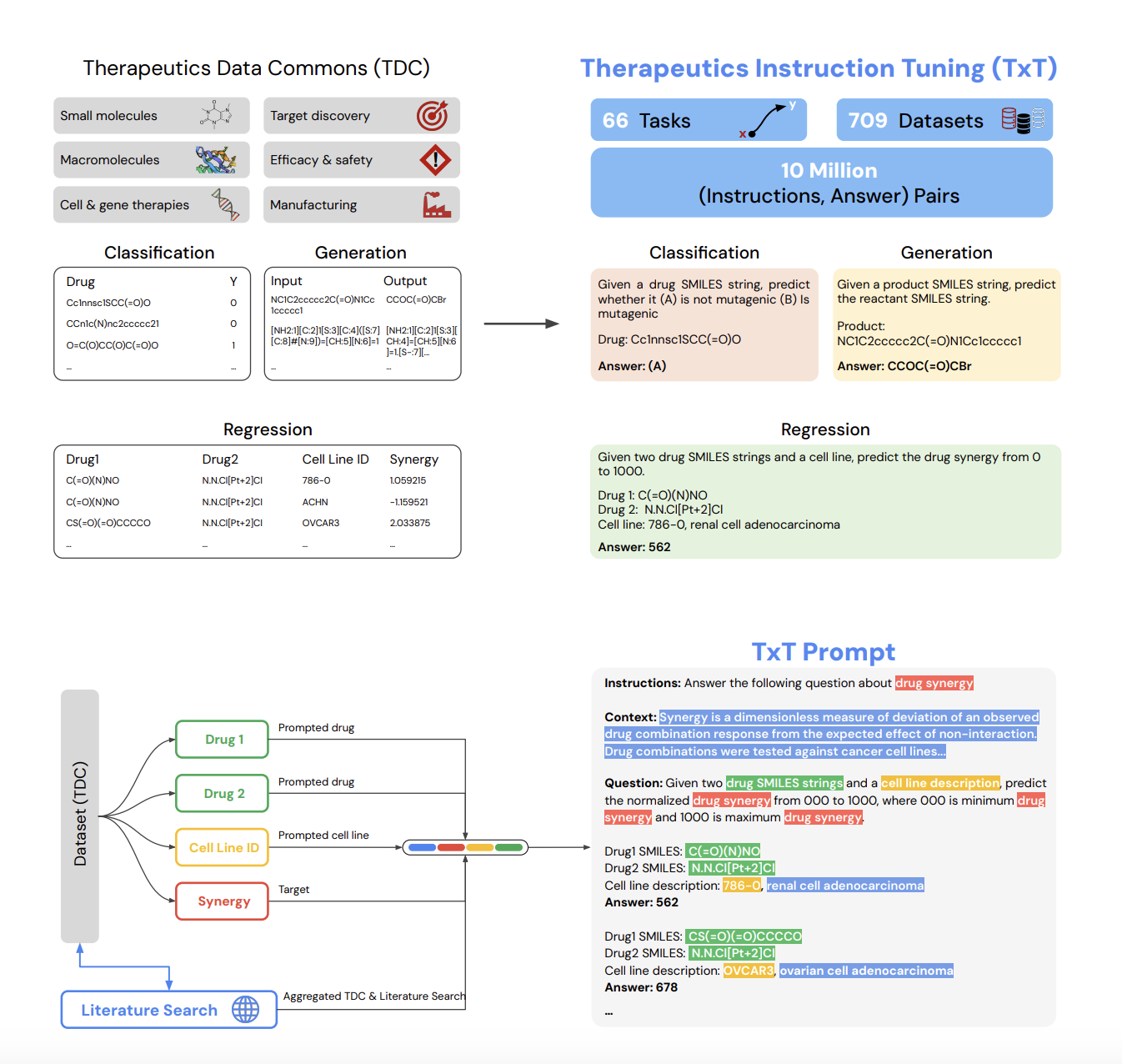
Understanding the Challenges in Therapeutic Development
Creating new drugs is expensive and takes a long time, often requiring 10-15 years and up to $2 billion. Many drug candidates fail during clinical trials. Successful drugs must interact well with targets, be non-toxic, and have good pharmacokinetics.
The Role of AI in Drug Development
Current AI models focus on specific tasks in the drug development process, but their limited scope can reduce effectiveness. The Therapeutics Data Commons (TDC) provides datasets to help AI models predict drug properties, yet these models often work separately. Large Language Models (LLMs) can enhance drug development by learning from various tasks in a unified way.
Advancements in LLMs
LLMs, especially transformer-based models, have improved natural language processing through self-supervised learning on large datasets. They can perform various tasks, including regression, using text-based representations. In drug discovery, specialized models like graph neural networks (GNNs) represent molecules as graphs, while LLMs are increasingly used in biology and chemistry.
Introducing Tx-LLM
Researchers from Google Research and Google DeepMind developed Tx-LLM, a generalist LLM fine-tuned from PaLM-2. This model is designed to handle multiple therapeutic tasks and is trained on 709 datasets covering 66 functions in drug discovery. Tx-LLM uses a single set of weights to process various chemical and biological entities, achieving competitive performance across many tasks.
Dataset Collection and Model Evaluation
The TxT dataset collection includes 709 drug discovery datasets from the TDC, formatted for instruction tuning. The model was evaluated using metrics like AUROC and Spearman correlation to ensure robust results. Tx-LLM showed strong performance, surpassing or matching state-of-the-art results on many tasks.
Key Findings and Future Potential
Tx-LLM is the first LLM trained on diverse TDC datasets, showing improved performance on small molecule tasks when trained with non-small molecule data. While it excels in regression tasks, it still requires improvements in natural language instruction and prediction accuracy for broader applications.
Get Involved and Stay Updated
Check out the research paper for more details. Follow us on Twitter, join our Telegram Channel, and LinkedIn Group for updates. If you enjoy our work, subscribe to our newsletter and join our 50k+ ML SubReddit community.
Transform Your Business with AI
To stay competitive, consider how AI can enhance your operations:
- Identify Automation Opportunities: Find customer interaction points that can benefit from AI.
- Define KPIs: Ensure measurable impacts on business outcomes.
- Select an AI Solution: Choose tools that fit your needs and allow customization.
- Implement Gradually: Start with a pilot project, gather data, and expand AI usage wisely.
For AI KPI management advice, connect with us at hello@itinai.com. For continuous insights, follow us on Telegram or Twitter @itinaicom.
Upcoming Event
RetrieveX – The GenAI Data Retrieval Conference on Oct 17, 2023.