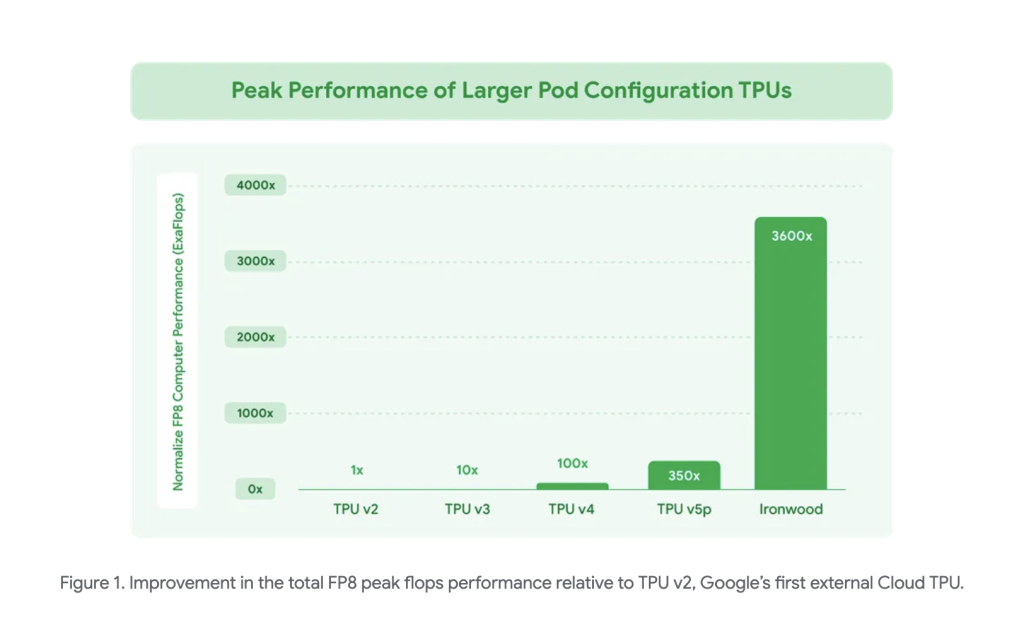
Introducing Ironwood: Google’s New TPU for AI Inference
At the 2025 Google Cloud Next event, Google unveiled Ironwood, the latest generation of its Tensor Processing Units (TPUs). This new chip is specifically designed for large-scale AI inference workloads, indicating a shift in focus from training AI models to deploying them efficiently.
Key Features of Ironwood
Ironwood is the seventh generation in Google’s TPU lineup and boasts significant enhancements:
- Performance: Each chip achieves a peak throughput of 4,614 teraflops (TFLOPs).
- Memory: It includes 192 GB of high-bandwidth memory (HBM), with bandwidths reaching 7.4 terabits per second (Tbps).
- Scalability: Ironwood can be configured with either 256 or 9,216 chips, offering up to 42.5 exaflops of compute power.
Focus on Inference
Unlike its predecessors, which balanced both training and inference, Ironwood is optimized solely for inference. This aligns with a growing industry trend where inference, especially for large language and generative models, has become the primary workload. The design prioritizes low-latency and high-throughput performance, essential for real-time applications.
Innovative Architecture
A notable advancement in Ironwood is the enhanced SparseCore technology, which accelerates sparse operations typical in ranking and retrieval tasks. This optimization minimizes data movement across the chip, leading to improved latency and reduced power consumption for inference-heavy applications.
Energy Efficiency
Ironwood significantly improves energy efficiency, providing over double the performance-per-watt compared to previous models. As businesses scale their AI deployments, managing energy consumption becomes critical for both economic and environmental reasons. Ironwood addresses these challenges effectively.
Integration with Google Cloud
Ironwood is part of Google’s AI Hypercomputer framework, a modular platform that combines high-speed networking, custom silicon, and distributed storage. This integration simplifies the deployment of complex AI models, enabling developers to implement real-time applications with minimal setup.
Competitive Landscape
This launch underscores Google’s commitment to maintaining competitiveness in the AI infrastructure market, where companies like Amazon and Microsoft are also developing proprietary AI accelerators. As custom silicon solutions grow in prominence, traditional reliance on GPUs, particularly from Nvidia, is being challenged.
Meeting Enterprise Needs
Ironwood’s release signifies the evolution of AI infrastructure, where efficiency, reliability, and deployment readiness are now as vital as raw computational power. By concentrating on inference-first design, Google aims to fulfill the evolving requirements of businesses utilizing foundational models for various applications, including search, content generation, and recommendation systems.
Conclusion
In summary, Ironwood marks a significant advancement in TPU design, focusing on the specific needs of inference-heavy workloads. With enhanced compute capabilities, improved efficiency, and tight integration within Google Cloud infrastructure, it positions itself as a crucial component for scalable and responsive AI systems. As AI increasingly becomes operational across various industries, hardware optimized for inference will be essential for cost-effective and effective AI solutions.