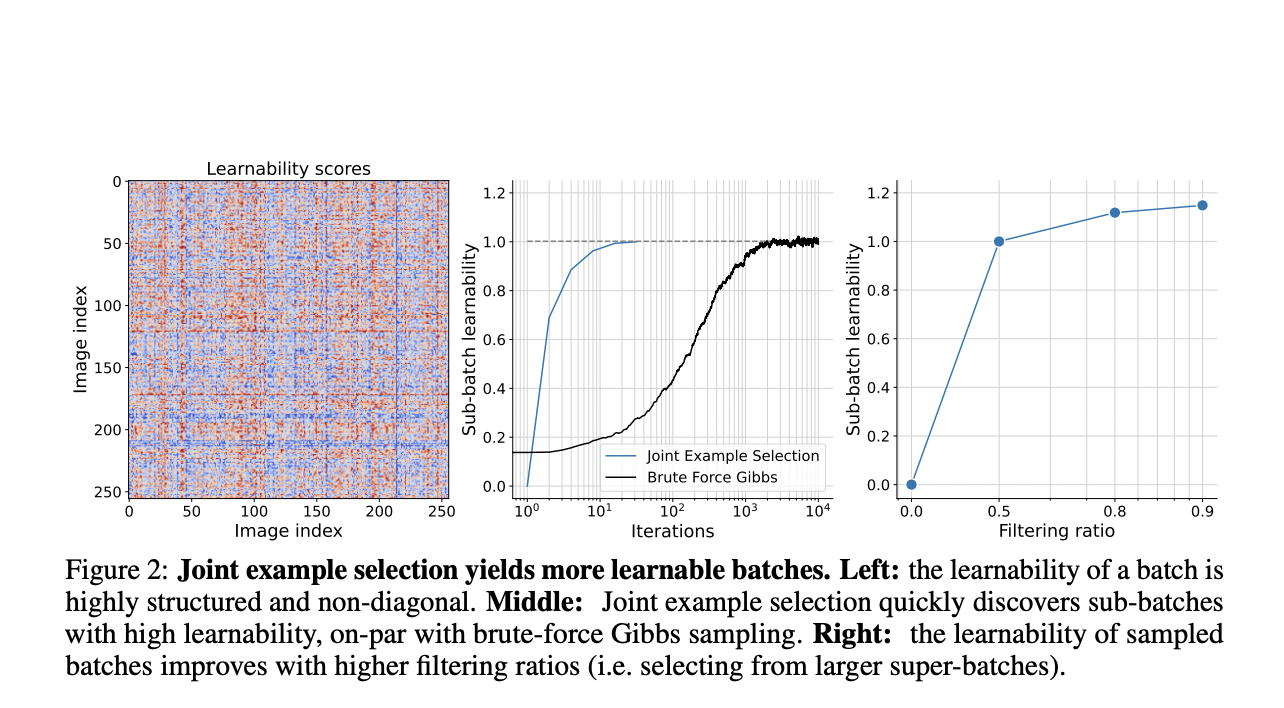
Practical Solutions and Value of JEST AI Training Method
Enhancing Large-Scale Learning with JEST
Data curation is crucial for superior performance in language, vision, and multimodal modeling. Efficient curation with JEST method offers significant improvements in scaling efficiency by selecting high-quality data based on model features.
Accelerated Training and Reduced Computational Overhead
JEST algorithm selects relevant sub-batches from super-batches, accelerating training and reducing computational costs. By leveraging pretrained reference models, it offers improved performance with fewer iterations and less computation.
Improving Model-Based Data Curation
JEST method and its variant, Flexi-JEST, demonstrate the effectiveness of model-based data curation. They outperform traditional methods, thus proving the value of JEST in large-scale learning.
Optimizing Data Selection Efficiency
JEST optimizes the data selection process, improving learnability scoring and increasing batch learnability with few iterations. It significantly accelerates training and improves final performance, showcasing its effectiveness in training and compute efficiency.
Evolve Your Company with AI
Leveraging JEST for Improved Efficiency
Use JEST to stay competitive and enhance AI training efficiency. Identify automation opportunities, define KPIs, select suitable AI solutions, and implement gradual adoption to transform your company’s workflow with AI.
Connect with Us for AI KPI Management
For AI KPI management advice and insights on leveraging AI, connect with us at hello@itinai.com or stay tuned on our Telegram t.me/itinainews or Twitter @itinaicom.
Redefining Sales Processes with AI
Discover how AI can redefine sales processes and customer engagement. Explore AI solutions at itinai.com to optimize your sales and customer interaction using innovative AI technology.