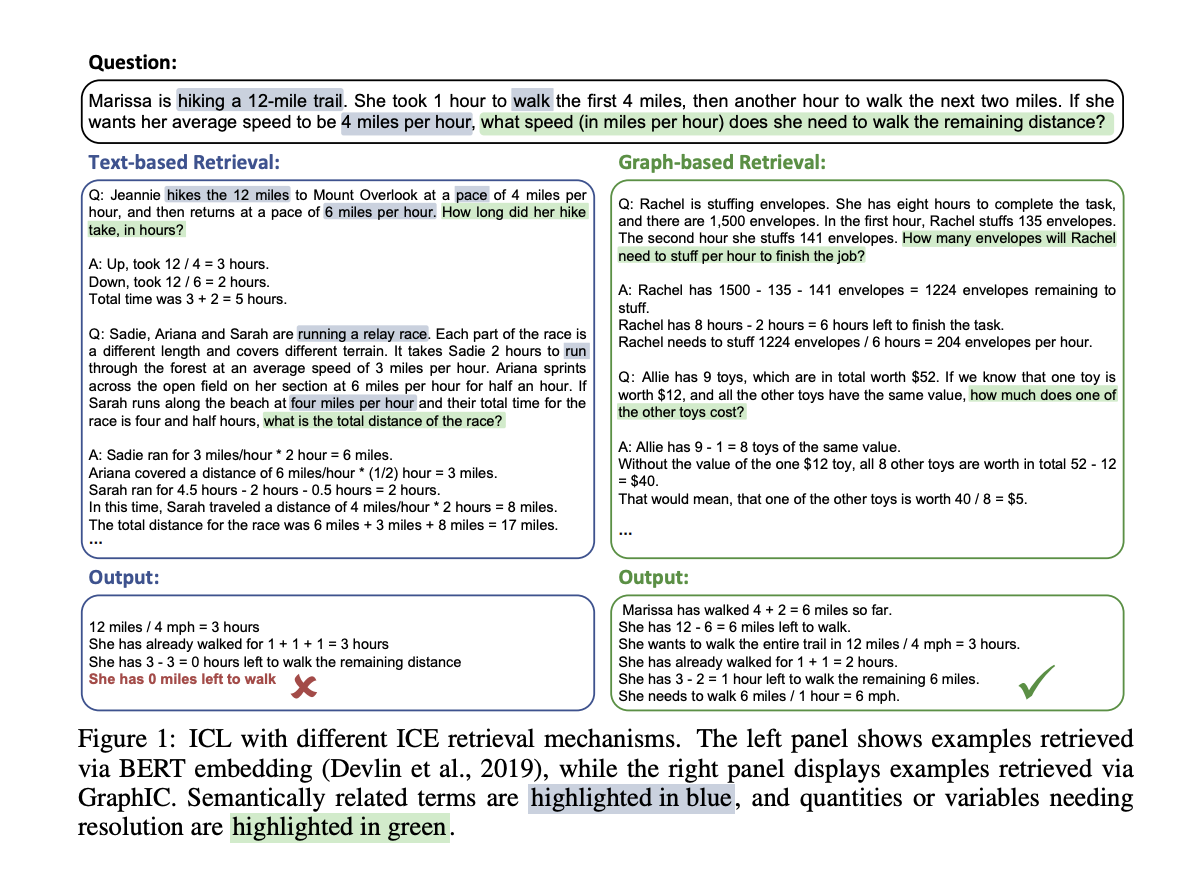
GraphIC: Enhancing Example Selection with Graph-based Models
Practical Solutions and Value
In the realm of artificial intelligence, GraphIC introduces a novel approach for selecting In-Context Examples (ICE) by leveraging graph-based representations and Bayesian Networks. This innovative method aims to improve Language Model Models (LLMs) performance on multi-step reasoning tasks, particularly in domains like math and logic.
GraphIC’s key contribution lies in its ability to capture intricate human-like cognitive processes through thought graphs and Bayesian Networks. By modeling thought dependencies and utilizing personalized PageRank mechanisms, this method excels in selecting ICEs that align with the core reasoning of a given query, even if they lack semantic similarity.
Through experimental evaluations on challenging tasks such as mathematical reasoning, logical reasoning, and code generation, GraphIC consistently outperforms existing training-free and training-based models. The results showcase significant gains in efficiency and effectiveness, highlighting the supremacy of graph-based representations in enhancing example selection for complex reasoning functions.
For businesses seeking to harness the power of AI, GraphIC offers a transformative way to enhance decision-making processes and streamline reasoning tasks. By employing GraphIC’s methodology, organizations can expect improved performance in AI-driven functions, setting the stage for enhanced competitiveness and efficiency in the AI landscape.