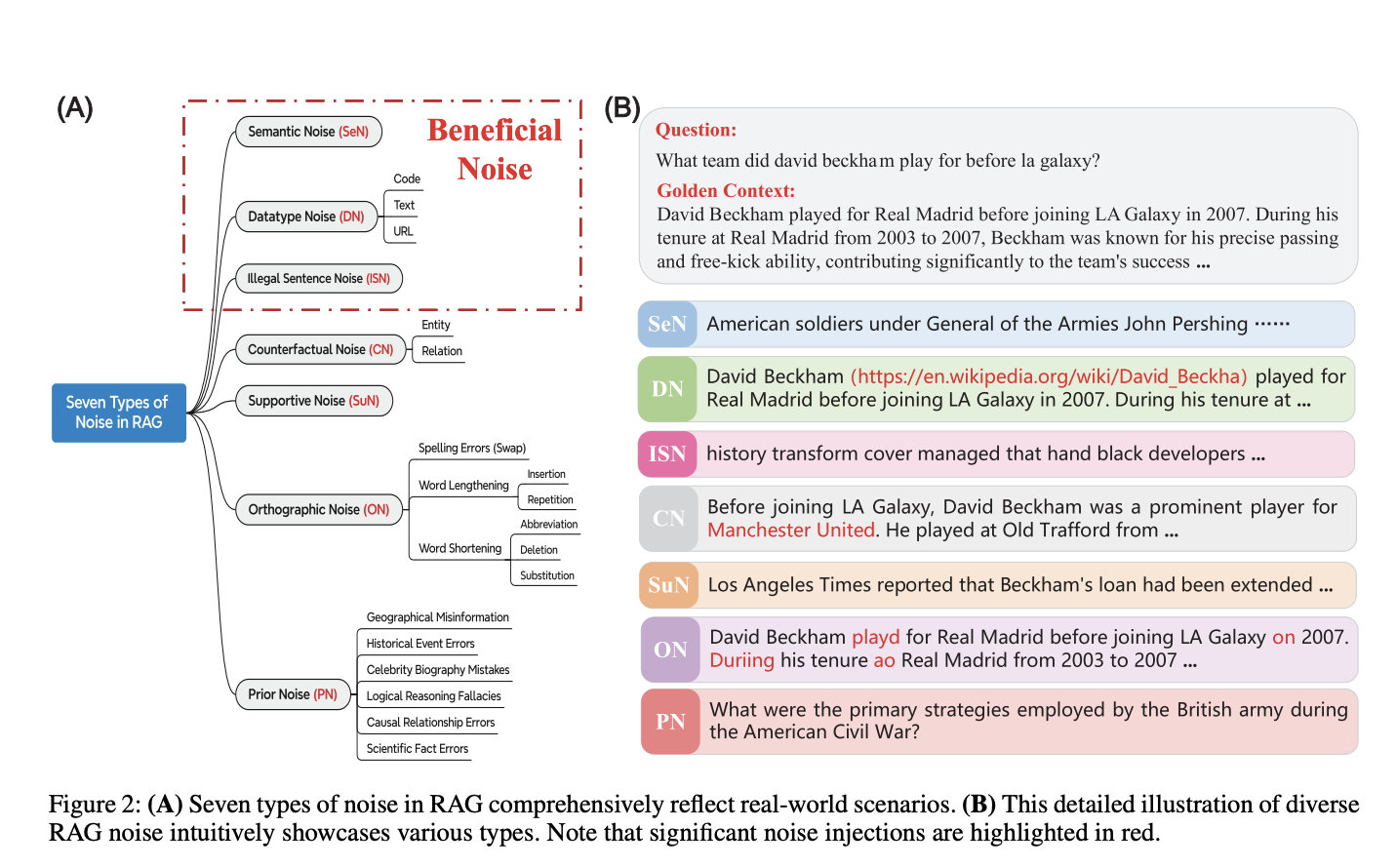
Exploring the Dual Nature of RAG Noise: Enhancing Large Language Models Through Beneficial Noise and Mitigating Harmful Effects
Value of the Research
Research on Retrieval-Augmented Generation (RAG) in large language models (LLMs) has identified practical solutions to improve model performance and mitigate noise effects. The study introduces a novel evaluation framework, NoiserBench, and categorizes noise into beneficial and harmful types, offering a structured approach to enhancing RAG systems and improving LLM performance across various scenarios.
Practical Solutions
The study defines seven distinct noise types and categorizes them as beneficial or harmful. It introduces a systematic framework, NoiserBench, to create diverse noisy documents for comprehensive evaluation of their influence on model outputs. The research highlights the significance of managing noise types to optimize LLM performance in RAG systems.
Impact on Large Language Models
The study shows that beneficial noise, such as illegal sentence noise (ISN), consistently improves model accuracy by up to 3.32%, enhancing reasoning and response confidence. On the other hand, harmful noise types, like counterfactual noise (CN) and orthographic noise (ON), degrade performance, disrupting fact discernment. The evaluation framework underscores the importance of managing noise types to optimize LLM performance in RAG systems.
Recommendations for AI Implementation
The research emphasizes the need to focus on leveraging beneficial noise while mitigating harmful effects, setting the foundation for more robust and adaptable RAG systems.
Engage with Us
If you are interested in evolving your company with AI and exploring automation opportunities, connect with us at hello@itinai.com. For continuous insights into leveraging AI, stay tuned on our Telegram t.me/itinainews or Twitter @itinaicom.