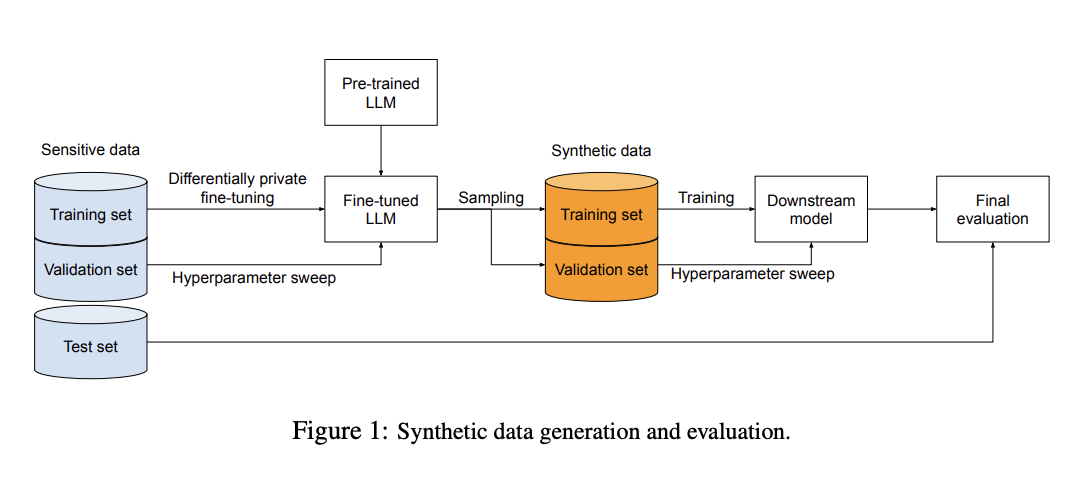
Google AI Described New Machine Learning Methods for Generating Differentially Private Synthetic Data
Practical Solutions and Value
Google AI researchers have developed a novel approach to creating high-quality synthetic datasets that protect user privacy, crucial for training predictive models without compromising sensitive information.
Their method involves leveraging parameter-efficient fine-tuning techniques, such as LoRa and prompt fine-tuning, to generate differentially private synthetic data. This approach reduces computational overhead and potentially improves the quality of the synthetic data.
Empirical results demonstrate that classifiers trained on synthetic data generated by this method outperform those trained on data from other fine-tuning methods, as well as classifiers trained directly on the original sensitive data using differentially private stochastic gradient descent (DP-SGD).
This approach not only preserves privacy but also maintains high utility for training predictive models, making it a valuable tool for organizations looking to leverage sensitive data without compromising user privacy.
Evolve Your Company with AI
Identify Automation Opportunities: Locate key customer interaction points that can benefit from AI.
Define KPIs: Ensure your AI endeavors have measurable impacts on business outcomes.
Select an AI Solution: Choose tools that align with your needs and provide customization.
Implement Gradually: Start with a pilot, gather data, and expand AI usage judiciously.
Spotlight on a Practical AI Solution
Consider the AI Sales Bot from itinai.com/aisalesbot designed to automate customer engagement 24/7 and manage interactions across all customer journey stages.