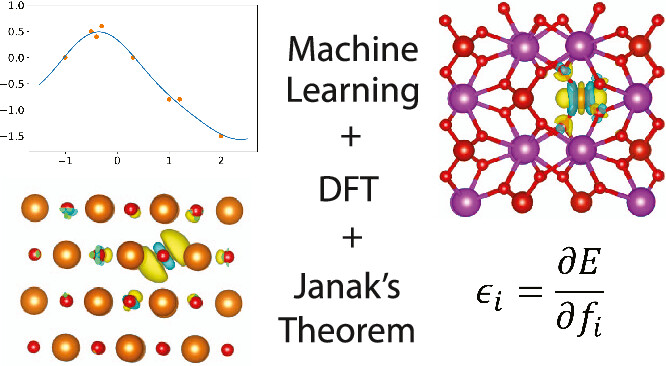
Enhancing Density Functional Theory Accuracy with Machine Learning
Practical Solutions and Value
One of the core challenges in semilocal density functional theory (DFT) is the consistent underestimation of band gaps, hindering accurate prediction of electronic properties and charge transfer mechanisms. Hybrid DFT and machine learning approaches offer improved band gap predictions, addressing self-interaction errors and enhancing precision.
Harvard SEAS researchers have developed a machine learning method using Gaussian processes to improve the precision of density functionals for predicting energy gaps and reaction energies. This advancement builds upon the CIDER framework known for its efficiency and scalability in handling large systems.
The study introduces key concepts and methods for fitting exchange-correlation (XC) functionals in DFT, focusing on band gap prediction and single-particle energies. The CIDER24X exchange energy model was developed using a Gaussian process, enhancing flexibility and predictive accuracy for molecular energies and HOMO-LUMO gaps.
In conclusion, the study presents a framework for fitting density functionals to both total energy and single-particle energy levels using machine learning, offering the potential for efficient and accurate electronic property predictions across diverse systems.
Practical Implementation and Evolution with AI
Discover how AI can redefine your way of work and sales processes, identifying automation opportunities, defining KPIs, selecting AI solutions, and implementing AI gradually. For AI KPI management advice and continuous insights into leveraging AI, connect with us at hello@itinai.com and stay tuned on our Telegram and Twitter channels.
Explore how AI can redefine your sales processes and customer engagement at itinai.com.