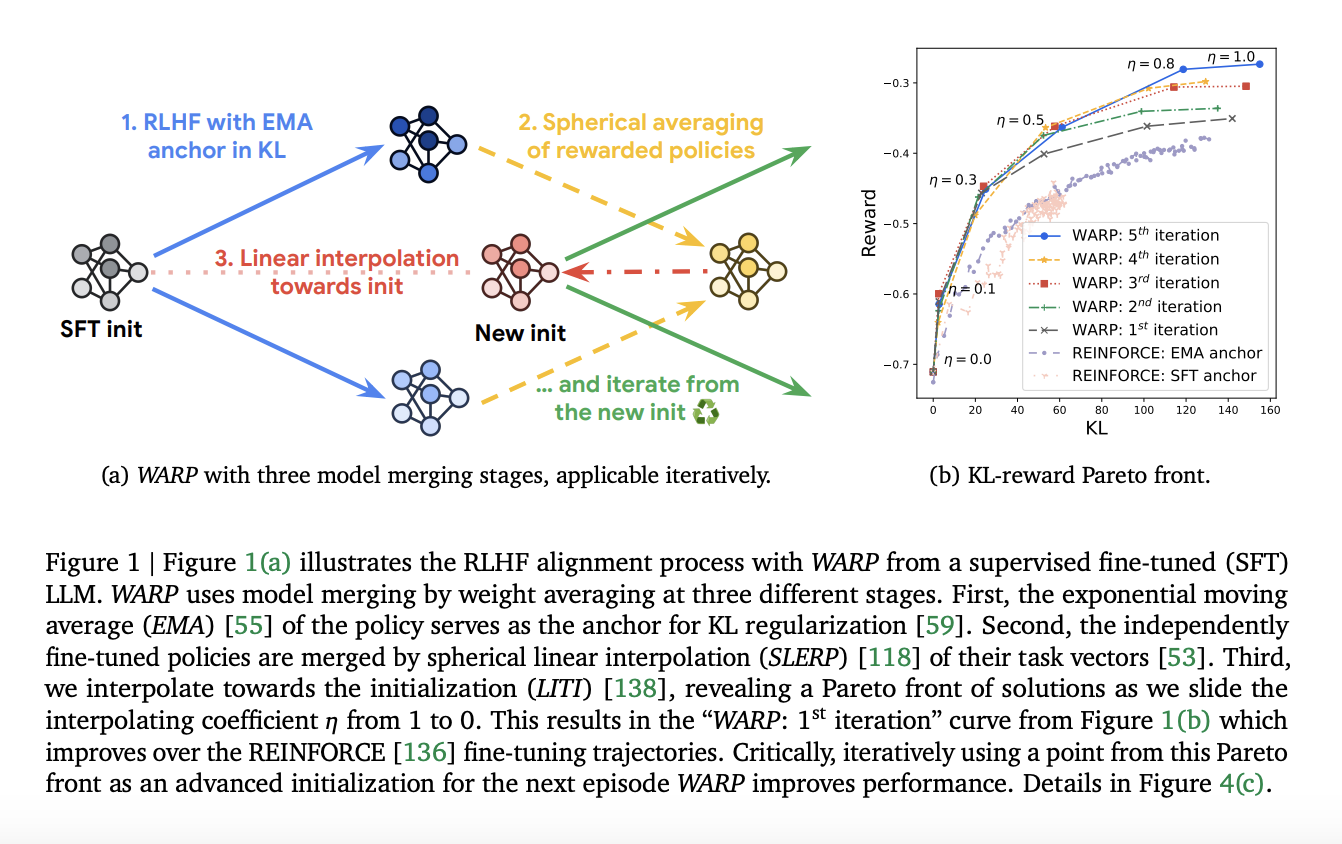
Practical Solutions and Value
Reinforcement Learning from Human Feedback (RLHF) Challenges
RLHF encourages high rewards but faces issues like limited fine-tuning, imperfect reward models, and reduced output variety.
Model Merging and Weight Averaging (WA)
Weight averaging (WA) merges deep models in the weight space to improve generalization, reduce variance, and flatten loss landscape. It also combines strengths in multi-task setups.
Weight Averaged Rewarded Policies (WARP)
Google DeepMind’s WARP aligns large language models (LLMs) and optimizes the KL-reward Pareto front. It uses weight averaging at three stages to enhance rewards and align LLMs while protecting pre-training knowledge.
Experiment Results
WARP outperformed Mistral and Mixtral LLMs, validating its efficiency in improving policies and aligning LLMs.
Future Prospects
WARP could contribute to creating safe and powerful AI systems by improving alignment and encouraging the study of model merging techniques.
Value for Your Company
Discover how AI can redefine your way of work and redefine your sales processes and customer engagement.
AI Solutions for Your Company
Identify Automation Opportunities, Define KPIs, Select an AI Solution, and Implement Gradually.
Connect with Us
For AI KPI management advice, connect with us at hello@itinai.com. For continuous insights into leveraging AI, stay tuned on our Telegram or Twitter.