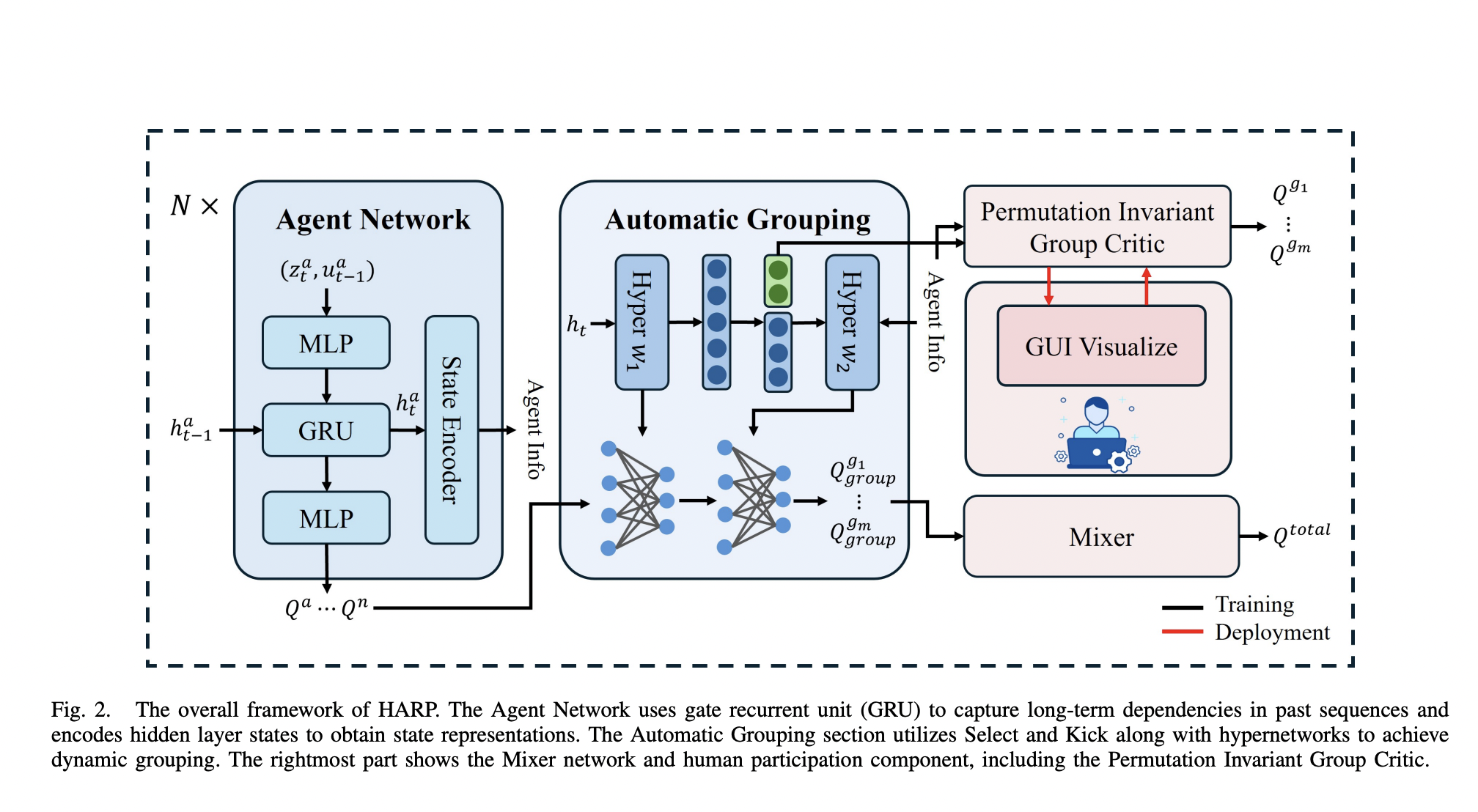
Practical Solutions and Value of HARP in Multi-Agent Reinforcement Learning
Introduction to MARL and Its Challenges
Multi-agent reinforcement learning (MARL) focuses on systems where multiple agents collaborate to tackle tasks beyond individual capabilities. It is crucial in autonomous vehicles, robotics, and gaming. Challenges include coordination difficulties and the need for human expertise.
Existing Methods and Their Limitations
Current methods like RODE and GACG aim to enhance agent collaboration but face issues with adaptability and human intervention. They lack flexibility in real-world applications.
HARP Framework Overview
HARP allows agents to regroup dynamically with minimal human intervention. It combines automatic grouping during training and human-assisted regrouping during deployment, bridging the gap between automation and human guidance.
Performance and Results
HARP outperformed traditional methods in cooperative environments, achieving a 100% win rate in various difficulty levels. It significantly improved agent performance and adaptability, showcasing its effectiveness in complex scenarios.
Conclusion and Impact
HARP reduces the need for continuous human involvement during training while enhancing performance through human input during deployment. It addresses challenges of low sample efficiency and poor generalization, offering a scalable solution for multi-agent coordination.