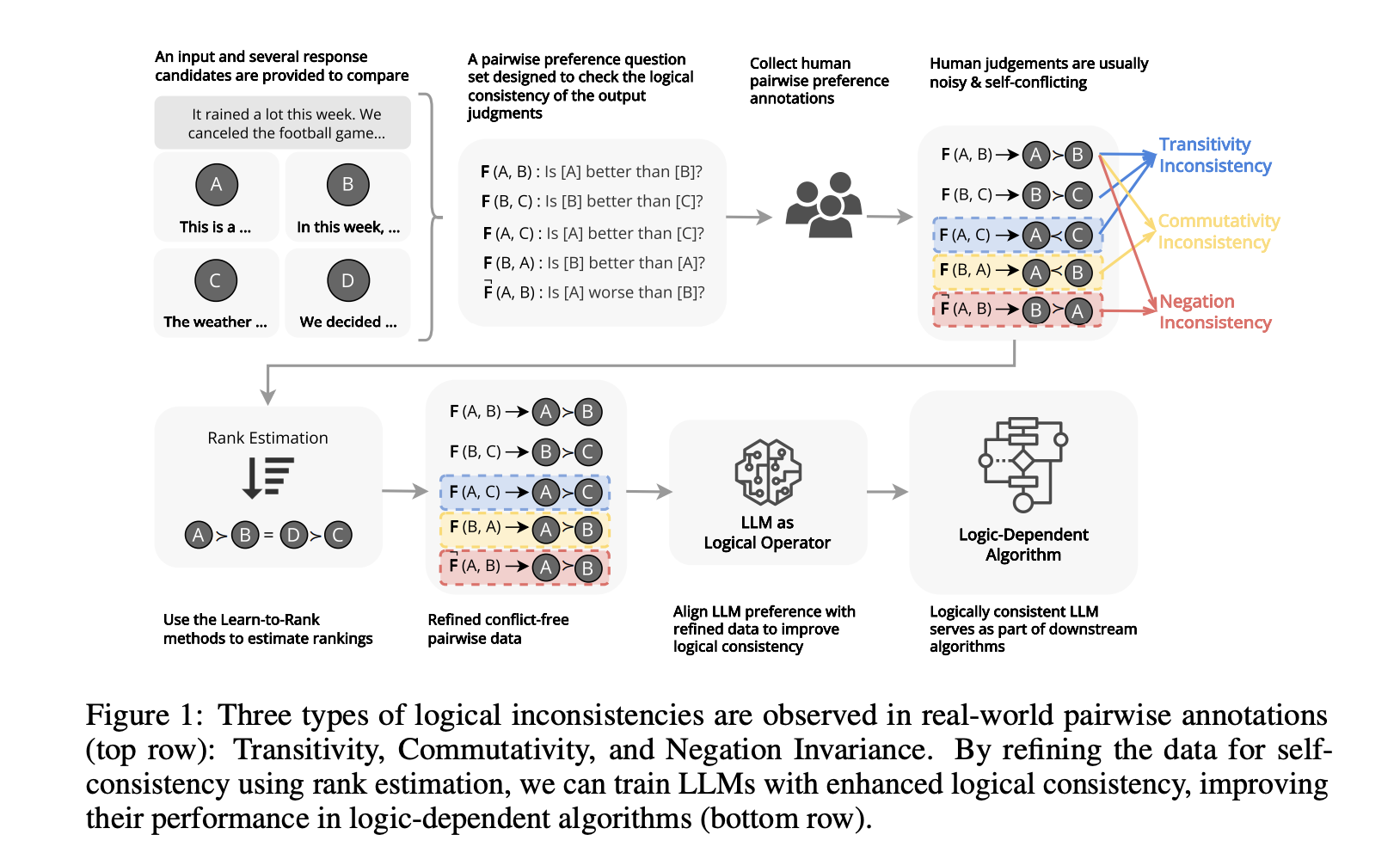
Understanding Large Language Models (LLMs)
Large Language Models (LLMs) are designed to align with human preferences, ensuring they make reliable and trustworthy decisions. However, they can develop biases and logical inconsistencies, which can make them unsuitable for critical tasks that require logical reasoning.
Challenges with Current LLMs
Current methods for training LLMs involve supervised learning and reinforcement learning from human feedback. Unfortunately, these methods often lead to issues like hallucinations and biases, which affect the models’ reliability. Most improvements have focused on simple factual knowledge, leaving gaps in more complex decision-making scenarios.
Evaluating Logical Consistency
Researchers from the University of Cambridge and Monash University have proposed a framework to measure logical consistency in LLMs. They assess three key properties:
- Transitivity: If a model prefers item A over B and B over C, it should also prefer A over C.
- Commutativity: The model’s judgments should remain the same regardless of the order of comparison.
- Negation Invariance: The model should handle negations consistently.
Measuring Consistency
The researchers formalized the evaluation process by treating an LLM as a function that compares items and makes decisions. They used metrics to measure transitivity and commutativity, with scores ranging from 0 to 1—higher scores indicate better performance.
Improving Logical Consistency
To address biases, the researchers introduced a data refinement technique that enhances logical consistency without losing alignment with human preferences. This is crucial for improving the performance of logic-dependent algorithms.
Testing Logical Consistency
They tested LLMs on tasks like summarization and event ordering using various datasets. Results showed that newer models had better logical consistency, although this did not always match human agreement. The findings highlighted the need for cleaner training data to ensure reliable reasoning.
Conclusion
The research emphasizes the importance of logical consistency in enhancing LLM reliability. The proposed framework can guide future research and improve the integration of LLMs into decision-making systems, boosting effectiveness and productivity.
Get Involved
Check out the research paper for more insights. Follow us on Twitter, join our Telegram Channel, and connect with our LinkedIn Group. Don’t miss our 60k+ ML SubReddit community.
Join Our Webinar
Gain actionable insights into improving LLM performance while ensuring data privacy.
Transform Your Business with AI
Stay competitive by leveraging AI solutions:
- Identify Automation Opportunities: Find customer interaction points that can benefit from AI.
- Define KPIs: Ensure measurable impacts on business outcomes.
- Select an AI Solution: Choose tools that fit your needs and allow customization.
- Implement Gradually: Start with a pilot project, gather data, and expand wisely.
Contact Us
For AI KPI management advice, reach out at hello@itinai.com. Stay updated on AI insights via our Telegram or Twitter.
Explore AI Solutions
Discover how AI can enhance your sales processes and customer engagement at itinai.com.