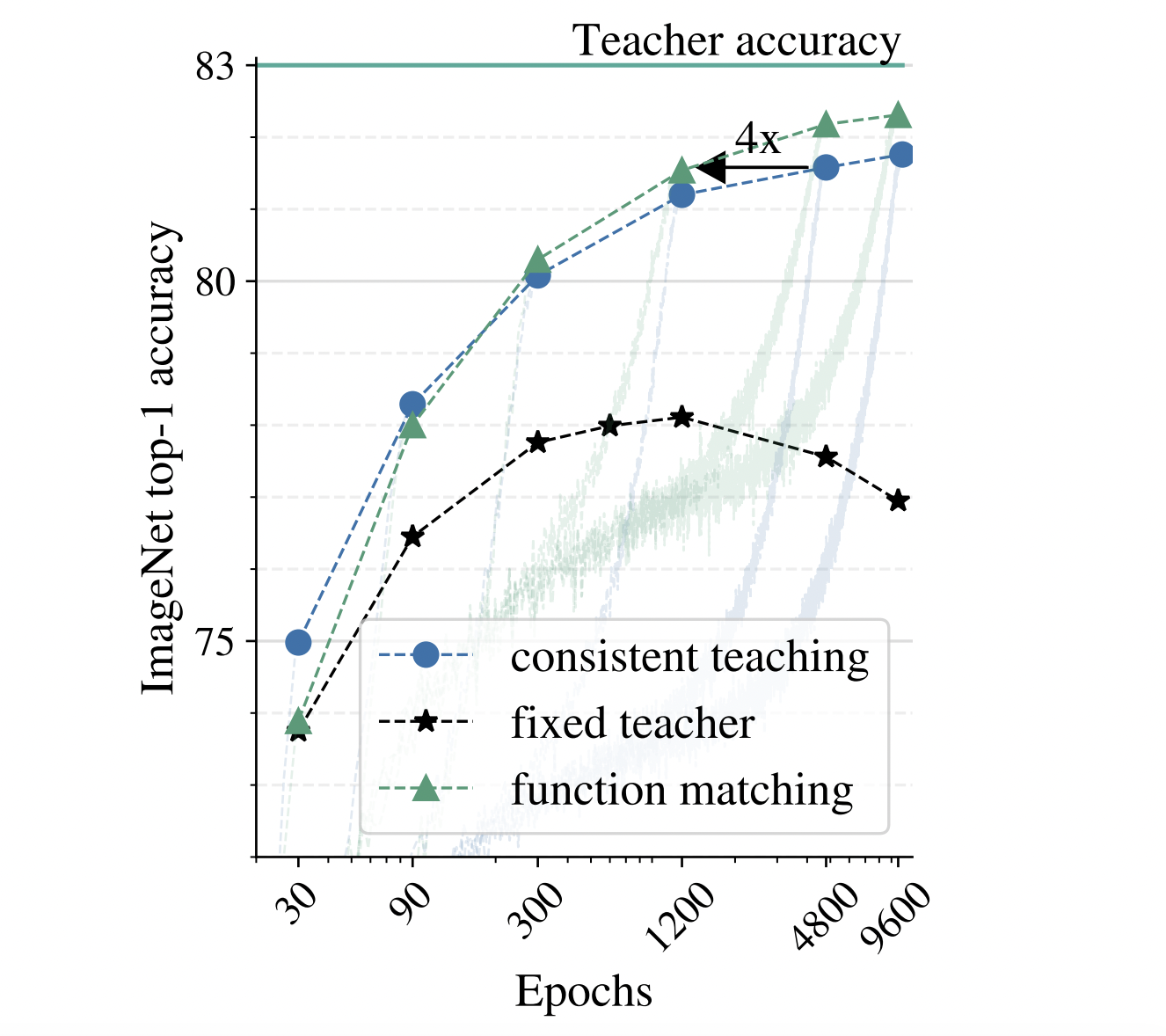
Practical Insights into Knowledge Distillation for Model Compression
Introduction
Many computer vision tasks are dominated by large-scale vision models, which often exceed hardware capabilities. Google Research Team focuses on reducing the computational costs of these models while maintaining performance.
Solution Highlights
- Model pruning and knowledge distillation are employed to reduce the size and improve the efficiency of large models.
- Knowledge distillation involves compressing a large instructor model to a smaller student model by aligning their predictions.
- Aggressive mixup and data augmentation techniques are used to enhance the student model’s generalizability.
Empirical Results
The team successfully compressed the BiT-ResNet-152×2 to a typical ResNet-50 architecture without sacrificing accuracy. The solution achieved an impressive ResNet-50 SOTA of 82.8% on ImageNet, outperforming existing models.
Impact and Future Potential
This study showcases the potential of knowledge distillation for model compression in computer vision, demonstrating the effectiveness and robustness of the proposed distillation formula. It instills optimism about the future of model compression in this field.
For more details, you can check out the full paper here.
AI Solutions for Your Business
Implement AI to stay competitive and redefine your company’s way of work. Identify automation opportunities, define KPIs, select suitable AI solutions, and implement gradually for maximum impact.
For AI KPI management advice and insights into leveraging AI, connect with us at hello@itinai.com or follow our updates on Telegram and Twitter.
Discover how AI can redefine your sales processes and customer engagement. Explore solutions at itinai.com.