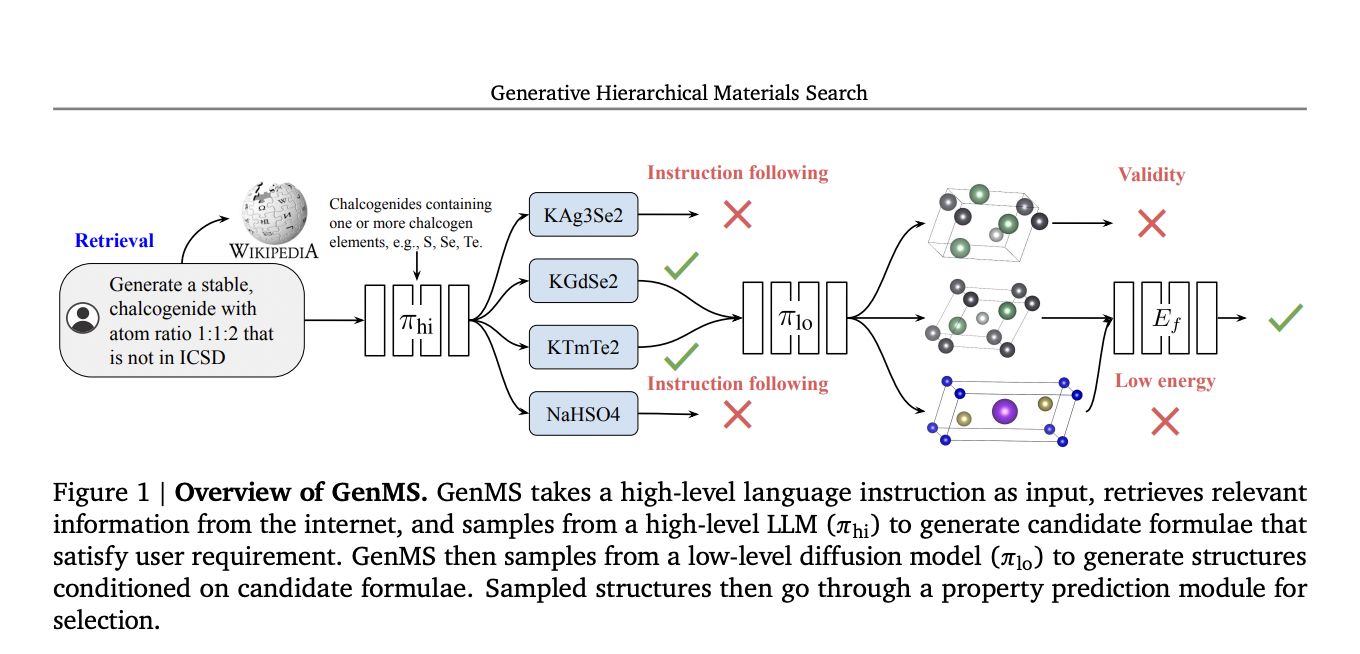
GenMS: An Hierarchical Approach to Generating Crystal Structures from Natural Language Descriptions
Overview
Generative models have progressed considerably, enabling the creation of diverse data types, including crystal structures. In materials science, these models propose new crystals by combining existing knowledge and can handle natural language descriptions to generate crystal structures. The GenMS method by Google DeepMind is a significant advancement in this area, showcasing high success rates in generating complex structures and outperforming traditional methods.
Key Features
GenMS combines a language model, diffusion model, and graph neural network to generate crystal structures from natural language descriptions. It approaches crystal structure generation as a multi-objective optimization problem, ensuring the structures meet user specifications and have low formation energies. The method employs efficient sampling techniques and advanced retrieval methods for precise and effective structure generation.
Performance
GenMS consistently outperforms baseline models in end-to-end tests by producing more valid and unique structures with lower formation energies. It effectively meets specific user requests and enhances formula validity and match rates through advanced retrieval methods. The method lays the groundwork for advanced material design and demonstrates effectiveness with various crystal families.
Implementation Guidance
If you want to evolve your company with AI, consider using GenMS to redefine your way of work. To implement AI solutions effectively, consider identifying automation opportunities, defining KPIs, selecting suitable AI tools, and implementing AI gradually. For AI KPI management advice and insights into leveraging AI, connect with us at hello@itinai.com or stay tuned on our Telegram channel t.me/itinainews or Twitter @itinaicom.