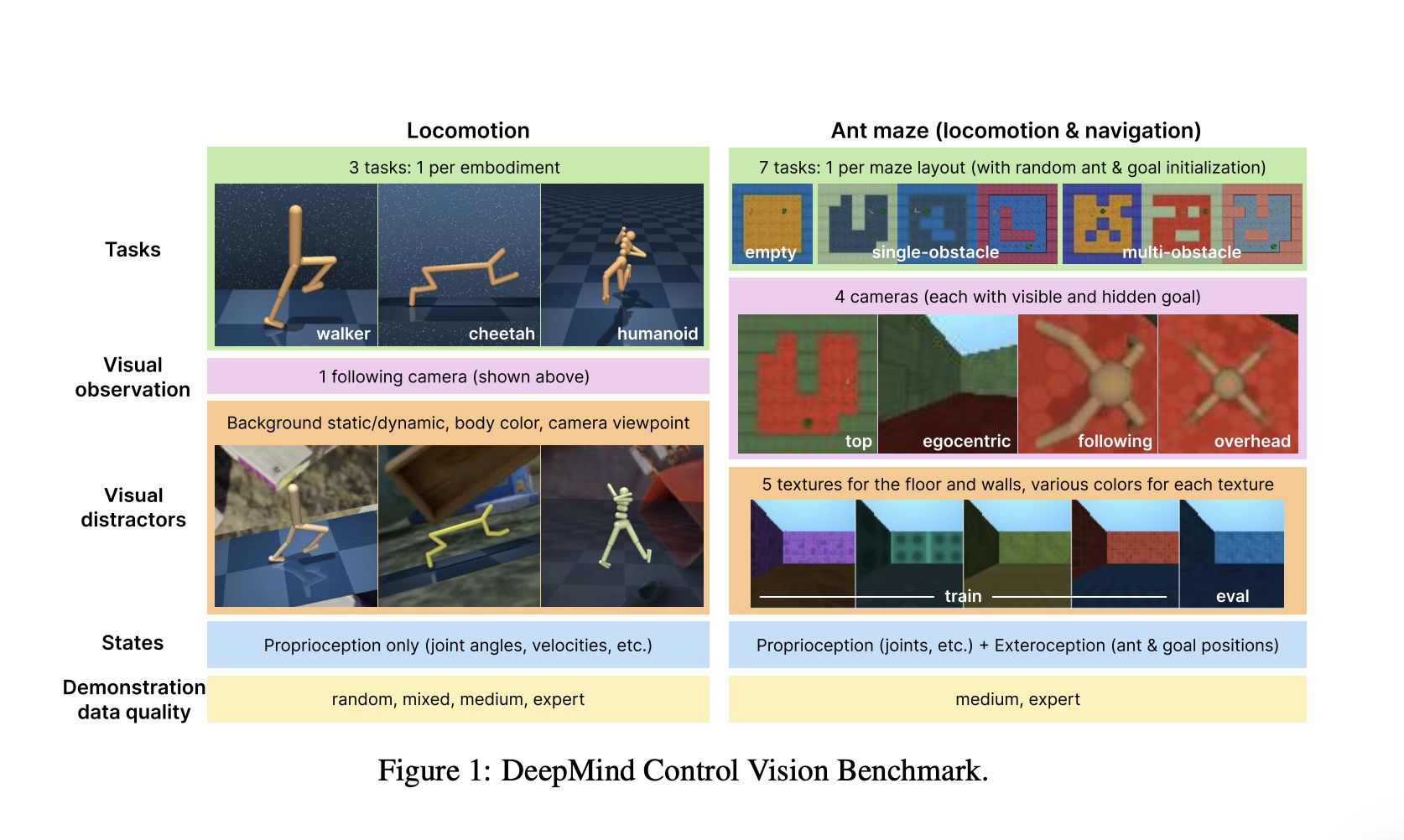
Understanding Reinforcement Learning and Its Challenges
Reinforcement Learning (RL) helps models learn how to make decisions and control actions to maximize rewards in different environments. Traditional online RL methods learn slowly by taking actions, observing outcomes, and updating their strategies based on recent experiences. However, a new approach called offline RL uses large datasets to train models more efficiently. Despite this, models trained on visual data often struggle to adapt to new visual situations, which limits their effectiveness.
Limitations of Current Datasets
Many environments exist to test RL agents, but they often focus on online learning and lack pre-collected data for offline training. Existing datasets do not provide the necessary variety and robustness to thoroughly evaluate how well agents perform under different visual conditions. This gap in data limits our understanding of how well these agents can generalize their learning.
Introducing the DeepMind Control Vision Benchmark (DMC-VB)
To address these challenges, researchers from Google DeepMind have created the DeepMind Control Vision Benchmark (DMC-VB). This dataset is designed to rigorously test offline RL agents in continuous control tasks with various visual distractions. DMC-VB includes:
- Diverse tasks: It features tasks that challenge current algorithms and promote the development of new ones.
- Visual variations: It includes different types of visual distractions, such as changing backgrounds and moving cameras.
- Quality demonstrations: It provides examples of varying quality to see if effective policies can emerge from less-than-perfect demonstrations.
- Comprehensive observations: It offers both pixel data and state measurements, allowing for a better understanding of the representation gap.
- Larger dataset: DMC-VB is more extensive than previous datasets, enhancing its utility.
- Complex tasks: It includes tasks where goals are not visually obvious, highlighting the importance of pretraining representations.
Benchmarks for Evaluation
Alongside the dataset, three benchmarks have been proposed to evaluate representation learning methods:
- Benchmark 1: Assesses how policy learning is affected by visual distractions and measures the representation gap between state-trained and pixel-trained agents.
- Benchmark 2: Explores how agents learn from a mix of high and low-quality data, revealing that pre-training visual representations can enhance learning efficiency.
- Benchmark 3: Investigates how agents adapt to tasks with hidden goals, showing that pre-trained representations facilitate quicker learning.
Future Implications
The DMC-VB dataset and benchmarks provide a solid foundation for advancing research in representation learning for control tasks. They can be expanded to include more complex environments and real-world applications. This research is crucial for improving the generalization of offline RL agents and their performance in various scenarios.
Stay Connected
For more insights and updates, check out the paper and follow us on Twitter, join our Telegram Channel, and connect with our LinkedIn Group. If you appreciate our work, subscribe to our newsletter and join our 50k+ ML SubReddit.
Explore AI Solutions for Your Business
To leverage AI for your company, consider the following steps:
- Identify Automation Opportunities: Find key areas in customer interactions that can benefit from AI.
- Define KPIs: Ensure your AI initiatives have measurable impacts on business outcomes.
- Select an AI Solution: Choose tools that fit your needs and allow for customization.
- Implement Gradually: Start with a pilot project, gather data, and expand AI usage thoughtfully.
For AI KPI management advice, contact us at hello@itinai.com. Stay tuned for continuous insights on leveraging AI through our Telegram and Twitter channels.