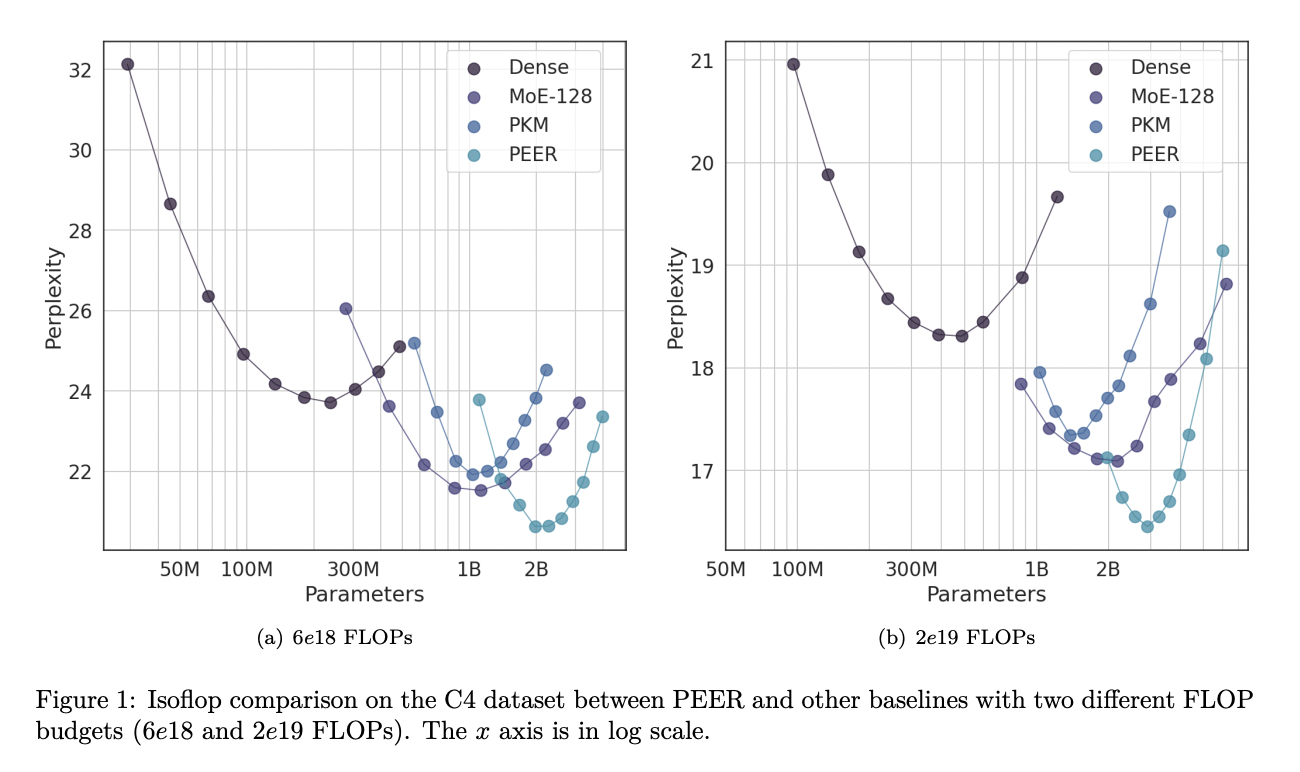
Advancing AI Research with PEER Architecture
Addressing Computational Challenges in Transformer Models
In transformer architectures, the computational costs and activation memory grow linearly with the increase in the hidden layer width of feedforward (FFW) layers. This scaling issue poses a significant challenge, especially as models become larger and more complex.
Practical Solution: PEER leverages a vast pool of tiny experts and efficient routing techniques to overcome this challenge, enabling the deployment of large-scale models in real-world applications.
Value: PEER models demonstrate substantial improvements in efficiency and performance for language modeling tasks.
PEER: A Novel Approach to Model Efficiency
PEER leverages the product key technique for sparse retrieval from a vast pool of tiny experts, numbering over a million. This approach enhances the granularity of MoE models, resulting in a better performance-compute trade-off.
Practical Solution: PEER decouples computational cost from parameter count, representing a significant advancement over previous architectures.
Value: PEER layers significantly outperform dense FFWs and coarse-grained MoEs in terms of performance-compute trade-off.
Superior Performance of PEER Architecture
The PEER model’s superior performance-compute trade-off, demonstrated through extensive experiments, highlights its potential to advance AI research by enabling more efficient and powerful language models.
Practical Solution: PEER can effectively scale to handle extensive and continuous data streams, making it a promising solution for lifelong learning and other demanding AI applications.
Value: PEER models achieved notably lower perplexity scores compared to dense and MoE models, showcasing their efficiency and effectiveness.
For more information, check out the Paper. All credit for this research goes to the researchers of this project.