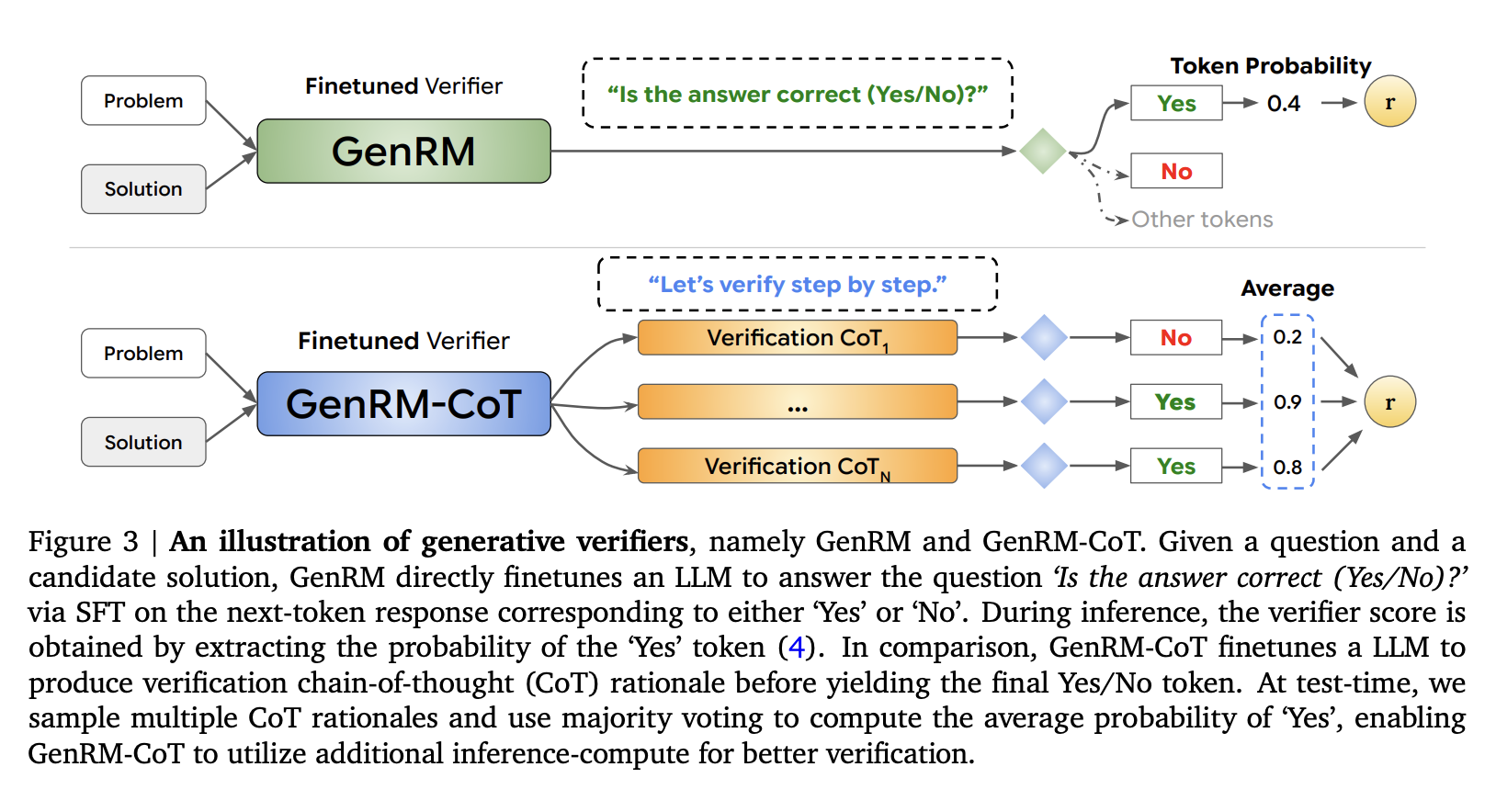
Practical Solutions and Value of Generative AI
Challenges in Generative AI Models
Generative AI models are crucial in various applications, but they often need help with the accuracy and reliability of their outputs. This is particularly problematic in reasoning tasks where a single error can invalidate an entire solution.
Addressing Accuracy and Reliability
Researchers have introduced the Generative Reward Modeling (GenRM) approach to improve the accuracy and reliability of AI-generated solutions. This method redefines the verification process by framing it as a next-token prediction task, integrating the text-generation strengths of large language models (LLMs) into the verification process.
Unified Training Approach
The GenRM methodology employs a unified training approach combining solution generation and verification. It predicts the correctness of a solution through next-token prediction, allowing the model to generate and evaluate potential solutions simultaneously. This approach also supports Chain-of-Thought (CoT) reasoning, enabling more detailed and structured evaluations.
Performance and Scalability
The GenRM model, particularly when paired with CoT reasoning, significantly surpasses traditional verification methods. It has demonstrated a remarkable improvement in accuracy, especially in complex reasoning scenarios. Furthermore, the model scales effectively with increased dataset size and model capacity, enhancing its applicability across various reasoning tasks.
Advancement in Generative AI
The introduction of the GenRM method marks a significant advancement in generative AI, particularly in addressing the verification challenges associated with reasoning tasks. It offers a more reliable and accurate approach to solving complex problems by unifying solution generation and verification into a single process.
AI Application and Evolution
The GenRM approach provides a solid foundation for further research and development in areas where precision and reliability are crucial. It is a valuable tool for future AI applications across multiple domains.