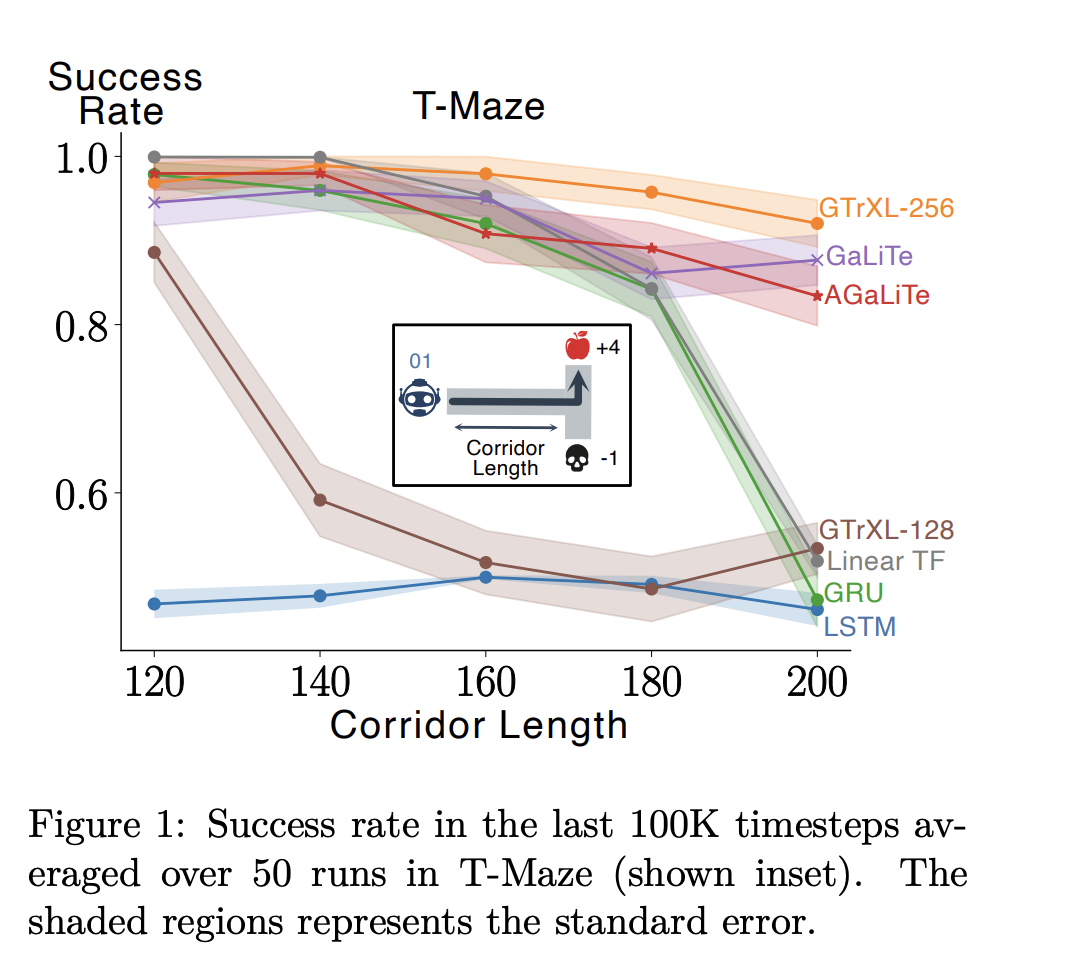
Understanding the Challenges in Decision-Making for Agents
In real-life situations, agents often struggle with limited visibility, making it hard to make decisions. For example, a self-driving car needs to remember road signs to adjust its speed, but storing all observations isn’t practical due to memory limits. Instead, agents must learn to summarize important information efficiently.
Key Solutions for Efficient Learning
To tackle this, incremental state construction is essential in partially observable online reinforcement learning (RL). Recurrent neural networks (RNNs), like LSTMs, can handle sequences well but are challenging to train. Transformers can capture long-term dependencies but require more computational power.
Innovative Approaches to Transformers
Researchers have developed various methods to enhance linear transformers for better handling of sequential data. Some architectures use scalar gating to accumulate information over time, while others introduce recurrence and non-linear updates to improve learning from sequences. Additionally, models that calculate sparse attention or cache previous activations can manage longer sequences without excessive memory use.
New Transformer Architectures
Researchers from the University of Alberta and Amii have created two new transformer models, GaLiTe and AGaLiTe, specifically for partially observable online reinforcement learning. These models reduce the high inference costs and memory demands typical of traditional transformers. They use a gated self-attention mechanism to efficiently manage and update information, leading to improved performance in tasks requiring long-range dependencies.
Performance and Efficiency Gains
Testing in environments like T-Maze and Craftax showed that GaLiTe and AGaLiTe outperformed or matched the best existing models, reducing memory and computation by over 40%. AGaLiTe even achieved up to 37% better performance on complex tasks.
Key Features of GaLiTe and AGaLiTe
GaLiTe enhances linear transformers by introducing a gating mechanism that controls information flow, allowing for selective memory retention. AGaLiTe further improves efficiency by using a low-rank approximation to minimize memory needs, storing recurrent states as vectors instead of matrices.
Evaluating AGaLiTe’s Effectiveness
The AGaLiTe model was tested across various partially observable RL tasks, demonstrating its ability to handle different levels of partial observability. It outperformed traditional models like GTrXL and GRU in both effectiveness and computational efficiency, significantly reducing operations and memory usage.
Conclusion and Future Directions
Transformers are powerful for processing sequential data but face challenges in online reinforcement learning due to high computational demands. The introduction of GaLiTe and AGaLiTe offers efficient alternatives, achieving over 40% lower inference costs and over 50% reduced memory usage. Future research may enhance AGaLiTe with real-time learning updates and applications in model-based RL approaches.
Get Involved and Learn More
Check out the research paper for more details. Follow us on Twitter, join our Telegram Channel, and LinkedIn Group for updates. If you appreciate our work, subscribe to our newsletter and join our 55k+ ML SubReddit community.
Free AI Webinar
Join our upcoming webinar on implementing Intelligent Document Processing with GenAI in Financial Services and Real Estate Transactions.
Transform Your Business with AI
Stay competitive by leveraging GaLiTe and AGaLiTe for your AI needs. Discover how AI can transform your operations:
- Identify Automation Opportunities: Find key customer interaction points that can benefit from AI.
- Define KPIs: Ensure your AI initiatives have measurable impacts on business outcomes.
- Select an AI Solution: Choose tools that fit your needs and allow for customization.
- Implement Gradually: Start with a pilot project, gather data, and expand AI usage wisely.
For AI KPI management advice, contact us at hello@itinai.com. For ongoing insights into leveraging AI, follow us on Telegram at t.me/itinainews or Twitter @itinaicom.
Explore AI Solutions for Sales and Customer Engagement
Discover how AI can enhance your sales processes and customer interactions at itinai.com.