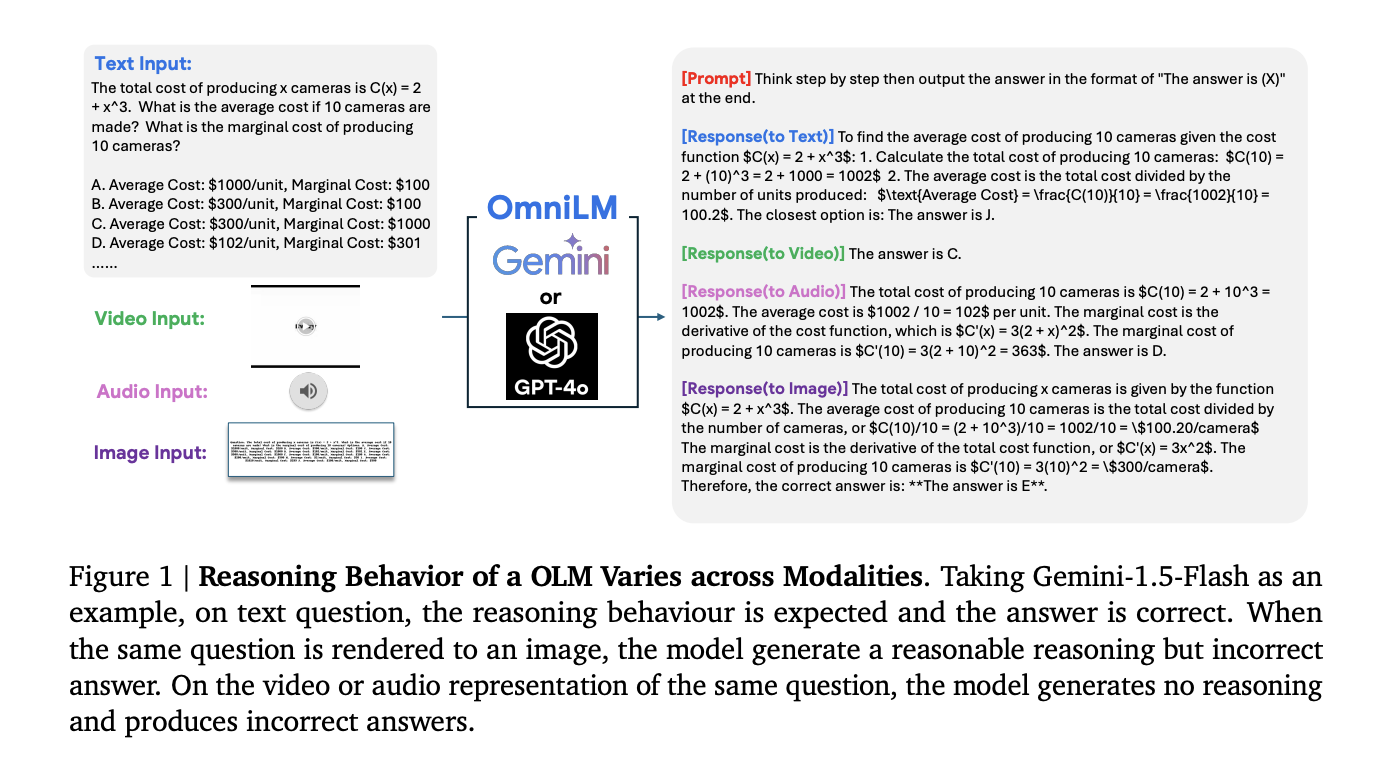
Understanding Omni-Modality Language Models (OLMs)
Omni-modality language models (OLMs) are advanced AI systems that can understand and reason with various types of data, such as text, audio, video, and images. These models aim to mimic human comprehension by processing different inputs at the same time, making them valuable for real-world applications.
The Challenge of Multimodal Inputs
A key challenge for OLMs is their inconsistent performance with multiple data types. For instance, a model may struggle to analyze text, images, and audio together. This inconsistency can lead to different outputs when the same information is presented in various formats.
Limitations of Current Benchmarks
Most existing benchmarks only test simple combinations of two modalities, like text and images. However, real-world tasks often require integrating three or more modalities, which many current models cannot handle effectively.
Introducing Omni×R: A New Evaluation Framework
Researchers from Google DeepMind and the University of Maryland have created Omni×R, a new framework for rigorously evaluating OLMs. This framework presents complex multimodal challenges, requiring models to integrate various data forms to answer questions.
Datasets Used in Omni×R
- Omni×Rsynth: A synthetic dataset that automatically converts text into images, audio, and video, challenging models to process complex inputs.
- Omni×Rreal: A real-world dataset with videos covering topics like math and science, requiring models to combine visual and auditory information.
Key Insights from Research
Experiments with OLMs like Gemini 1.5 Pro and GPT-4o revealed that:
- Models excel with text but struggle with video and audio.
- Performance drops significantly when integrating different modalities.
- Smaller models can outperform larger ones in specific tasks, highlighting a trade-off between model size and flexibility.
Importance of the Findings
The results emphasize the need for further research to improve OLMs’ reasoning capabilities across multiple data types. The synthetic dataset, Omni×Rsynth, is particularly valuable for simulating real-world challenges.
Conclusion
The Omni×R framework represents a significant advancement in evaluating OLMs. By testing models across diverse data types, this research highlights the challenges and opportunities in developing AI systems that can reason like humans.
To stay updated on AI advancements, follow us on Twitter, join our Telegram Channel, and connect with us on LinkedIn. For further insights, subscribe to our newsletter and join our 50k+ ML SubReddit.
Upcoming Webinar
Upcoming Live Webinar- Oct 29, 2024: The Best Platform for Serving Fine-Tuned Models: Predibase Inference Engine.
Transform Your Business with AI
- Identify Automation Opportunities: Find key customer interaction points for AI application.
- Define KPIs: Ensure measurable impacts from your AI initiatives.
- Select an AI Solution: Choose tools that fit your needs and allow for customization.
- Implement Gradually: Start with a pilot project, gather data, and expand your AI usage wisely.
For AI KPI management advice, contact us at hello@itinai.com. For ongoing AI insights, follow us on Telegram at t.me/itinainews or Twitter at @itinaicom.