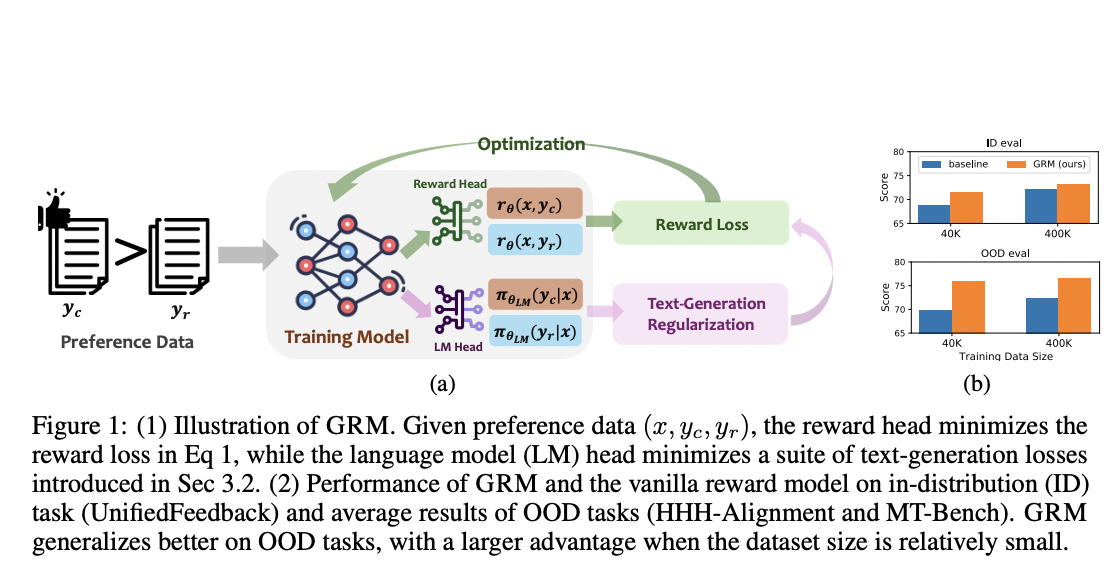
Practical Solutions and Value of Generalizable Reward Model (GRM)
Improving Large Language Models (LLMs) Performance
Pretrained large models can align with human values and avoid harmful behaviors using alignment methods such as supervised fine-tuning (SFT) and reinforcement learning from human feedback (RLHF).
Addressing Overoptimization Challenges
GRM efficiently reduces the overoptimization problem in RLHF, enhancing the accuracy of reward models in various out-of-distribution (OOD) tasks.
Enhancing Generalization Ability
GRM greatly improves the generalization ability of reward models, leading to better performance on both in-distribution (ID) and OOD evaluation sets.
Robustness and Efficiency
GRM is robust against label noise in preference data, showing strong performance even with limited datasets, outperforming baselines with a significant margin.
Conclusion
Generalizable Reward Model (GRM) is an efficient method that aims to improve the generalizability and robustness of reward learning for LLMs. It uses regularization techniques on the hidden states of reward models, significantly improving their generalization performance for unseen data and reducing the problem of overoptimization in RLHF.
AI Solutions for Business
Discover how AI can redefine your way of work by identifying automation opportunities, defining KPIs, selecting AI solutions, and implementing gradually.
Connect with Us
For AI KPI management advice, connect with us at hello@itinai.com. For continuous insights into leveraging AI, stay tuned on our Telegram or Twitter.
Discover AI Solutions for Sales Processes and Customer Engagement
Explore solutions at itinai.com.