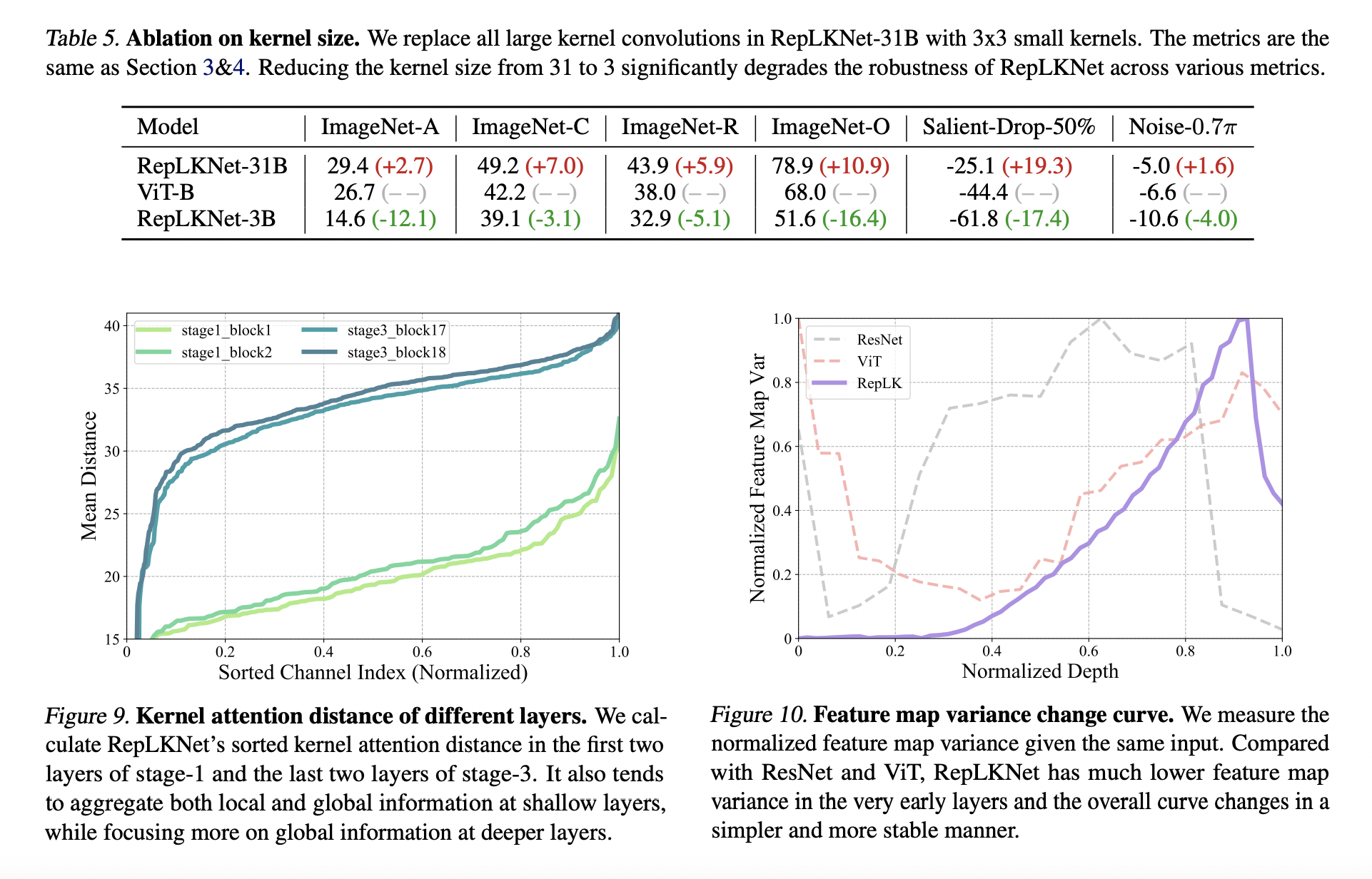
Robustness of Vision Transformers and Convolutional Neural Networks
Practical Solutions for Real-World Applications
The Study
Recent advancements in large kernel convolutions have shown potential to match or exceed the performance of Vision Transformers (ViTs). This study evaluates the robustness of large kernel convolutional networks (convents) compared to traditional CNNs and ViTs, highlighting their unique properties that contribute to robustness.
Research Findings
The study demonstrates that large kernel convents exhibit remarkable robustness, sometimes even outperforming ViTs. Through a series of experiments, unique properties such as occlusion invariance, kernel attention patterns, and frequency characteristics contribute to their robustness. The study challenges the belief that self-attention is necessary for robustness, suggesting that traditional CNNs can achieve comparable levels of robustness.
Implications
The research confirms the significant robustness of large kernel ConvNets across six standard benchmark datasets, shedding light on the factors underlying their resilience. This suggests promising avenues for the application and development of large kernel ConvNets in future research and practical use.
Application of AI Solutions
If you want to evolve your company with AI, stay competitive, and use AI for your advantage, exploring the robustness of large kernel ConvNets can redefine your way of work. Tasks include identifying automation opportunities, defining KPIs, selecting AI solutions, and implementing them gradually to leverage their impact on business outcomes. For AI KPI management advice and continuous insights into leveraging AI, stay connected with us at hello@itinai.com and on Telegram and Twitter.
Discover AI-driven Sales Processes and Customer Engagement
Explore solutions that redefine sales processes and customer engagement at itinai.com.