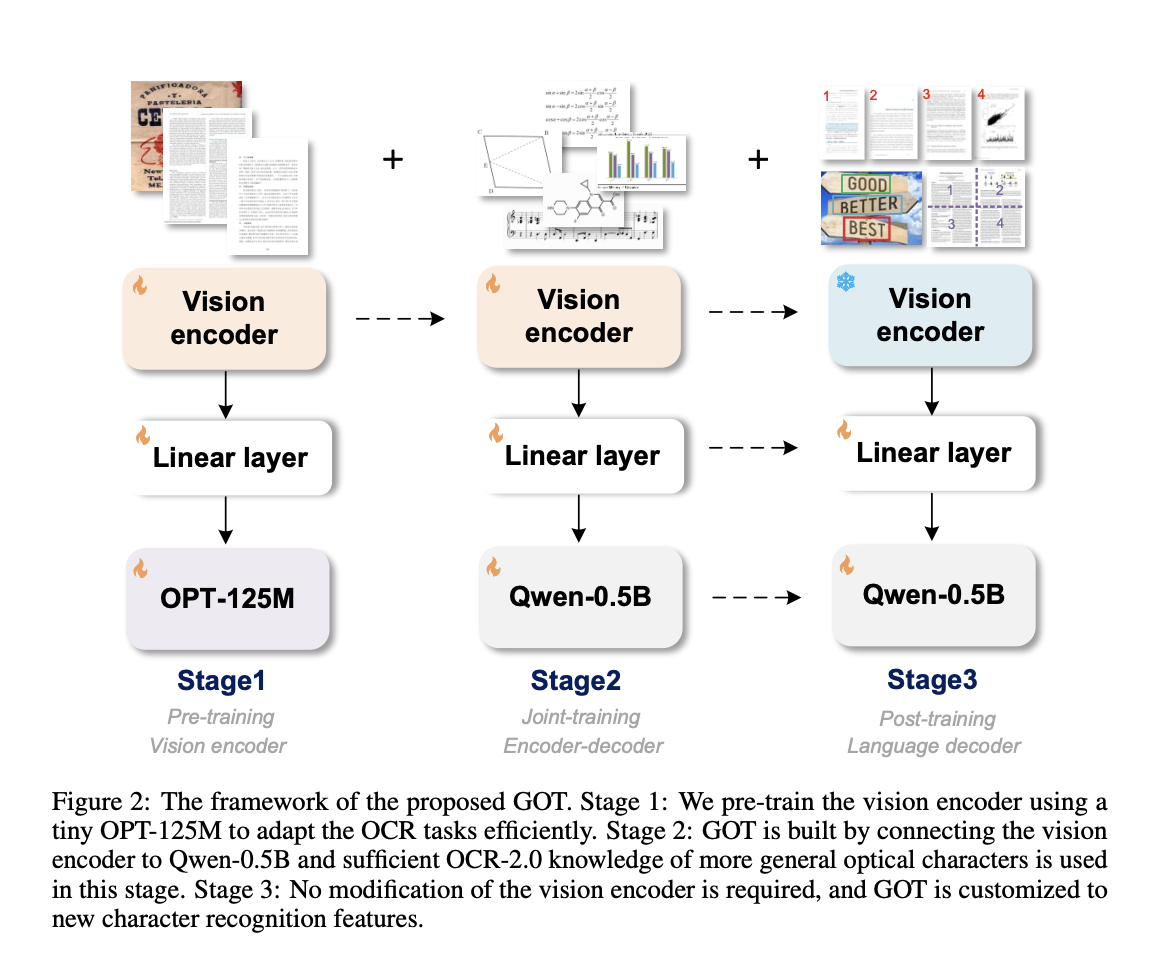
Optical Character Recognition (OCR) Evolution
Challenges of Traditional OCR Systems
Traditional OCR systems, known as OCR-1.0, struggle with versatility and efficiency. They require multiple models for different tasks, leading to complexity and high maintenance costs.
Advances in Large Vision-Language Models (LVLMs)
Recent LVLMs like CLIP and LLaVA have shown impressive text recognition capabilities. However, they are not optimized for OCR-specific functions and require significant computational resources.
The Introduction of GOT Model
Researchers introduced the General OCR Theory (GOT) model as part of OCR-2.0, aiming to provide a unified, end-to-end solution for OCR tasks. GOT can recognize diverse text formats and offers interactive OCR capabilities.
GOT Model Architecture and Performance
The GOT model architecture comprises a high-compression encoder and a long-context decoder with 580 million parameters. It outperforms competing models in various OCR tasks, achieving high accuracy across different languages and complex characters.
Practical Applications and Enhancements
The GOT model incorporates dynamic resolution strategies and multi-page OCR technology, making it practical for real-world applications with high-resolution images or multi-page documents.
Conclusion and AI Solutions
GOT addresses the limitations of traditional OCR-1.0 models and current LVLM-based OCR methods, offering unmatched efficiency and precision. Companies can use AI solutions like GOT to redefine their work processes, identify automation opportunities, and enhance customer engagement.