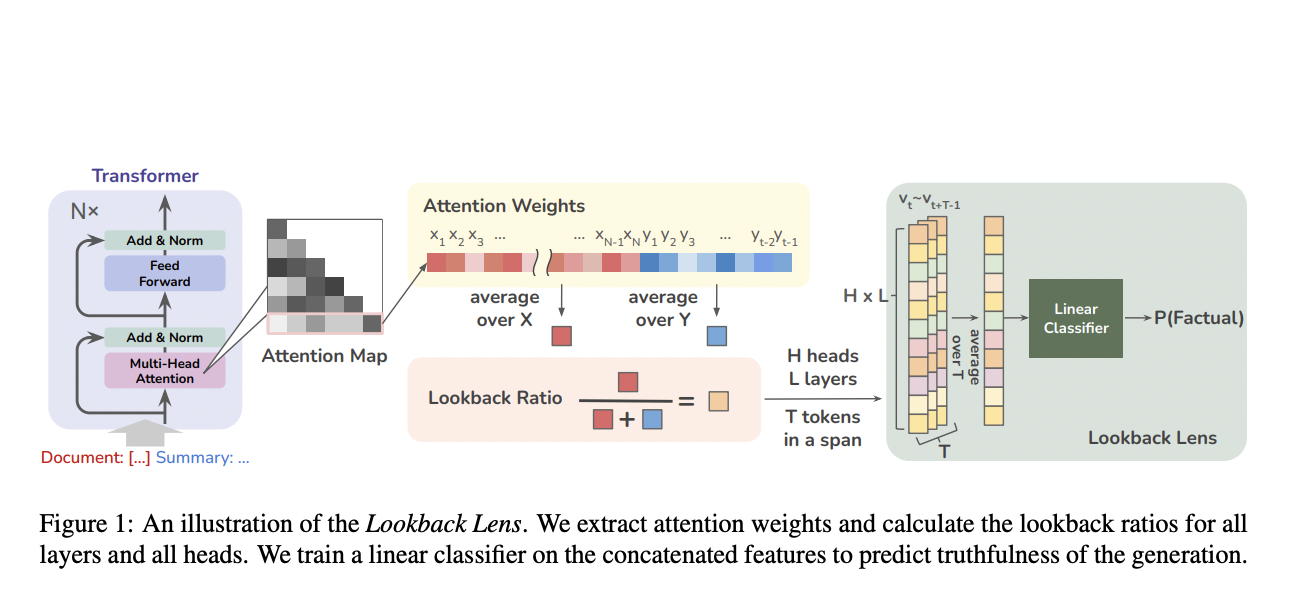
Enhancing LLM Reliability: The Lookback Lens Approach to Hallucination Detection
Practical Solutions and Value
Large Language Models (LLMs) like GPT-4 are powerful in text generation but can produce inaccurate or irrelevant content, termed “hallucinations.” These errors undermine the reliability of LLMs in critical applications.
Prior work focused on detecting and mitigating hallucinations, but existing methods do not specifically address contextual hallucinations, where the provided context is key.
The Lookback Lens, a novel approach, leverages attention maps of LLMs to detect and mitigate hallucinations. It introduces a simple yet effective hallucination detection model based on the ratio of attention weights on the context versus the newly generated tokens, termed the ‘lookback ratio.’
The Lookback Lens is validated through experiments on summarization and question-answering tasks, demonstrating its robustness and generalizability. It can be transferred across different models and tasks without retraining.
A classifier-guided decoding strategy further mitigates hallucinations during text generation, reducing hallucinations by 9.6% in the XSum summarization task.
This approach represents a promising step toward more accurate and reliable LLM-generated content.
AI Solutions for Your Company
Identify Automation Opportunities: Locate key customer interaction points that can benefit from AI.
Define KPIs: Ensure your AI endeavors have measurable impacts on business outcomes.
Select an AI Solution: Choose tools that align with your needs and provide customization.
Implement Gradually: Start with a pilot, gather data, and expand AI usage judiciously.
For AI KPI management advice, connect with us at hello@itinai.com. For continuous insights into leveraging AI, stay tuned on our Telegram or Twitter.
Discover how AI can redefine your sales processes and customer engagement. Explore solutions at itinai.com.