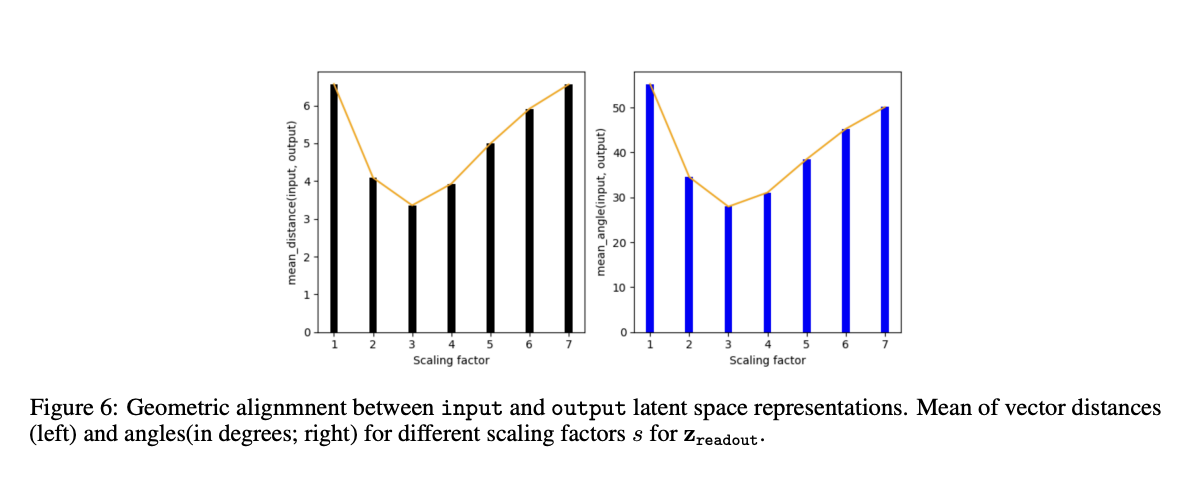
Practical Solutions for Mitigating Hallucinations in Large Language Models (LLMs)
Addressing the Challenge
Large language models (LLMs) are essential in various applications, but they often produce unreliable content due to hallucinations. This undermines their trustworthiness, especially in sensitive domains like medical and legal documents.
Effective Methods
Researchers have explored methods like model editing and context-grounding to reduce hallucinations. However, these approaches have limitations, such as increased computational complexity and the need for extensive retraining.
Introducing Larimar
A team of researchers from IBM Research and T. J. Watson Research Center has introduced a novel method leveraging the memory-augmented LLM named Larimar. This model integrates an external episodic memory controller to enhance text generation capabilities, reducing the chances of generating hallucinated content.
Superior Performance
The Larimar model demonstrated superior performance in experiments compared to existing methods, showcasing substantial improvements in generating factual content and offering a promising solution by utilizing lightweight memory operations.
Practical Value
Larimar’s method simplifies the process and ensures better performance and accuracy, showcasing a substantial speed advantage and ensuring higher factual accuracy in generated text.
Conclusion and Next Steps
The research from IBM Research and T. J. Watson Research Center highlights a novel and efficient method to address hallucinations in LLMs, paving the way for more trustworthy applications of LLMs across various critical fields.
AI Solutions for Your Business
Discover how AI can redefine your way of work and sales processes. Identify Automation Opportunities, Define KPIs, Select an AI Solution, and Implement Gradually. For AI KPI management advice, connect with us at hello@itinai.com.