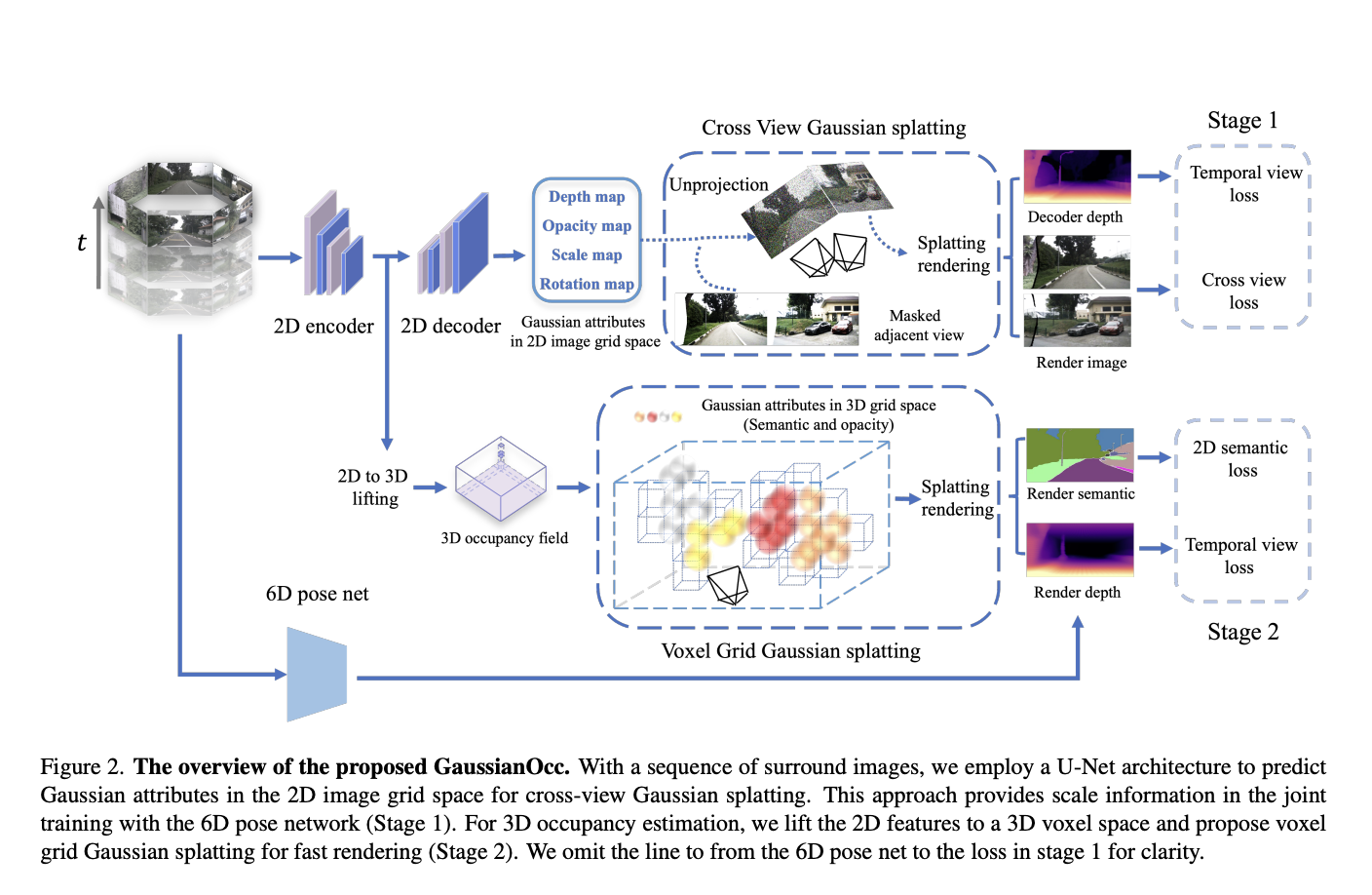
Practical Solutions for 3D Occupancy Estimation
Introducing GaussianOcc: A Self-Supervised Approach
Researchers have developed GaussianOcc, a fully self-supervised approach using Gaussian splatting, to address limitations in existing 3D occupancy estimation methods. This innovative method offers practical solutions to improve efficiency and accuracy in real-world scenarios.
Key Advantages of GaussianOcc
GaussianOcc achieves 2.7 times faster training and 5 times faster rendering compared to traditional methods, demonstrating superior performance in occupancy metrics and depth estimation. The method eliminates the need for ground truth poses during training and enhances rendering efficiency, making it highly suitable for practical applications in 3D occupancy estimation.
Methodology and Innovations
GaussianOcc employs Gaussian Splatting for Projection (GSP) and Gaussian Splatting from Voxel Space (GSV) to optimize model performance, eliminate dependency on external pose data, and enhance rendering efficiency. The method utilizes a U-Net architecture with New-CRFs based on the Swin Transformer for depth estimation and a 6D pose network consistent with SurroundDepth.
Practical Implementation and Impact
GaussianOcc demonstrates strong generalization ability across diverse environments and significantly reduces computational costs. The method’s innovative use of a 6D pose network for self-supervised learning, coupled with its rendering advancements, marks a significant leap forward in 3D scene understanding and reconstruction techniques.
AI Solutions for Business Transformation
For companies looking to evolve with AI, GaussianOcc offers practical advantages in 3D occupancy estimation. To leverage AI effectively, it is essential to identify automation opportunities, define KPIs, select suitable AI solutions, and implement gradually. For AI KPI management advice and insights into leveraging AI, connect with us at hello@itinai.com or stay tuned on our Telegram t.me/itinainews or Twitter @itinaicom.