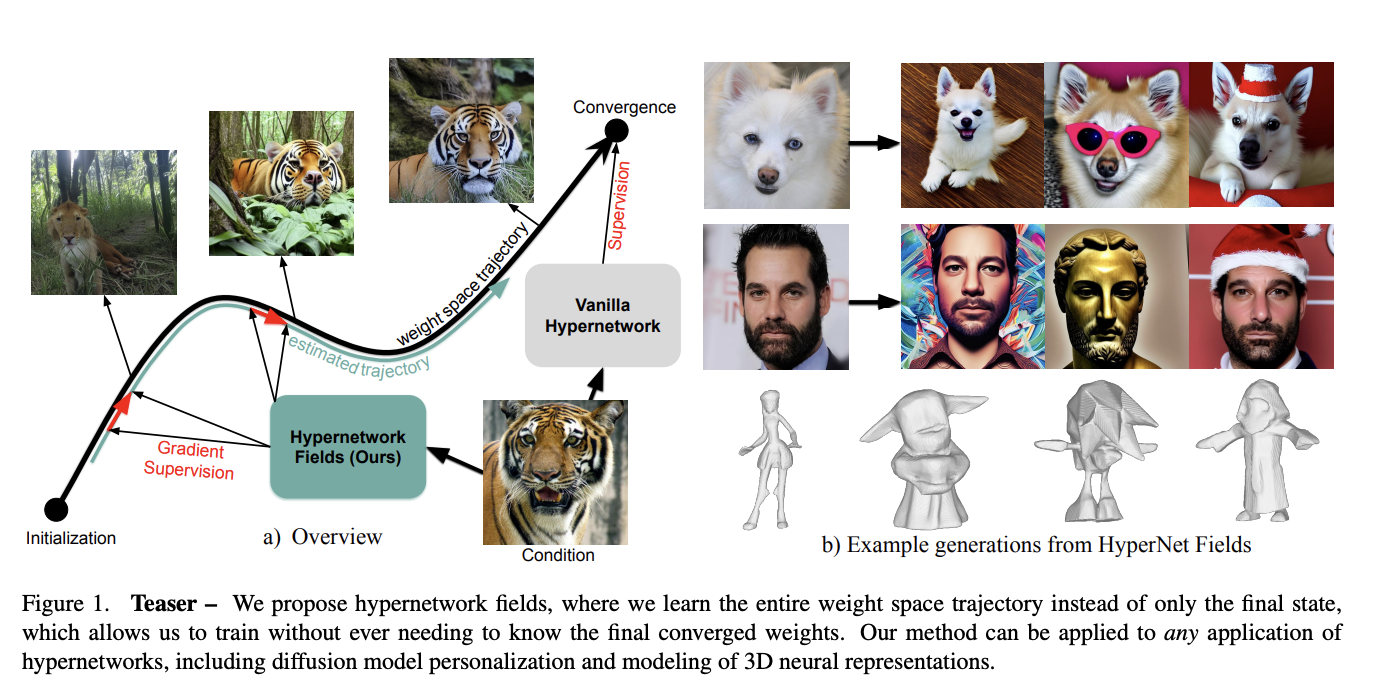
Understanding Hypernetworks and Their Benefits
Hypernetworks are innovative tools that help adapt large models and train generative models efficiently. However, traditional training methods can be time-consuming and require extensive computational resources due to the need for precomputed optimized weights for each data sample.
Challenges with Current Methods
Current approaches often assume a direct one-to-one relationship between input samples and their optimized weights. This can limit the flexibility and expressiveness of hypernetworks. To overcome these challenges, researchers are working on methods that reduce the need for extensive precomputation, allowing for faster and more scalable training.
Advancements in Hypernetwork Training
Recent developments have introduced gradient-based supervision in hypernetwork training. This method removes the dependency on precomputed weights while ensuring stability and scalability. By guiding the learning process through gradients, hypernetworks can efficiently transition through weight spaces, inspired by generative models.
Introducing the Hypernetwork Field
Researchers from the University of British Columbia and Qualcomm AI Research have proposed a new method called the Hypernetwork Field. This approach models the entire optimization process of task-specific networks, allowing the hypernetwork to estimate weights at any point during training. This eliminates the need for precomputed targets, significantly reducing training costs while achieving competitive results in tasks like personalized image generation and 3D shape reconstruction.
Key Features of the Hypernetwork Field
The Hypernetwork Field captures the complete training dynamics, enabling accurate weight predictions without repetitive optimization for each sample. This method is not only computationally efficient but also delivers strong performance in various applications.
Practical Applications and Results
Experiments have shown the effectiveness of the Hypernetwork Field in personalized image generation and 3D shape reconstruction. It personalizes images using conditioning tokens and achieves faster training and inference compared to traditional methods. The framework also successfully predicts weights for 3D shape reconstruction, significantly reducing computational costs while maintaining high-quality outputs.
Conclusion
The Hypernetwork Field offers a groundbreaking approach to training hypernetworks efficiently. By modeling the entire optimization trajectory and using gradient supervision, it eliminates the need for precomputed weights while ensuring competitive performance. This method is adaptable, reduces computational overhead, and can scale to various tasks and larger datasets.
Get Involved and Learn More
For more insights, check out the research paper and follow us on Twitter, join our Telegram Channel, and LinkedIn Group. Don’t forget to join our 60k+ ML SubReddit.
Transform Your Business with AI
Explore how Hypernetwork Fields can enhance your company’s AI capabilities:
- Identify Automation Opportunities: Find key areas in customer interactions that can benefit from AI.
- Define KPIs: Ensure measurable impacts from your AI initiatives.
- Select an AI Solution: Choose tools that fit your needs and allow for customization.
- Implement Gradually: Start small, gather data, and expand AI usage wisely.
For AI KPI management advice, connect with us at hello@itinai.com. For continuous insights, follow us on Telegram or Twitter @itinaicom.
Discover how AI can transform your sales processes and customer engagement at itinai.com.