The tutorial discusses efficient dataset sampling techniques in Python. It compares three methods: uniform, random, and Latin Hypercube Sampling (LHS). Uniform sampling is simple but scales poorly with dimensions. Random sampling is straightforward, better for large dimensions, yet may form clusters. LHS offers stratified random samples, preferable for high dimensions with fewer samples, albeit more complex. Code examples are given. The method choice depends on the goal. The author invites engagement through LinkedIn, a newsletter subscription, or a referred membership for unlimited content access.
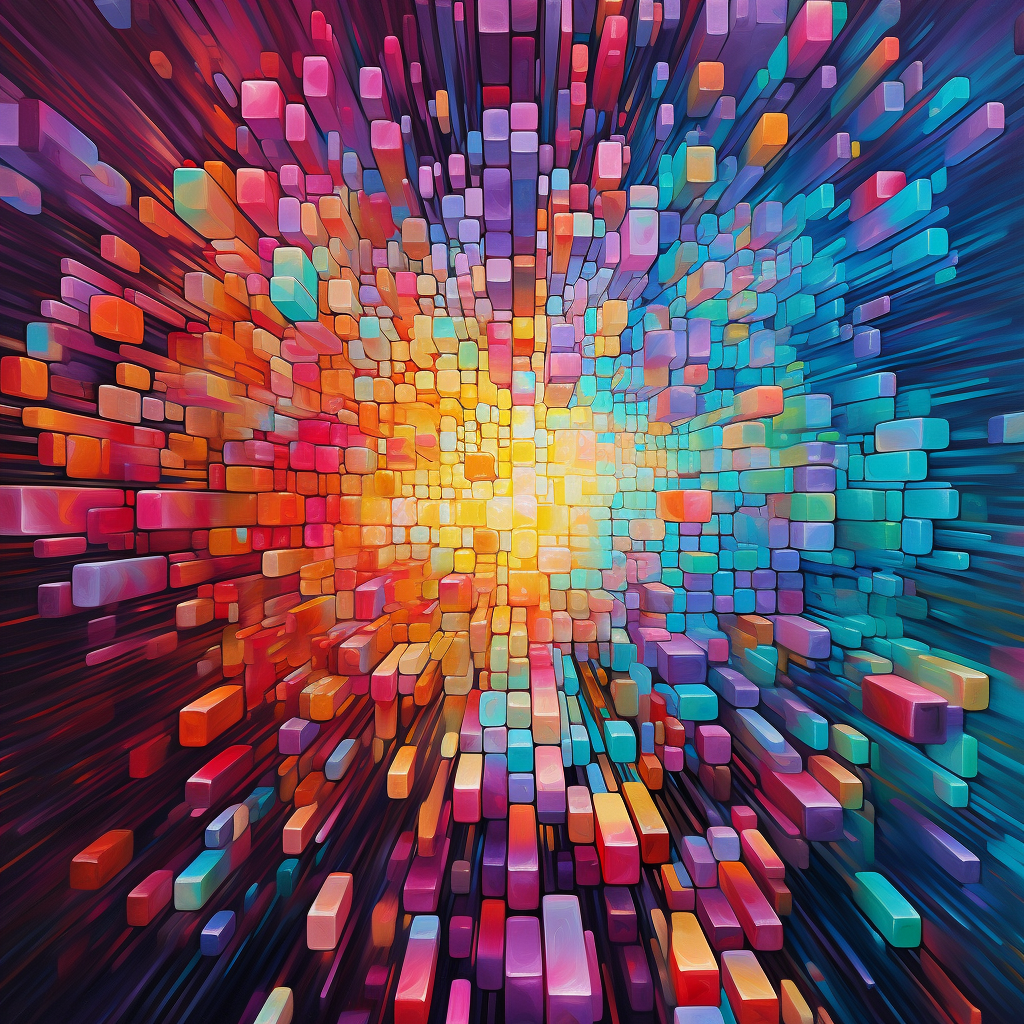
“`html
Efficient Data Sampling Techniques in Python: A Practical Guide for Managers
Practical AI Solutions: Enhance your company’s efficiency with AI-driven data sampling techniques. Discover how to stay ahead in the competitive market by leveraging Python for smarter data management.
Introduction to Data Sampling
Data sampling is a critical step in machine learning. It’s like decorating a Christmas tree; you can place the ornaments (data points) uniformly, randomly, or using a method called Latin Hypercube. Each method has its own benefits and challenges.
Scenario 1: Fixed Datasets
When you’re given a dataset, like predicting New York house prices, you might face unbalanced data or missing values. You’ll need to clean and process this data before use.
Scenario 2: Controlled Experiments
If you can generate data, like in a lab, you have more control. You can ensure the quality of your data and repeat experiments if necessary.
Sampling Methods
Choosing the right sampling method is crucial for efficient data analysis. Here are three common methods:
1. Uniform Sampling
Pros: Simple to implement and ensures even coverage of the parameter space.
Cons: Not scalable for high dimensions due to exponential growth in data points.
2. Random Sampling
Pros: Easy to understand and captures output space complexity well.
Cons: Can lead to data clustering and uneven exploration of the parameter space.
3. Latin Hypercube Sampling
Pros: Provides a balanced random sampling and is ideal for high dimensions with fewer samples.
Cons: More complex to implement and understand, requiring domain knowledge.
Conclusion
No single method is perfect. The choice depends on your specific goals and the nature of your data.
Take Your Company Forward with AI
Identify where AI can automate customer interactions, define measurable goals, select the right AI tools, and implement them step by step.
AI Sales Bot: A Highlighted Solution
Explore the AI Sales Bot at itinai.com/aisalesbot, which automates customer engagement around the clock, enhancing your sales processes and customer service.
For personalized AI KPI management advice, reach out to us at hello@itinai.com. Stay updated with AI insights on our Telegram t.me/itinainews or Twitter @itinaicom.
“`