Prompting techniques like chain of thought (CoT) and tree of thought (ToT) have drastically improved the problem-solving capabilities of large language models (LLMs). However, they assume linear reasoning, in contrast to the non-linear patterns characteristic of human reasoning. A new approach, called graph-of-thought reasoning (GOTR), models reasoning processes as a graph structure that captures non-sequential human thinking, leading to better reasoning capabilities. Conversely, another method, Graph of Thought (GoT) prompting, models each thought generated by an LLM as a node within a graph, using vertices to represent thought dependencies. Despite its benefits, these approaches may be more complex and costly, providing notable improvements primarily for specific types of problems.
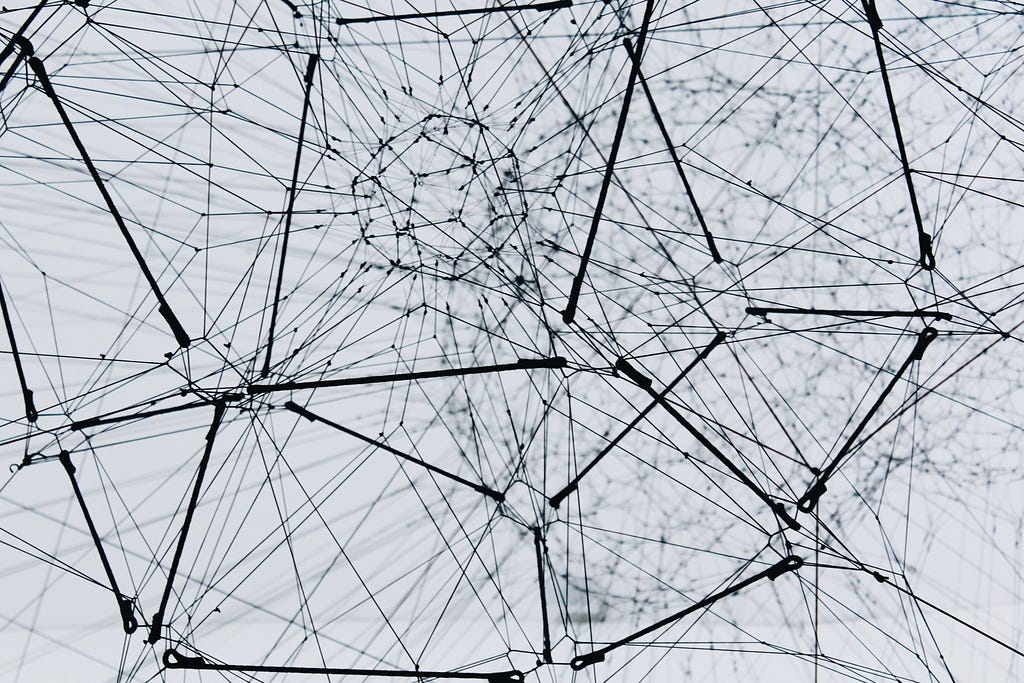
Graph-Based Prompting and Reasoning with Language Models
Introduction
AI solutions are evolving to redefine the way businesses work and stay competitive. Graph-Based Prompting and Reasoning with Language Models provide practical opportunities to automate key customer interactions, define measurable KPIs, select appropriate AI solutions, and implement AI gradually. For more insights into leveraging AI, follow our updates on Telegram and Twitter.
Practical AI Solution Spotlight: AI Sales Bot
Explore the AI Sales Bot, an innovative tool designed to automate customer engagement 24/7 and manage interactions across all stages of the customer journey. Learn more at itinai.com/aisalesbot.
For AI KPI management advice and continuous insights into leveraging AI, connect with us at hello@itinai.com.
List of Useful Links:
- AI Lab in Telegram @aiscrumbot – free consultation
- Graph-Based Prompting and Reasoning with Language Models
- Towards Data Science – Medium
- Twitter –Β @itinaicom