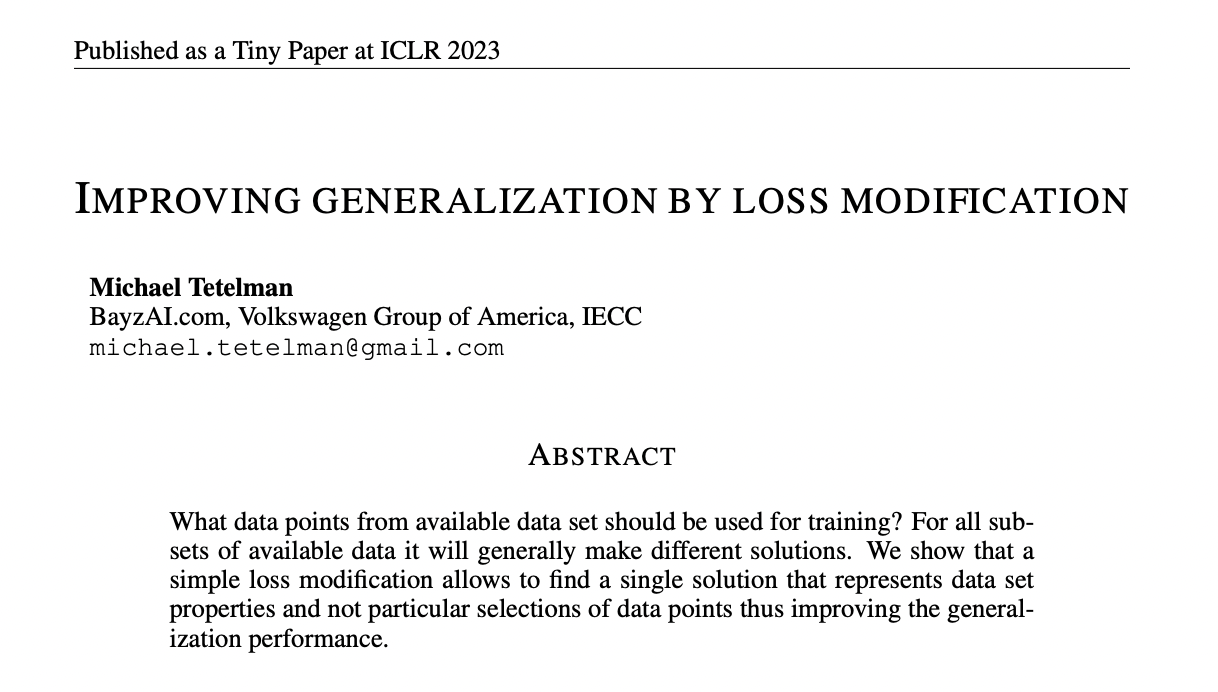
Enhancing Neural Network Generalization with Outlier Suppression Loss
A research study from BayzAI.com, Volkswagen Group of America, and IECC addresses the challenge of training neural networks to accurately represent the distributional properties of a dataset without being influenced by specific data points. This is crucial for achieving better generalization to unseen data.
The proposed method focuses on using heuristics like outlier suppression and robust loss functions to improve convergence and generalization of neural networks. By utilizing techniques such as the Huber loss and selecting low-loss samples in Stochastic Gradient Descent (SGD), the method aims to handle outliers and enhance robustness.
The key idea behind the method involves defining a weight distribution that averages probability distributions across all subsets of the dataset through Bayesian inference. This results in a method that mitigates the influence of outliers, thereby improving robustness and generalization.
The study demonstrates that the method significantly improves prediction accuracy and stabilizes learning, particularly evident in applications like GAN training, where stability is crucial for reaching Nash equilibrium.
AI Solutions for Your Company
Evolve your company with AI and stay competitive by leveraging the Enhancing Neural Network Generalization with Outlier Suppression Loss research. Identify automation opportunities, define KPIs, select an AI solution, and implement gradually to reap the benefits of AI in your business.
For AI KPI management advice and insights into leveraging AI, connect with us at hello@itinai.com. Stay updated on our Telegram t.me/itinainews and Twitter @itinaicom.
Discover how AI can redefine your sales processes and customer engagement. Explore solutions at itinai.com.