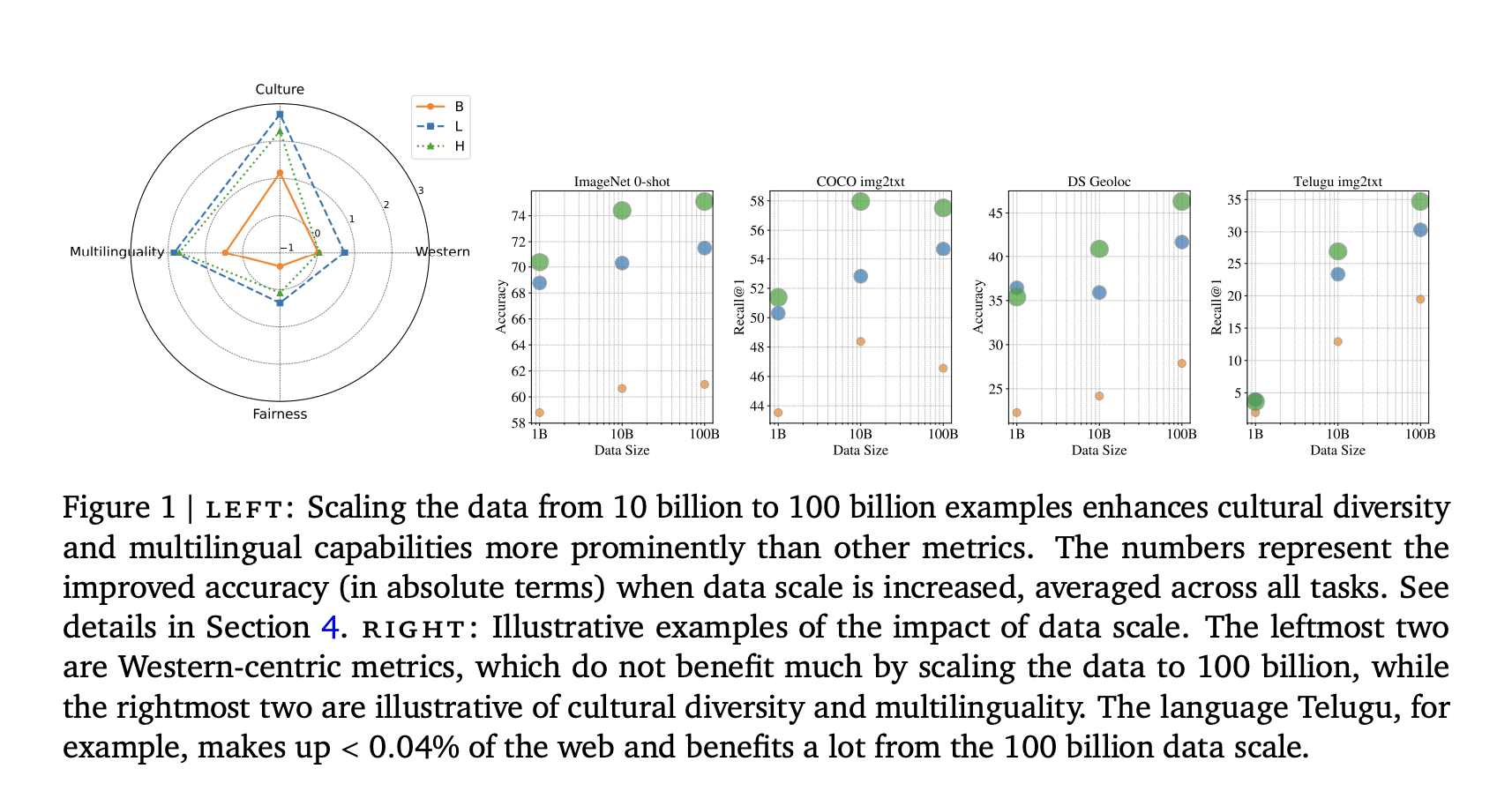
Understanding Vision-Language Models
Machines learn to connect images and text through large datasets. More data helps these models recognize patterns and improve accuracy. Vision-language models (VLMs) use these datasets for tasks like image captioning and answering visual questions. However, the question remains: Does increasing datasets to 100 billion examples significantly enhance accuracy and cultural diversity? As datasets grow beyond 10 billion, the benefits seem to diminish, raising concerns about quality control, bias, and computational limits.
Current Dataset Limitations
At present, VLMs rely on extensive datasets like Conceptual Captions and LAION, containing millions to billions of image-text pairs. While these datasets enable zero-shot classification and image captioning, their growth has plateaued around 10 billion pairs. This limits further improvements in model accuracy and inclusivity. Existing datasets often suffer from low-quality samples and cultural bias, making it hard to enhance multilingual understanding.
Introducing WebLI-100B
To address these challenges, Google DeepMind has developed WebLI-100B, a groundbreaking dataset with 100 billion image-text pairs. This dataset captures rare cultural concepts and enhances performance in low-resource languages. Unlike previous datasets, WebLI-100B focuses on scaling data without excessive filtering, preserving important cultural details. The model training involves various subsets (1B, 10B, and 100B) to evaluate the benefits of data scaling.
Research Findings
Models trained on the full WebLI-100B dataset outperformed those trained on smaller datasets, especially in cultural and multilingual tasks. Researchers created a quality-filtered 5B dataset and a language-rebalanced version to boost low-resource languages. The training used the SigLIP model and evaluated performance across various benchmarks. Results showed that increasing the dataset size improved cultural diversity tasks and low-resource language retrieval, although Western-centric benchmarks saw minimal gains. Bias analysis revealed ongoing gender-related biases despite improvements in diversity.
Conclusion and Future Directions
Scaling vision-language datasets to 100 billion pairs has enhanced inclusivity by improving cultural diversity and multilingual capabilities, while reducing performance gaps across different groups. Although traditional benchmarks showed limited progress, quality filters like CLIP boosted performance on standard tasks at the cost of data diversity. This research can guide future studies in creating filtering algorithms that enhance diversity and promote inclusivity in VLMs.
Leverage AI for Your Business
To evolve your company with AI and stay competitive, consider the following practical steps:
- Identify Automation Opportunities: Find key customer interaction points that can benefit from AI.
- Define KPIs: Ensure your AI initiatives have measurable impacts on business outcomes.
- Select an AI Solution: Choose tools that align with your needs and allow customization.
- Implement Gradually: Start with a pilot project, gather data, and expand AI usage cautiously.
For AI KPI management advice, connect with us at hello@itinai.com. Stay updated on leveraging AI by following us on Telegram or Twitter @itinaicom.
Discover how AI can transform your sales processes and customer engagement at itinai.com.