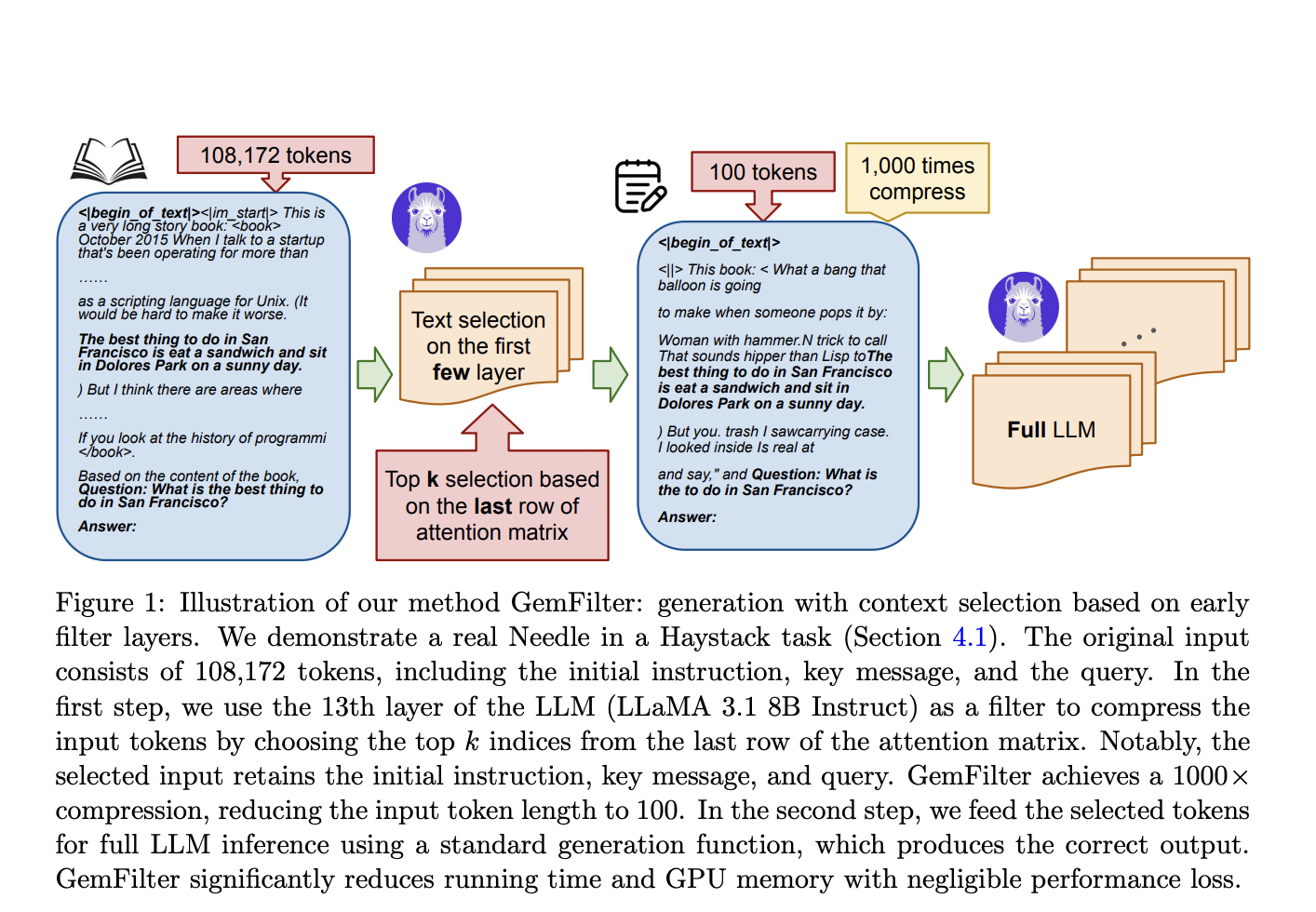
Practical AI Solutions for Optimizing Large Language Models (LLMs)
Challenges in LLM Optimization
Researchers face challenges in accelerating LLM generation speed and reducing GPU memory consumption for long-context inputs.
Existing Techniques
Previous methods focused on KV cache optimization, selective eviction, and dynamic sparse indexing to reduce memory usage and runtime.
GemFilter Approach
GemFilter introduces a two-step process to compress input tokens, leveraging early layer information for efficient token selection.
Results and Performance
GemFilter outperforms existing methods in benchmarks, showcasing significant improvements in efficiency and resource utilization.
Advantages of GemFilter
GemFilter achieves a 2.4× speedup and reduces GPU memory usage, offering simplicity, training-free operation, and broad applicability.
AI Integration and Promotion
Explore how GemFilter can enhance your AI capabilities and drive business evolution by promoting automation opportunities and defining KPIs.
Connect with Us
For AI KPI management advice and insights into leveraging AI, reach out to us at hello@itinai.com or follow us on Telegram @itinainews and Twitter @itinaicom.