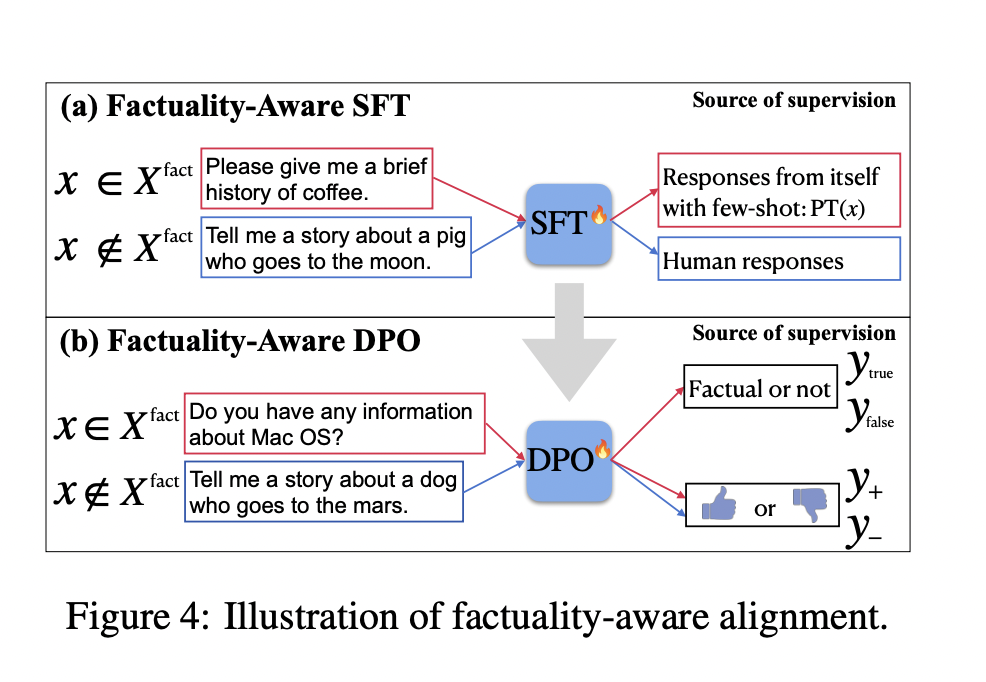
Improving Large Language Models with FLAME
Large Language Models (LLMs) offer robust natural language understanding and generation capabilities for various tasks, from virtual assistants to data analysis. However, they often struggle with factual accuracy, producing misleading information.
Challenges and Solutions
LLMs tend to generate fabricated or incorrect information, known as hallucinations, due to their training processes. Traditional methods prioritize detailed responses, leading to increased hallucinations. Factuality-Aware Alignment (FLAME) addresses this challenge by combining factuality-aware training and optimizing reward functions to improve factual accuracy without compromising instruction-following abilities.
Research Findings
FLAME significantly improved factual accuracy (+5.6-point FActScore) without sacrificing instruction-following capabilities. It was validated using Alpaca Eval and the Biography dataset, demonstrating its effectiveness in balancing factuality and instruction-following.
Practical Applications
FLAME provides a promising solution for enhancing the reliability of LLMs, making them better suited for applications where accuracy is crucial.
AI Optimization Strategies
Discover how AI can redefine your company’s operations by leveraging Factuality-Aware Alignment for reliable and accurate responses. To evolve your company with AI:
- Identify Automation Opportunities
- Define KPIs
- Select an AI Solution
- Implement Gradually
Practical AI Solutions from itinai.com
Explore the AI Sales Bot designed to automate customer engagement 24/7 and manage interactions across all customer journey stages.