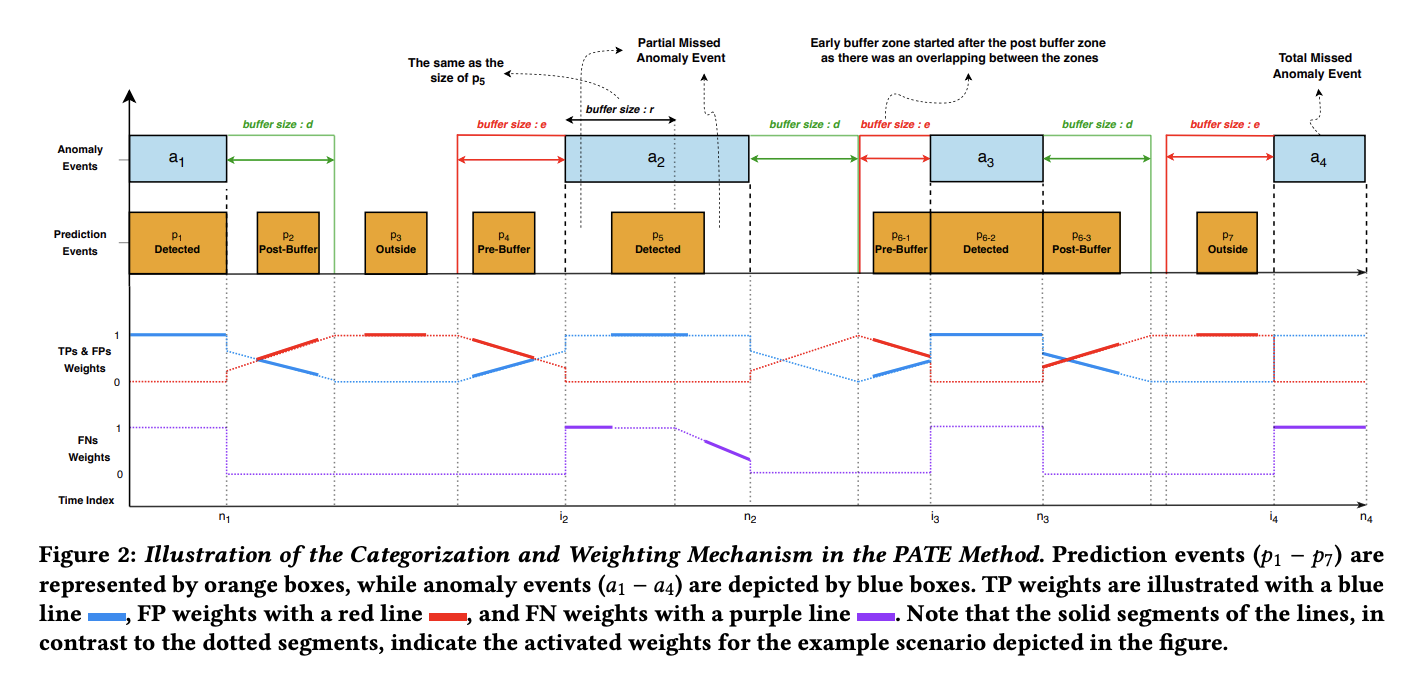
Anomaly Detection in Time Series Data
Time series anomaly detection is crucial for various applications, from monitoring industrial systems to detecting fraudulent activities. Conventional metrics like Precision and Recall may not accurately capture the intricacies of time series anomalies, leading to erroneous assessments in critical applications.
Introducing Proximity-Aware Time Series Anomaly Evaluation (PATE)
The PATE measure provides a more accurate evaluation of time series anomaly detection by incorporating proximity-based weighting and temporal correlations, addressing the shortcomings of existing metrics.
Practical Solutions and Value
The PATE measure offers a comprehensive tool for evaluating anomaly detection algorithms, considering crucial elements such as coverage level, onset response timing, and early and delayed detection. It enables a more thorough and precise evaluation of anomaly detection models, improving alignment with real-world applications where prompt and accurate detection is essential.
Implications and Application
The incorporation of temporal proximity and buffer zones in the PATE measure allows for a more accurate and nuanced assessment of anomaly detection models, with potential implications for guiding future research, influencing industry adoption, and enhancing practical applications in critical domains such as healthcare and finance.
AI Solutions for Business Evolution
Discover how AI can redefine your way of work and identify automation opportunities, define KPIs, select AI solutions, and implement gradually to stay competitive in the evolving business landscape.
Spotlight on a Practical AI Solution
Consider the AI Sales Bot from itinai.com/aisalesbot designed to automate customer engagement 24/7 and manage interactions across all customer journey stages, redefining sales processes and customer engagement.