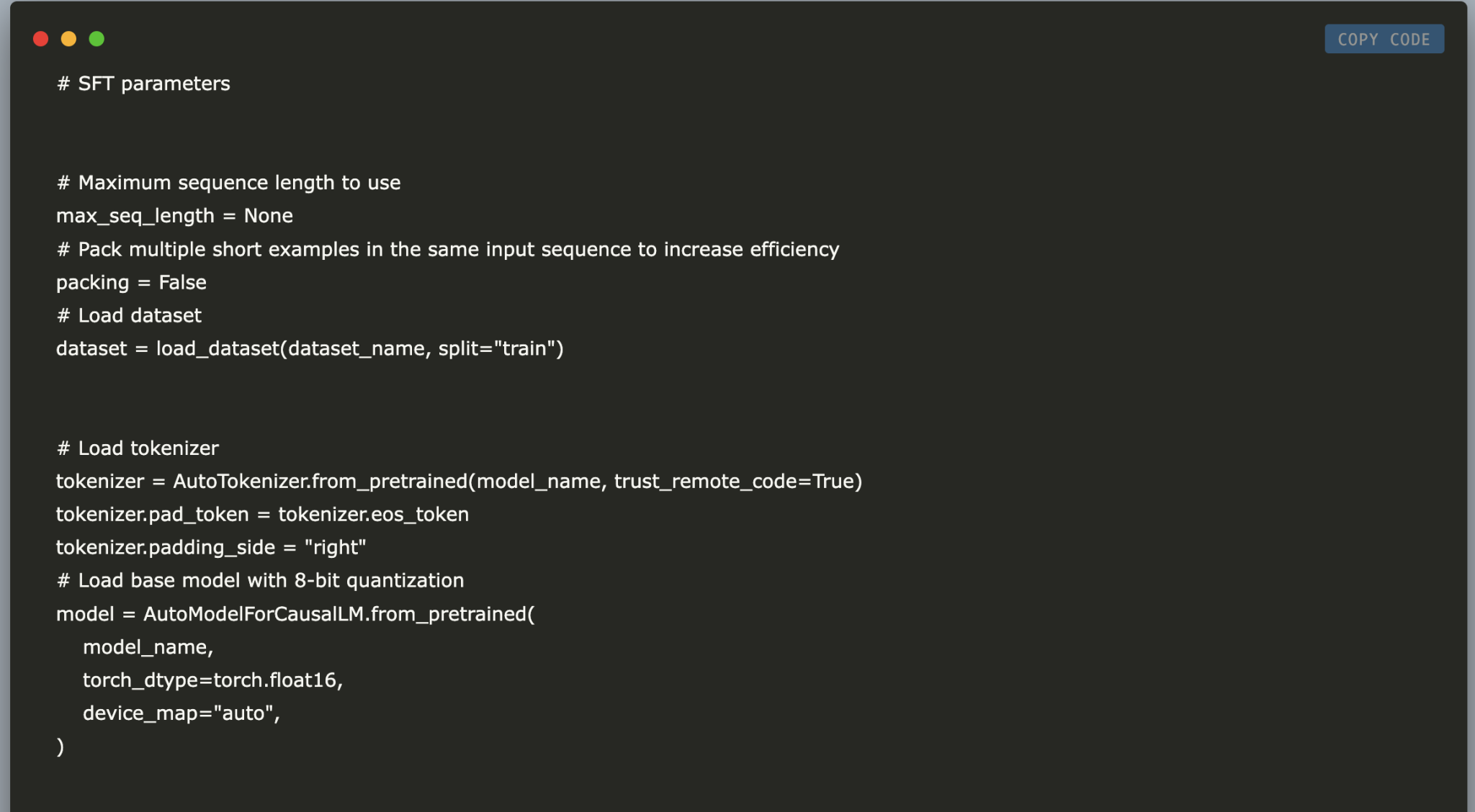
Fine-Tuning Llama-2 7B Chat for Python Code Generation
Overview
In this tutorial, we will show you how to fine-tune the Llama-2 7B Chat model for generating Python code. We will use techniques like **QLoRA**, **gradient checkpointing**, and **supervised fine-tuning** with the **SFTTrainer**. By utilizing the **Alpaca-14k dataset**, you’ll learn to set up your environment and optimize memory for effective training.
Practical Steps and Solutions
1. **Install Required Libraries**: Start by installing essential libraries like **accelerate**, **peft**, **transformers**, and **trl** to ensure your project has the necessary tools.
2. **Model and Dataset Setup**: Define the base model from Hugging Face and specify your dataset. This sets the groundwork for your fine-tuning process.
3. **Configure LoRA Parameters**: Set parameters for **LoRA** (Low-Rank Adaptation) to enhance model efficiency. This includes adjusting attention dimensions and dropout rates.
4. **Training Configuration**: Establish training parameters such as:
– Output directory for model checkpoints
– Number of training epochs
– Batch sizes for training and evaluation
– Learning rate and optimization settings
– Enable gradient checkpointing to save memory
5. **Model Preparation**: Load your dataset and tokenizer. Prepare the model for training by enabling gradient checkpointing, which helps in managing resources effectively.
6. **Apply PEFT**: Use the **get_peft_model** function to apply parameter-efficient fine-tuning to your model.
7. **Training the Model**: Start the training process with the **SFTTrainer** and save your fine-tuned model for future use.
8. **Text Generation**: Create a text generation pipeline to test your fine-tuned model. Input a prompt and generate a response to see how well it performs.
9. **Manage Resources**: After training, clear up GPU memory by deleting unnecessary variables and using garbage collection to optimize performance.
10. **Check GPU Availability**: Verify how many GPUs are available for your tasks, which helps in understanding resource allocation.
Conclusion
By following this tutorial, you have effectively fine-tuned the Llama-2 7B Chat model for Python code generation. This approach demonstrates how to perform fine-tuning while managing resources efficiently, allowing for high performance without extensive computational requirements.
Next Steps
If you’re looking to leverage AI for your business, consider the following:
– **Identify Automation Opportunities**: Pinpoint areas in customer interactions that can benefit from AI.
– **Define KPIs**: Measure the impact of your AI initiatives on business outcomes.
– **Select the Right AI Solution**: Choose tools that meet your needs and allow customization.
– **Implement Gradually**: Begin with pilot projects, collect data, and expand usage based on insights.
For additional guidance or insights, feel free to reach out to us at hello@itinai.com or follow our updates on Telegram and @itinaicom.