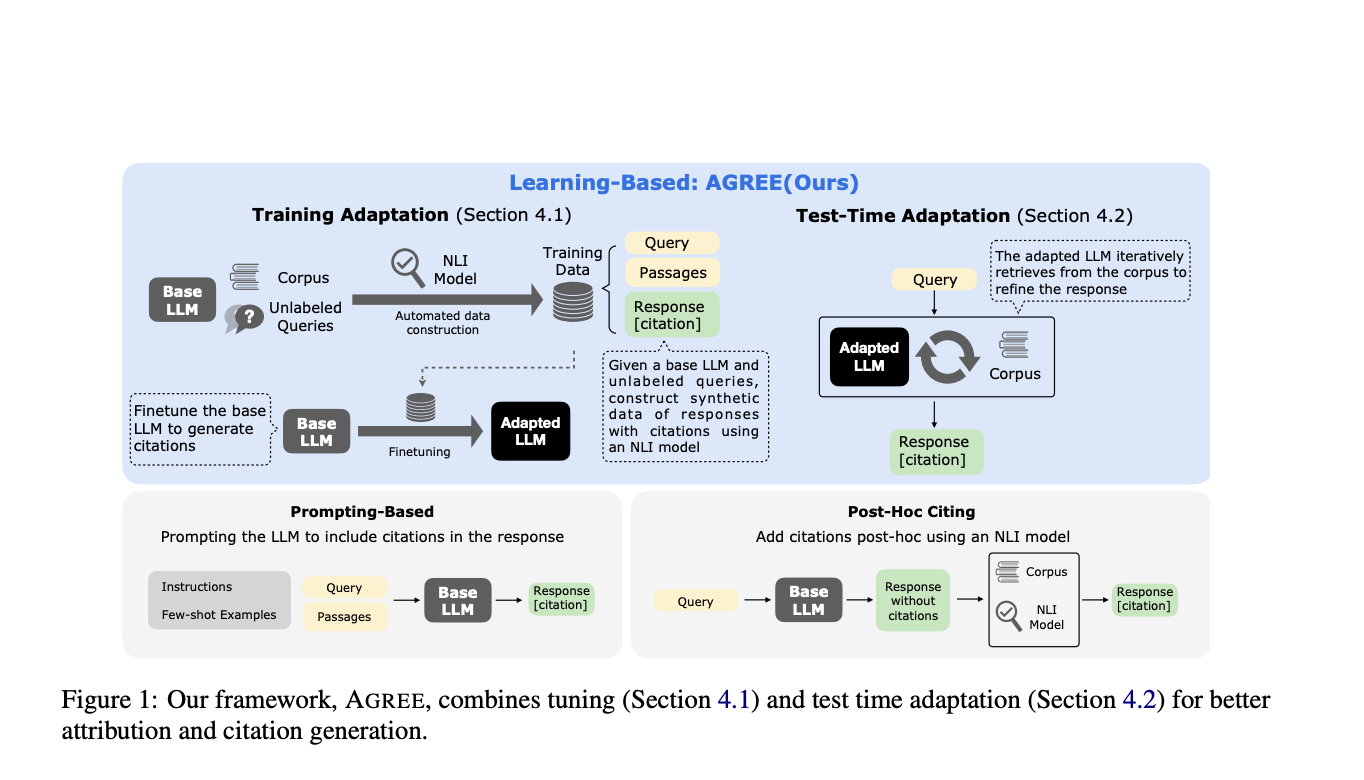
Maintaining Factual Accuracy in Large Language Models (LLMs)
Maintaining the accuracy of Large Language Models (LLMs), such as GPT, is crucial, particularly in cases requiring factual accuracy, like news reporting or educational content creation. LLMs are prone to generating nonfactual information, known as “hallucinations,” when faced with open-ended queries. Google AI Researchers introduced AGREE to address this issue.
Practical Solution: AGREE Framework
AGREE introduces a learning-based framework that enables LLMs to self-ground their responses and provide accurate citations. It combines learning-based adaptation and test-time adaptation to improve grounding and citation precision. AGREE effectively improves the factuality and verifiability of LLMs, enhancing their reliability in domains requiring high factual accuracy.
Value of AGREE
AGREE outperforms current approaches, achieving relative improvements of over 30% in grounding quality. It can work with out-of-domain data, suggesting its robustness across different question types. The inclusion of TTA in AGREE also leads to improvements in both grounding and answer correctness.
AI Implementation Process
1. Identify Automation Opportunities: Locate key customer interaction points that can benefit from AI.
2. Define KPIs: Ensure AI endeavors have measurable impacts on business outcomes.
3. Select an AI Solution: Choose tools that align with your needs and provide customization.
4. Implement Gradually: Start with a pilot, gather data, and expand AI usage judiciously.
AI Sales Bot from itinai.com
Consider the AI Sales Bot from itinai.com/aisalesbot designed to automate customer engagement 24/7 and manage interactions across all customer journey stages.