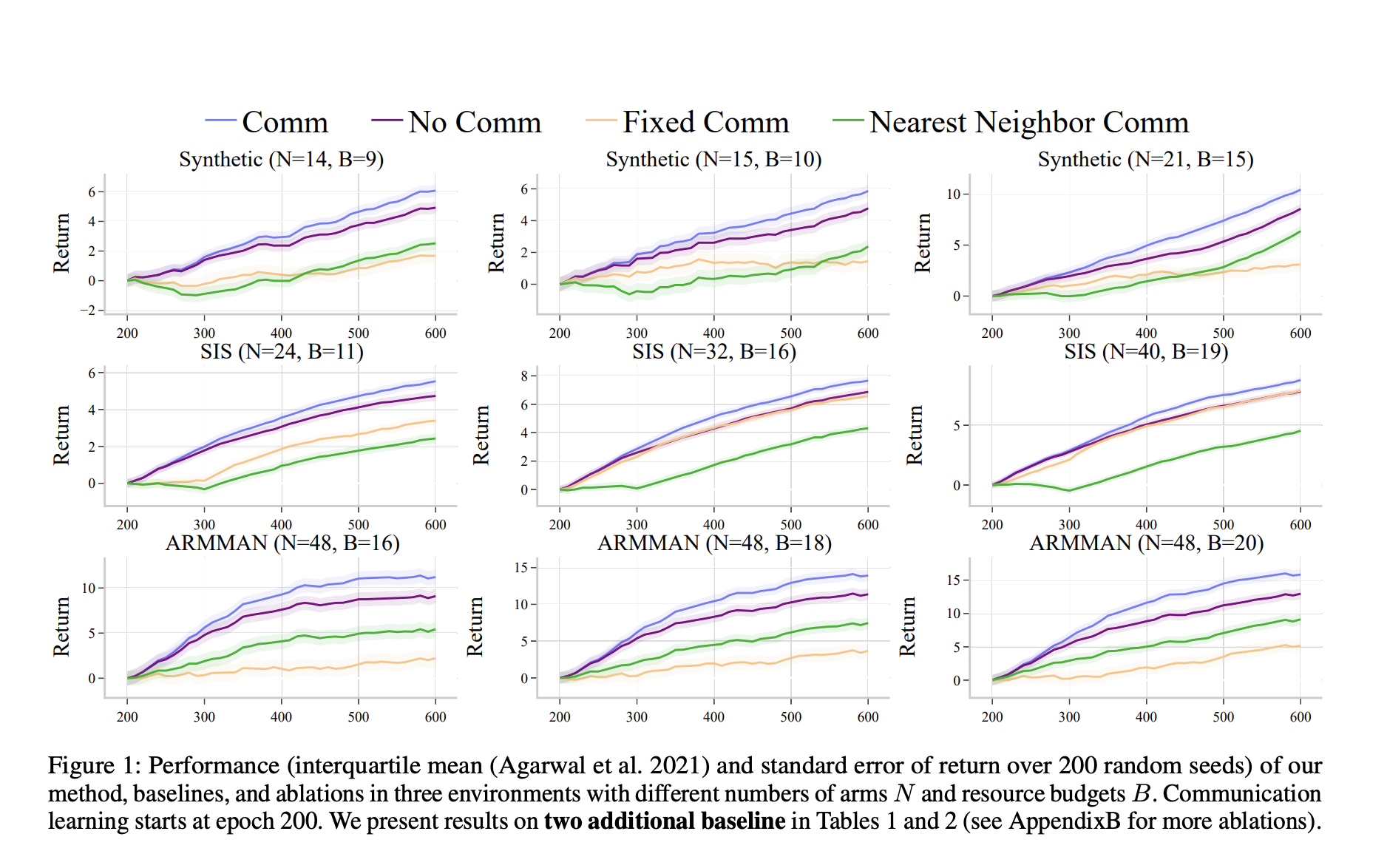
Practical Solutions for Noisy Restless Multi-Arm Bandits
Overview
The Restless Multi-Arm Bandit (RMAB) model offers practical solutions for resource allocation in various fields such as healthcare, online advertising, and conservation. However, challenges arise due to systematic data errors affecting efficient implementation.
Challenges and Solutions
Systematic data errors impact the performance of RMAB methods, leading to suboptimal decisions. To address this, researchers at Harvard University and Google proposed a communication learning approach. This approach allows arms in the RMAB to share Q-function parameters and learn from each other’s experiences, reducing the impact of systematic data errors and improving overall efficiency of resource allocation decisions.
Value and Application
Empirical testing has demonstrated that the proposed communication learning method outperforms existing methods and offers greater robustness and adaptability to real-world challenges. This advancement in RMAB technology has the potential to revolutionize resource allocation problems in various fields, from healthcare to public policy, leading to more efficient and effective decision-making processes.
Conclusion
The groundbreaking communication learning algorithm significantly enhances the performance of RMABs in noisy environments, offering a practical solution to address resource allocation challenges across various domains.
AI Integration for Business Transformation
Discover how AI can redefine your way of work and sales processes. Connect with us for AI KPI management advice and continuous insights into leveraging AI.