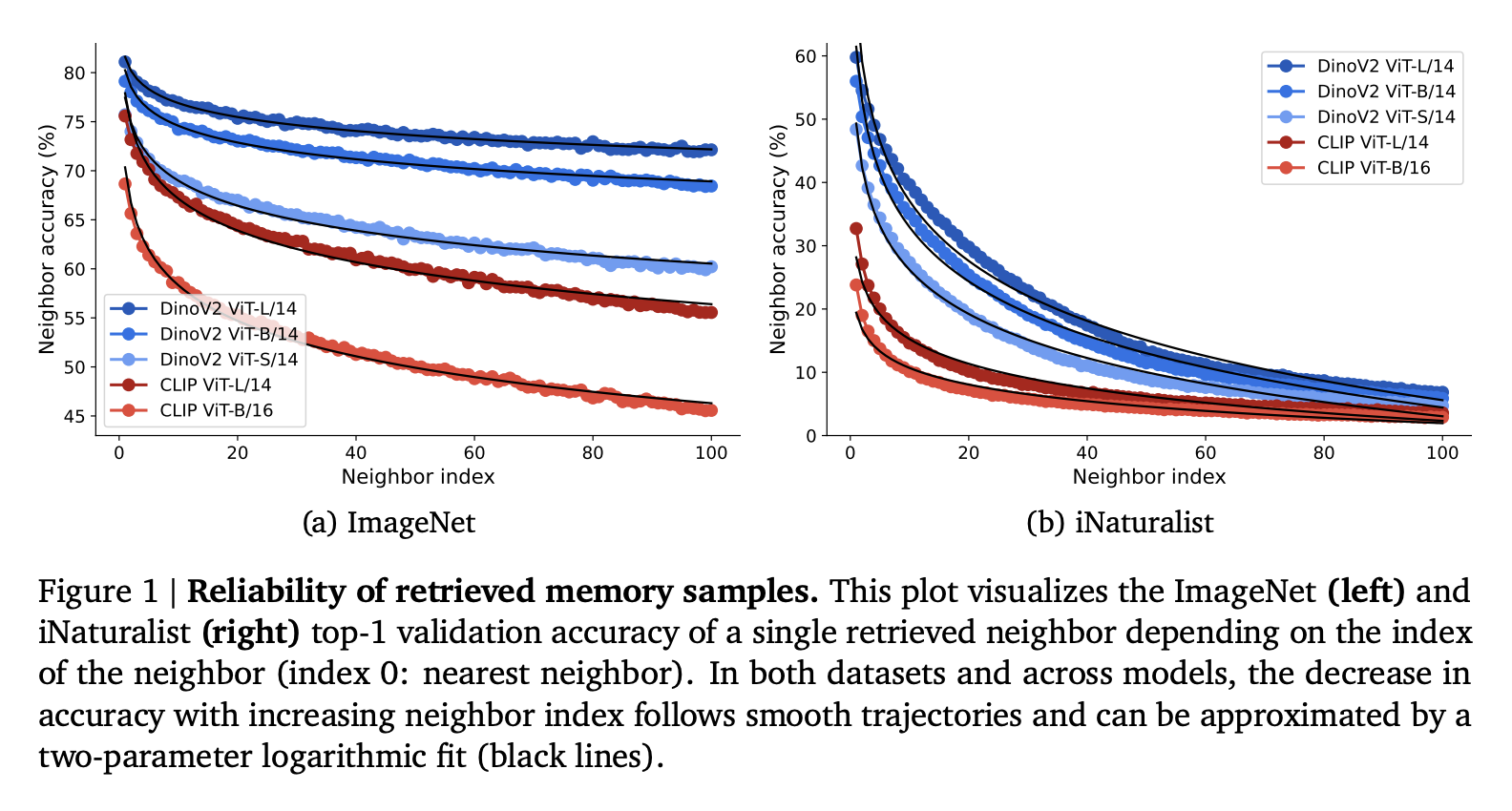
Practical Solutions for Dynamic Image Classification
Integrating Visual Memory for Adaptive Learning
Deep learning models often struggle to adapt to evolving data needs. The proposed solution integrates deep neural networks with a visual memory database, allowing seamless addition and removal of data without frequent retraining.
Retrieval-Based Visual Memory System
The system rapidly classifies images by retrieving the k nearest neighbors based on cosine similarity, enabling the model to adapt to new data without retraining.
RankVoting Aggregation Method
The RankVoting method assigns weights to neighbors based on rank, outperforming traditional techniques and enhancing classification accuracy.
Scalable and Flexible Visual Memory
The visual memory system can scale to billion-scale datasets without additional training, and can remove outdated data through unlearning and memory pruning, making it adaptable for dynamic environments.
Value of Dynamic Visual Memory System
The proposed visual memory not only enhances classification accuracy but also offers a robust framework for integrating new information and maintaining model relevance over time, providing a reliable solution for dynamic learning environments.
Future Applications and Adoption
The research highlights the potential of a flexible visual memory system as a solution to the challenges posed by static deep learning models, offering significant performance improvements and inspiring optimism for future applications.
AI Evolution with Google DeepMind’s Dynamic Visual Memory
Stay competitive and redefine your way of work with the proposed dynamic visual memory for flexible image classification. Discover how AI can redefine your sales processes and customer engagement.
AI Implementation Guidance
Identify automation opportunities, define KPIs, select AI solutions, and implement gradually to leverage the benefits of AI in your business. For AI KPI management advice and continuous insights into leveraging AI, connect with us at hello@itinai.com.