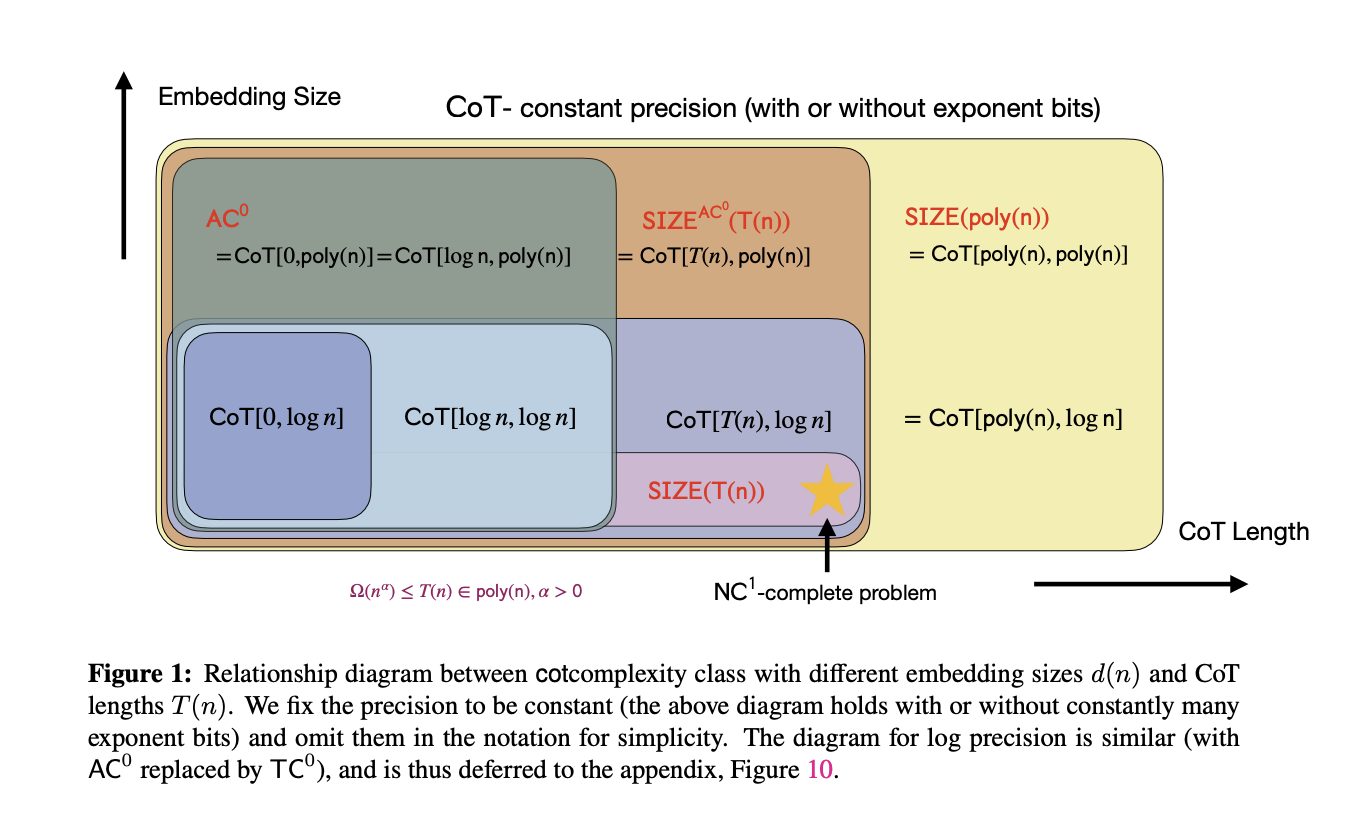
Large Language Models and Advanced Reasoning
Large Language Models (LLMs) like GPT-3 and ChatGPT excel in complex reasoning tasks like mathematical problem-solving and code generation, surpassing standard machine learning techniques. The key to unlocking these abilities lies in the “chain of thought” (CoT), allowing models to generate intermediate reasoning steps before arriving at the final answer, similar to how humans break down problems into smaller steps.
Practical Solution and Value
The “chain of thought” approach significantly enhances the reasoning capabilities of transformer models like GPT-3, enabling them to handle complex tasks requiring sequential logic that parallel models would struggle with.
Understanding the Power of “Chain of Thought”
Even generating incorrect or random intermediate steps improves the model’s reasoning ability. This approach allows transformers to perform more serial computations, making them powerful enough to solve almost any computationally hard problem, at least from a theoretical standpoint.
Practical Implementation of AI Solutions
- Identify Automation Opportunities: Locate key customer interaction points that can benefit from AI.
- Define KPIs: Ensure AI endeavors have measurable impacts on business outcomes.
- Select an AI Solution: Choose tools aligned with your needs and provide customization.
- Implement Gradually: Start with a pilot, gather data, and expand AI usage judiciously.
Spotlight on a Practical AI Solution
Consider the AI Sales Bot from itinai.com/aisalesbot, designed to automate customer engagement 24/7 and manage interactions across all customer journey stages.