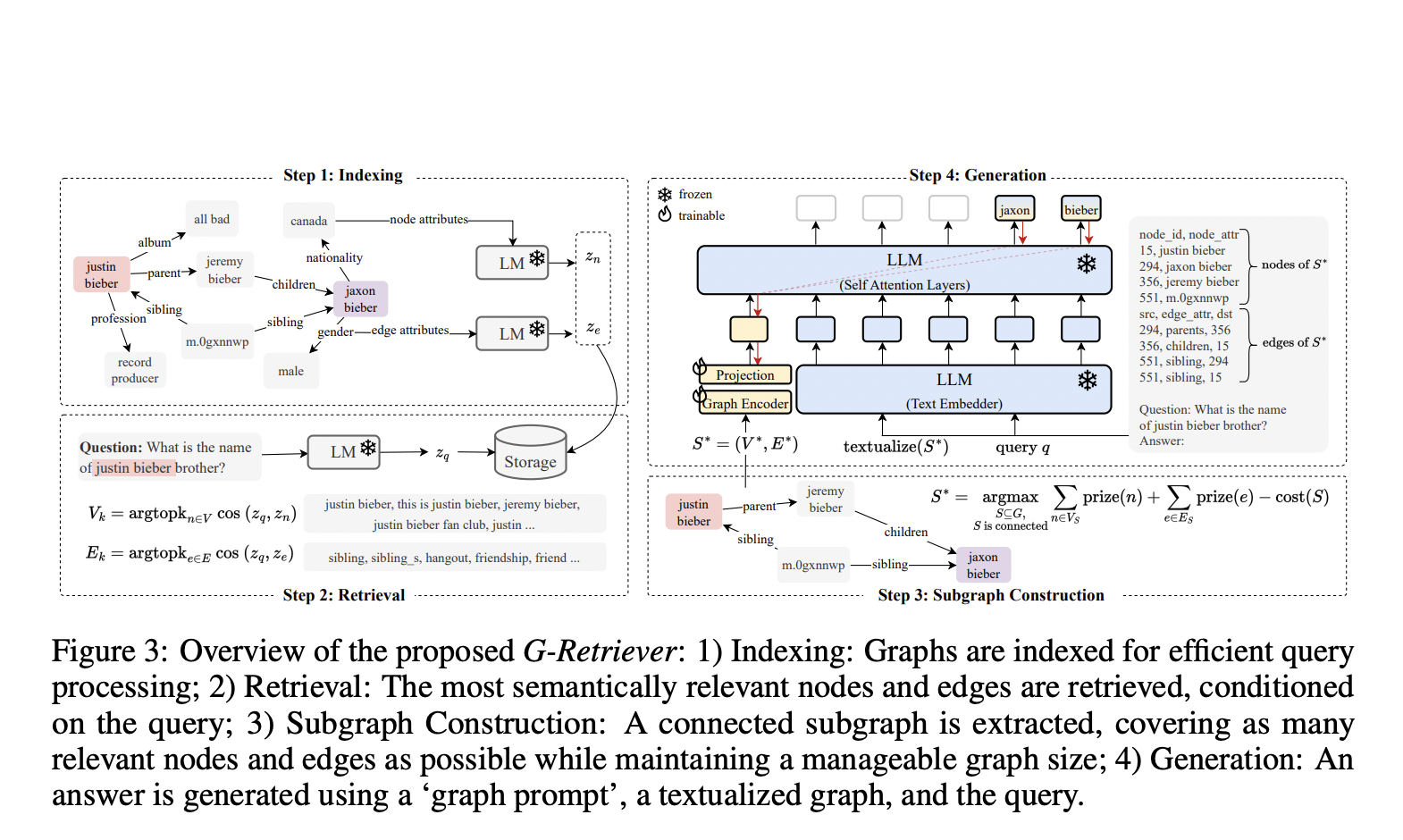
Advancing Real-World Graph Question Answering with G-Retriever
Practical Solutions and Value
Large Language Models (LLMs) have made significant strides in artificial intelligence, but their ability to process complex structured data, particularly graphs, remains challenging. In our interconnected world, a substantial portion of real-world data inherently possesses a graph structure, including the Web, e-commerce systems, and knowledge graphs. Many of these involve textual graphs, making them suitable for LLM-centric methods.
Researchers have developed G-Retriever, an innovative architecture designed for GraphQA, integrating the strengths of Graph Neural Networks (GNNs), LLMs, and Retrieval-augmented generation (RAG). This framework enables efficient fine-tuning while preserving the LLM’s pre-trained language capabilities by freezing the LLM and using a soft prompting approach on the GNN’s output.
G-Retriever demonstrates superior performance across three datasets in various configurations, outperforming baselines in inference-only settings and showing significant improvements with prompt tuning and LoRA fine-tuning. The method greatly enhances efficiency by reducing token and node counts, leading to faster training times. It effectively mitigates hallucination by 54% compared to baselines.
This work introduces a new GraphQA benchmark for real-world graph question answering and presents G-Retriever, an architecture designed for complex graph queries. Unlike previous approaches focusing on conventional graph tasks or simple queries, G-Retriever targets real-world textual graphs across multiple applications. The method implements a RAG approach for general textual graphs, using soft prompting for enhanced graph understanding. G-Retriever employs Prize-Collecting Steiner Tree optimization to perform RAG over graphs, enabling resistance to hallucination and handling of large-scale graphs.
If you want to evolve your company with AI, stay competitive, use for your advantage G-Retriever: Advancing Real-World Graph Question Answering with RAG and LLMs.
AI Solutions for Business
Discover how AI can redefine your way of work. Identify Automation Opportunities: Locate key customer interaction points that can benefit from AI. Define KPIs: Ensure your AI endeavors have measurable impacts on business outcomes. Select an AI Solution: Choose tools that align with your needs and provide customization. Implement Gradually: Start with a pilot, gather data, and expand AI usage judiciously.
For AI KPI management advice, connect with us at hello@itinai.com. And for continuous insights into leveraging AI, stay tuned on our Telegram or Twitter.
Discover how AI can redefine your sales processes and customer engagement. Explore solutions at itinai.com.