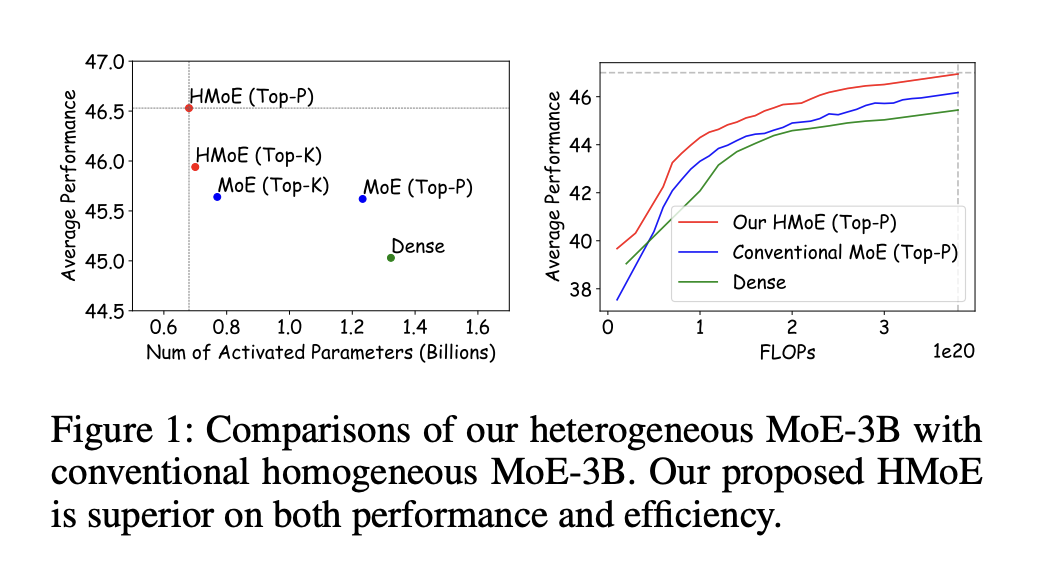
The Heterogeneous Mixture of Experts (HMoE) Model: Optimizing Efficiency and Performance
The HMoE model introduces experts of varying sizes to handle diverse token complexities, improving resource utilization and overall model performance. The research proposes a new training objective to prioritize the activation of smaller experts, enhancing computational efficiency.
Key Findings:
- HMoE outperforms traditional homogeneous MoE models, achieving lower loss with fewer activated parameters.
- Benefits are more pronounced as training progresses and computational resources increase.
- The model effectively allocates tokens based on complexity, with smaller experts handling general tasks and larger experts specializing in more complex ones.
Practical Solutions and Value:
- The HMoE model offers improved efficiency and performance by utilizing diverse expert capacities.
- It provides better task-specific specialization and efficient use of resources, leading to enhanced model effectiveness.
- This approach opens up new possibilities for large language model development, with potential applications in diverse natural language processing tasks.
AI Integration Guidance:
To evolve your company with AI and stay competitive, consider integrating the HMoE model:
– Identify Automation Opportunities
– Define Measurable KPIs
– Select Customizable AI Solutions
– Implement Gradually and Expand Usage
For AI KPI management advice and continuous insights into leveraging AI, connect with us at hello@itinai.com or follow us on Telegram and Twitter.