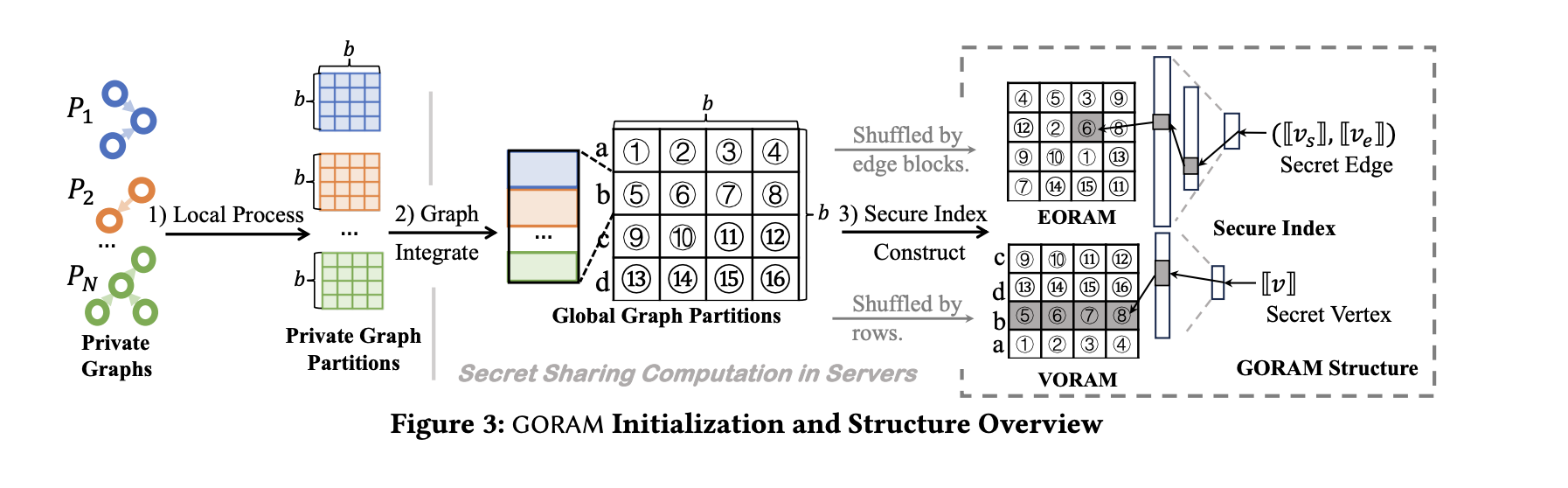
Ego-Centric Searches: Importance and Challenges
Ego-centric searches focus on a single node and its immediate connections. They are crucial for applications like financial fraud detection and social network analysis. However, ensuring privacy while conducting these searches across various data sources is challenging, especially when trust is limited.
Introducing GORAM
GORAM (Graph-Oriented RAM) is a specialized data structure designed to perform ego-centric queries on federated graphs while ensuring strong privacy protection. It uses Secure Multi-Party Computation (MPC), a cryptographic method that allows multiple parties to compute shared data without revealing their individual inputs.
Key Benefits of GORAM:
- Protects sensitive data related to graph vertices and edges.
- Ensures privacy even when data is distributed across potentially untrustworthy sources.
Performance Optimization
While MPC provides strong privacy, it can be slow, especially with large graphs. GORAM addresses this by using an indexing method inspired by Oblivious RAM (ORAM). This method allows access to data without revealing the access pattern, thus enhancing privacy and efficiency.
How GORAM Works:
- Each query targets a specific partition of the graph, reducing processing time.
- Only relevant graph subsets are accessed during queries, preserving privacy.
Proven Performance
Researchers built a prototype querying engine based on GORAM and tested it with five common queries on real-world graphs, including large networks like Twitter. GORAM achieved impressive query times, ranging from 58.1 milliseconds to 35.7 seconds, even for graphs with up to 41.6 million vertices and 1.4 billion edges.
Significant Contributions:
- GORAM enables efficient, sub-linear ego-centric queries with strong privacy protection.
- Optimizations like local processing and lifecycle parallelism enhance performance on large networks.
- The prototype demonstrated scalability and efficiency across various graph types.
Conclusion
GORAM represents a major advancement in conducting effective ego-centric searches on federated graphs while ensuring robust privacy. It balances performance and privacy through MPC and innovative partitioning techniques, making it suitable for large-scale graph processing.
Stay Connected
Check out the Paper for more details. Follow us on Twitter, join our Telegram Channel, and connect with our LinkedIn Group. If you enjoy our work, subscribe to our newsletter and join our 50k+ ML SubReddit.
Upcoming Event
RetrieveX – The GenAI Data Retrieval Conference on Oct 17, 2023.
Transform Your Business with AI
Leverage GORAM to enhance your company’s capabilities. Here are some practical steps:
- Identify Automation Opportunities: Find key customer interactions that can benefit from AI.
- Define KPIs: Ensure measurable impacts from your AI initiatives.
- Select an AI Solution: Choose tools that fit your needs and allow customization.
- Implement Gradually: Start with a pilot project, gather data, and expand wisely.
For AI KPI management advice, contact us at hello@itinai.com. For ongoing insights, follow us on Telegram or Twitter.
Explore AI Solutions
Discover how AI can enhance your sales processes and customer engagement at itinai.com.