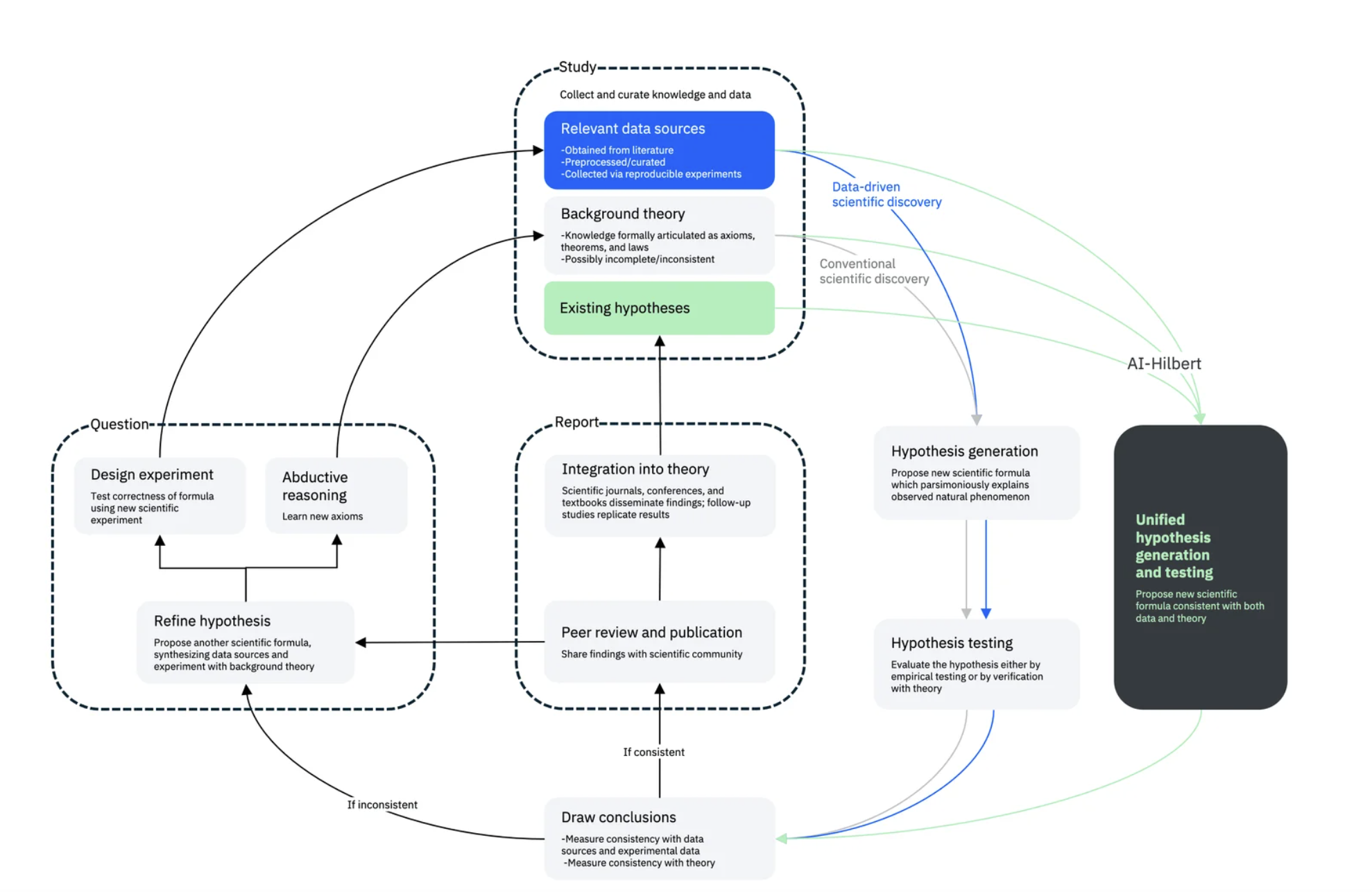
Practical Solutions for Scientific Discovery
Integrating Background Knowledge with Experimental Data
Recent advances in global optimization methods offer promising tools for scientific discovery by integrating background knowledge with experimental data.
Derive Well-Known Laws with Guaranteed Results
A solution proposed by researchers from Imperial College Business School, Samsung AI, and IBM can derive well-known scientific laws from hypotheses and data, ensuring consistency with background theory and experimental data. Unlike deep learning methods, this approach guarantees scalable and reliable discovery of new scientific laws.
AI-Hilbert: A Paradigm for Scientific Discovery
AI-Hilbert identifies polynomial laws consistent with experimental data and a background knowledge base of polynomial equalities and inequalities. It ensures that discovered laws are axiomatically correct given the background theory and helps address inconsistent or incomplete theories.
Innovative Method for Scientific Discovery
An innovative method integrates real algebraic geometry and mixed-integer optimization to derive new scientific laws from incomplete axioms and noisy data. This approach combines both theory and data, enabling discoveries in data-scarce and theory-limited contexts.
Value of AI-Hilbert for Businesses
Evolve Your Company with AI
AI-Hilbert offers an innovative machine learning framework for scientific discovery, enabling businesses to stay competitive and redefine their way of work.
Identify Automation Opportunities
AI solutions can help businesses locate key customer interaction points that can benefit from AI, leading to automation opportunities.
Implement Gradually and Connect with Us
Businesses can start with a pilot, gather data, and expand AI usage judiciously. For AI KPI management advice and continuous insights into leveraging AI, businesses can connect with a dedicated AI expert at hello@itinai.com or stay tuned on our Telegram t.me/itinainews or Twitter @itinaicom.