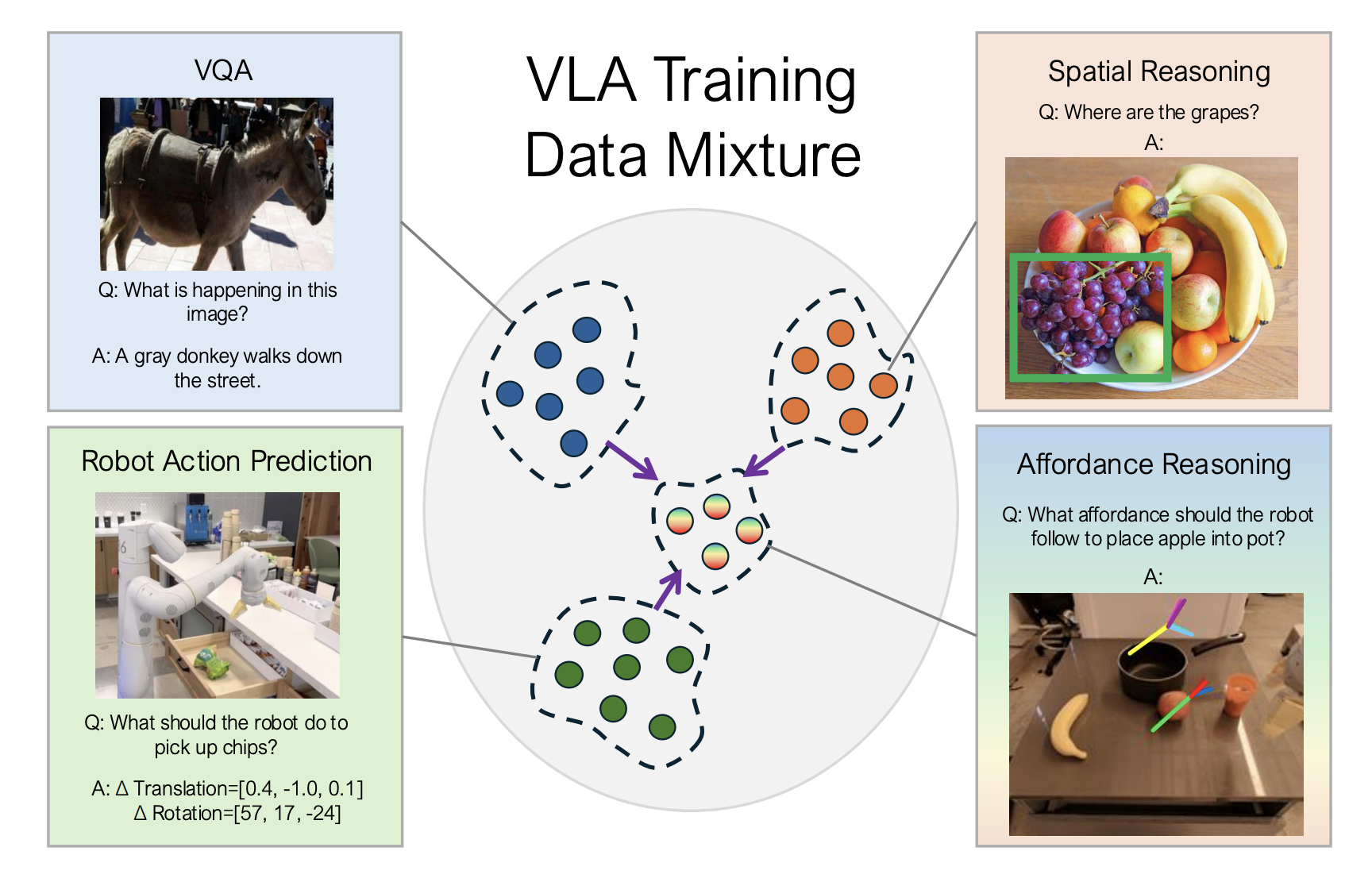
Recent Advances in Robot Policy Representation
Understanding Policy Representation
In recent years, there have been important developments in how robots learn to make decisions. “Policy representation” refers to the different methods robots use to decide what actions to take. This can help robots adapt to new tasks and environments.
Introducing Vision-Language-Action Models
Vision-language-action (VLA) models are trained using extensive robot data. They combine visual perception, language understanding, and action-based decision-making to help robots perform a variety of tasks. While these models show promise in adapting to new situations, they are not yet reliable enough for real-world use outside of controlled lab environments.
Challenges with Current Representations
Current methods like language descriptions, goal images, and trajectory sketches are useful but have limitations.
– **Language-based guidance** often lacks detail, making tasks hard to perform.
– **Goal images** provide spatial information but can be too complex, causing challenges in learning.
– **Trajectory sketches** guide actions but may not give enough details for specific movements.
Introducing RT-Affordance
Researchers from Google DeepMind have developed a new model called RT-Affordance. This hierarchical model creates an “affordance plan” based on task language, which helps guide the robot’s actions.
– **Affordances** refer to how robots can interact with objects based on their design.
– The RT-Affordance model combines information from various data sources, including web datasets and robot trajectories.
How RT-Affordance Works
1. An affordance plan is created using task language and an initial image.
2. This plan is combined with language instructions to guide the robot.
3. The robot uses this plan to understand how to interact with objects effectively.
This method utilizes both extensive web data and robot trajectory data, allowing the model to handle new tasks and environments better.
Experimental Success
The research team conducted experiments focusing on improved robotic grasping, especially with complex items like kettles and pots.
– The RT-Affordance model showed strong performance, achieving success rates between 68%-76% compared to only 24%-28% for previous models.
– In object placing tasks, RT-A achieved a notable 70% success rate.
While the model excels in many areas, its effectiveness slightly declines with entirely new objects.
Conclusion
The RT-Affordance method enhances the robustness and adaptability of robot policies, making it a valuable tool for varied tasks. Although it struggles with completely new actions, it performs better than traditional methods and paves the way for future advancements in robotics.
Stay Connected!
Check out the research paper for more insights. Follow us on Twitter, join our Telegram Channel, and connect with our LinkedIn Group. Don’t forget to subscribe to our newsletter for updates!
Sponsorship Opportunities
Promote your research, product, or webinar to our audience of over 1 million monthly readers and 500,000 community members!
Transform Your Business with AI
Leverage AI to enhance your company’s competitiveness. Key steps include:
– **Identify Automation Opportunities**: Find customer interaction points suitable for AI.
– **Define KPIs**: Measure the impact of AI on business outcomes.
– **Select AI Solutions**: Choose tools tailored to your needs.
– **Implement Gradually**: Start small, gather insights, and expand thoughtfully.
For AI management advice, contact us at hello@itinai.com. Stay updated on leveraging AI by following us on Telegram or Twitter!