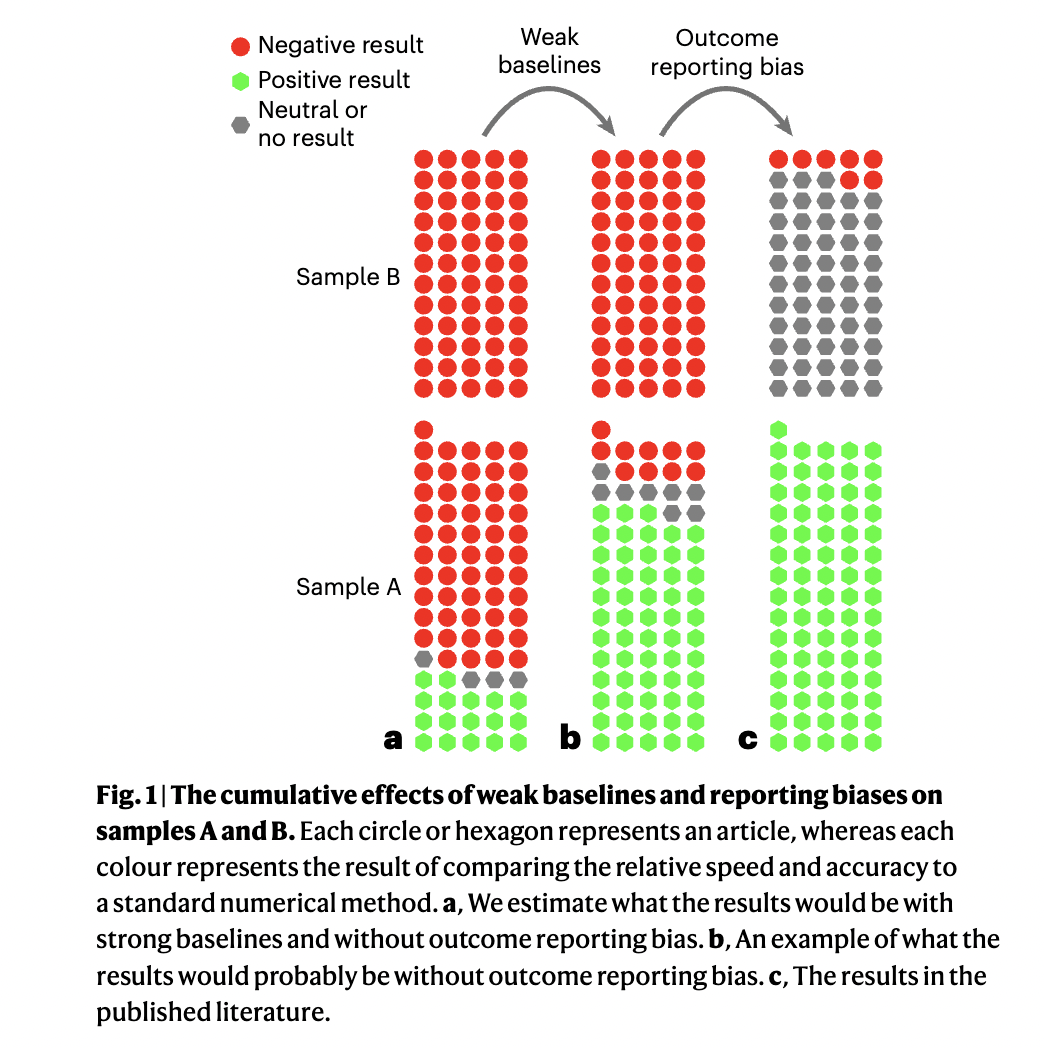
Practical Solutions and Value of Machine Learning in Solving Partial Differential Equations
Overview
Machine Learning (ML) accelerates solving partial differential equations (PDEs) in computational physics, aiming for faster and accurate solutions than traditional methods.
Challenges and Solutions
Concerns like data leakage and weak baselines hinder ML’s performance claims. Despite challenges, ML offers benefits for optimization and reducing computational time.
Research Findings
Studies reveal overoptimistic claims in ML literature for PDE solving, with weak baselines leading to exaggerated results. Reporting biases skew findings, impacting the evaluation of ML success.
Recommendations
Ensure fair comparisons by comparing ML solvers with efficient numerical methods. Justify hardware choices and enhance transparency in reporting to improve reliability in ML research for PDE applications.
AI Implementation
Evolve your company with AI by identifying automation opportunities, defining KPIs, selecting suitable AI solutions, and implementing gradually. Connect with us for AI KPI management advice and insights into leveraging AI.
For more information, visit the original research paper.