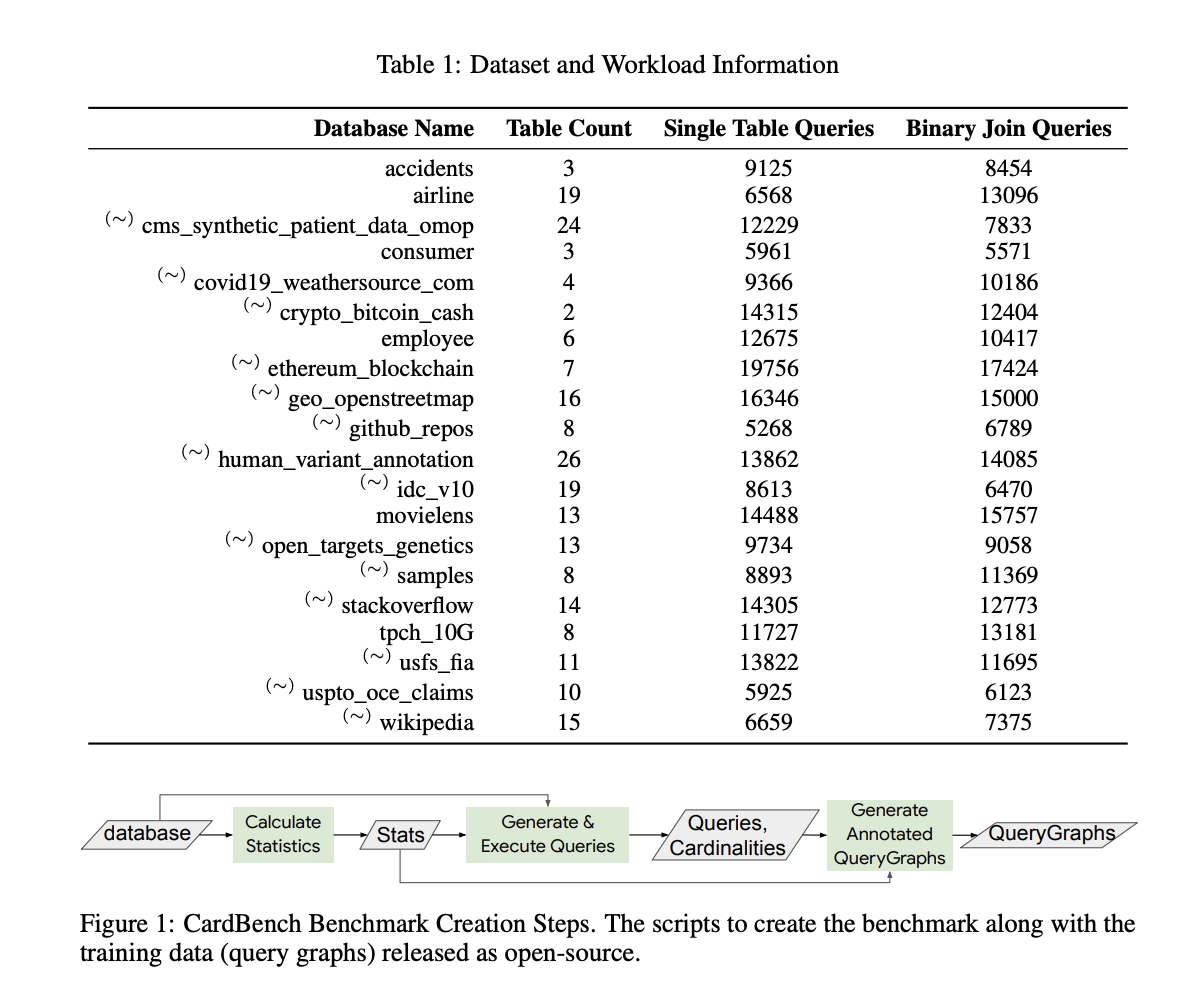
Cardinality Estimation – Driving Database Performance
Practical Solutions for Improved Query Performance
Cardinality estimation (CE) plays a crucial role in optimizing query performance in relational databases. It predicts the number of results a database query will return, influencing execution plans and join methods. Accurate estimates lead to efficient query execution, while inaccurate ones can significantly slow down performance.
Traditional CE techniques rely on heuristics and simplified models, often struggling with complex queries. Learned CE models offer better accuracy but face challenges like high training overheads and data requirements. Google’s CardBench addresses this by providing a comprehensive benchmark with thousands of queries across real-world databases, facilitating the evaluation of learned CE models.
CardBench supports three key setups: instance-based models, zero-shot models, and fine-tuned models, allowing for thorough evaluation under various conditions. It includes tools for generating realistic SQL queries and provides training data for single table and binary join queries, fostering a challenging environment for model evaluation.
Performance evaluations using CardBench demonstrate promising results, especially for fine-tuned models. These models achieve comparable accuracy to instance-based methods with less training data, making them viable for practical applications where training data may be limited.
CardBench represents a significant advancement in learned cardinality estimation, offering a practical solution for evaluating and comparing different CE models. It lowers the barrier for researchers interested in developing and testing new CE models, fostering further innovation in this critical area.
If you want to evolve your company with AI, stay competitive, and use Google AI’s CardBench to revolutionize learned cardinality estimation, connect with us at hello@itinai.com for AI KPI management advice. Follow us on Telegram or Twitter for continuous insights into leveraging AI.